On the limits of agency in agent-based models
Ayush Chopra, Shashank Kumar, Nurullah Giray-Kuru, Ramesh Raskar, Arnau Quera-Bofarull
2024-09-18
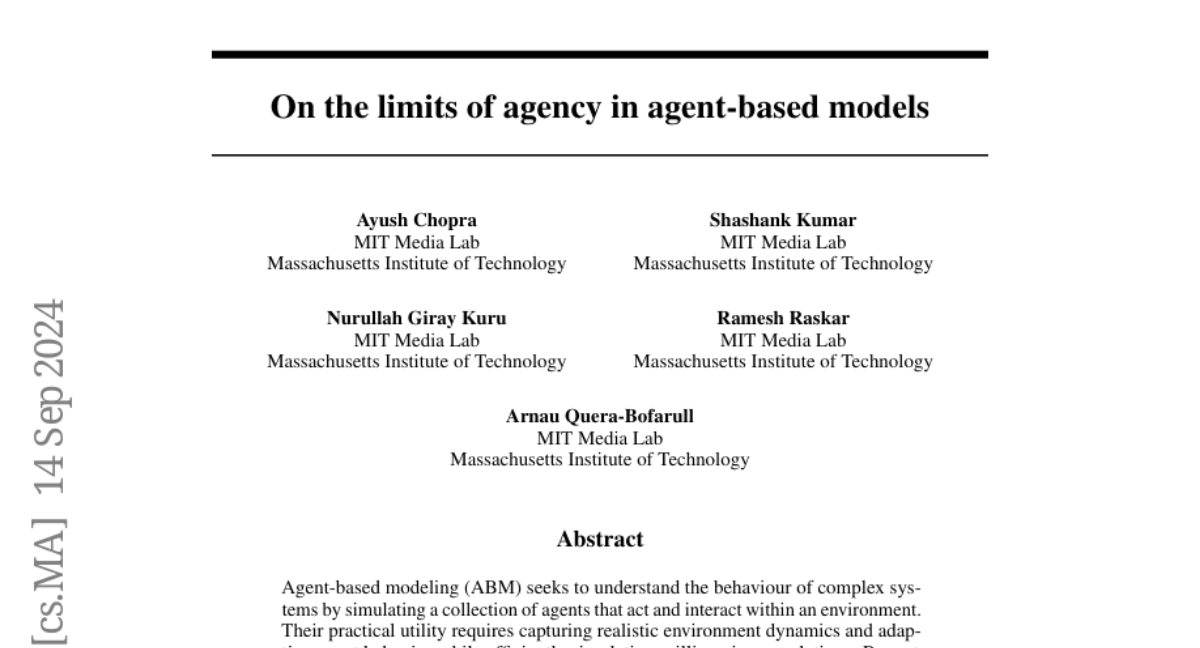
Summary
This paper discusses AgentTorch, a new framework designed to improve agent-based modeling (ABM) by allowing simulations of millions of agents that can interact and adapt in complex environments, such as during the COVID-19 pandemic.
What's the problem?
Agent-based models aim to simulate how individual agents (like people or cells) behave and interact within a system. However, existing models struggle to efficiently simulate large populations and accurately capture realistic behaviors. Additionally, using advanced AI models like large language models (LLMs) for these simulations is computationally expensive, making it hard to apply them on a large scale.
What's the solution?
AgentTorch addresses these challenges by creating a scalable framework that can handle millions of agents while using LLMs to capture adaptive behaviors. The framework allows researchers to simulate scenarios like the COVID-19 pandemic, examining how factors like isolation and employment affect health and economy. It uses a combination of different agent designs and benchmarks their performance to find the best methods for predicting outcomes like disease spread and unemployment rates. The framework is open-source, making it accessible for research and policy-making.
Why it matters?
This research is important because it enhances our ability to model complex systems, which can help in understanding real-world issues like pandemics or climate change. By improving how we simulate agent interactions, AgentTorch can provide valuable insights for better decision-making in public health, economic policies, and other critical areas.
Abstract
Agent-based modeling (ABM) seeks to understand the behavior of complex systems by simulating a collection of agents that act and interact within an environment. Their practical utility requires capturing realistic environment dynamics and adaptive agent behavior while efficiently simulating million-size populations. Recent advancements in large language models (LLMs) present an opportunity to enhance ABMs by using LLMs as agents with further potential to capture adaptive behavior. However, the computational infeasibility of using LLMs for large populations has hindered their widespread adoption. In this paper, we introduce AgentTorch -- a framework that scales ABMs to millions of agents while capturing high-resolution agent behavior using LLMs. We benchmark the utility of LLMs as ABM agents, exploring the trade-off between simulation scale and individual agency. Using the COVID-19 pandemic as a case study, we demonstrate how AgentTorch can simulate 8.4 million agents representing New York City, capturing the impact of isolation and employment behavior on health and economic outcomes. We compare the performance of different agent architectures based on heuristic and LLM agents in predicting disease waves and unemployment rates. Furthermore, we showcase AgentTorch's capabilities for retrospective, counterfactual, and prospective analyses, highlighting how adaptive agent behavior can help overcome the limitations of historical data in policy design. AgentTorch is an open-source project actively being used for policy-making and scientific discovery around the world. The framework is available here: github.com/AgentTorch/AgentTorch.