One missing piece in Vision and Language: A Survey on Comics Understanding
Emanuele Vivoli, Andrey Barsky, Mohamed Ali Souibgui, Artemis LLabres, Marco Bertini, Dimosthenis Karatzas
2024-09-17
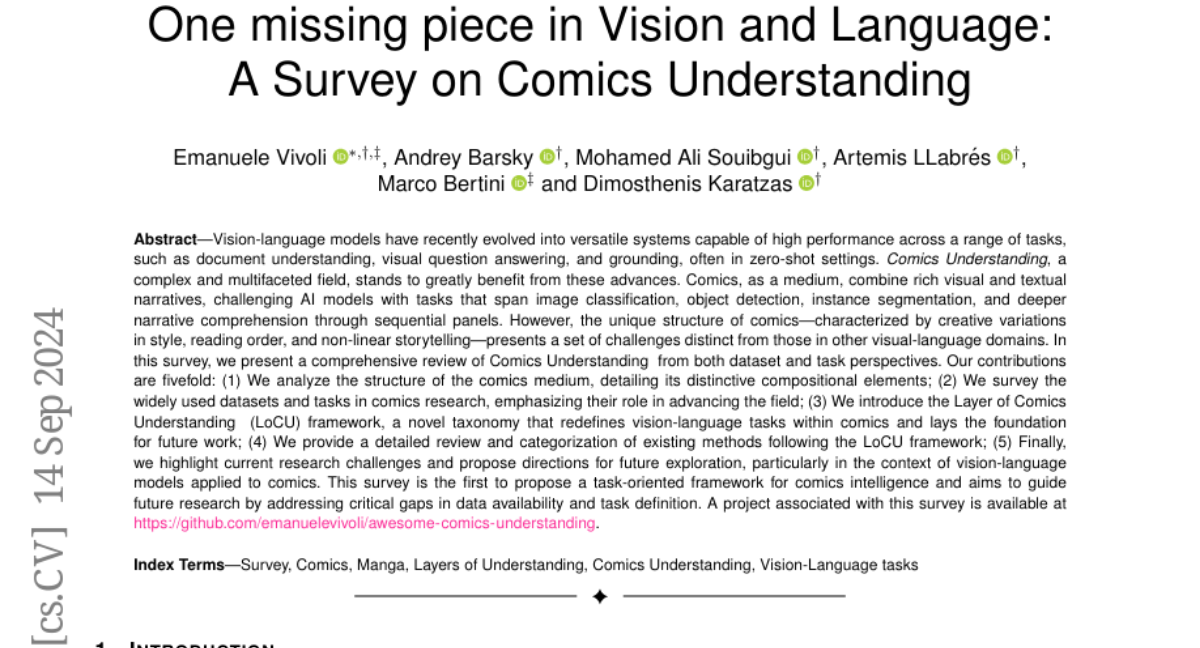
Summary
This paper surveys the field of Comics Understanding, exploring how vision-language models can be applied to analyze and interpret comics, which combine images and text in unique ways.
What's the problem?
Comics are a complex medium that mixes visuals and narratives, making it difficult for AI models to effectively understand them. Existing models excel in tasks like image classification or text comprehension but struggle with the specific challenges posed by comics, such as varying styles, reading orders, and non-linear storytelling.
What's the solution?
The authors provide a comprehensive review of Comics Understanding from two perspectives: datasets and tasks. They introduce a new framework called the Layer of Comics Understanding (LoCU) to categorize different tasks related to comic analysis. This framework helps clarify the unique aspects of comics and guides future research by identifying gaps in current methods and data availability.
Why it matters?
This research is significant because it lays the groundwork for improving how AI can interpret comics, which could enhance applications in education, entertainment, and digital media. By developing a structured approach to understanding comics, it opens up new possibilities for using AI in creative fields.
Abstract
Vision-language models have recently evolved into versatile systems capable of high performance across a range of tasks, such as document understanding, visual question answering, and grounding, often in zero-shot settings. Comics Understanding, a complex and multifaceted field, stands to greatly benefit from these advances. Comics, as a medium, combine rich visual and textual narratives, challenging AI models with tasks that span image classification, object detection, instance segmentation, and deeper narrative comprehension through sequential panels. However, the unique structure of comics -- characterized by creative variations in style, reading order, and non-linear storytelling -- presents a set of challenges distinct from those in other visual-language domains. In this survey, we present a comprehensive review of Comics Understanding from both dataset and task perspectives. Our contributions are fivefold: (1) We analyze the structure of the comics medium, detailing its distinctive compositional elements; (2) We survey the widely used datasets and tasks in comics research, emphasizing their role in advancing the field; (3) We introduce the Layer of Comics Understanding (LoCU) framework, a novel taxonomy that redefines vision-language tasks within comics and lays the foundation for future work; (4) We provide a detailed review and categorization of existing methods following the LoCU framework; (5) Finally, we highlight current research challenges and propose directions for future exploration, particularly in the context of vision-language models applied to comics. This survey is the first to propose a task-oriented framework for comics intelligence and aims to guide future research by addressing critical gaps in data availability and task definition. A project associated with this survey is available at https://github.com/emanuelevivoli/awesome-comics-understanding.