OneGen: Efficient One-Pass Unified Generation and Retrieval for LLMs
Jintian Zhang, Cheng Peng, Mengshu Sun, Xiang Chen, Lei Liang, Zhiqiang Zhang, Jun Zhou, Huajun Chen, Ningyu Zhang
2024-09-10
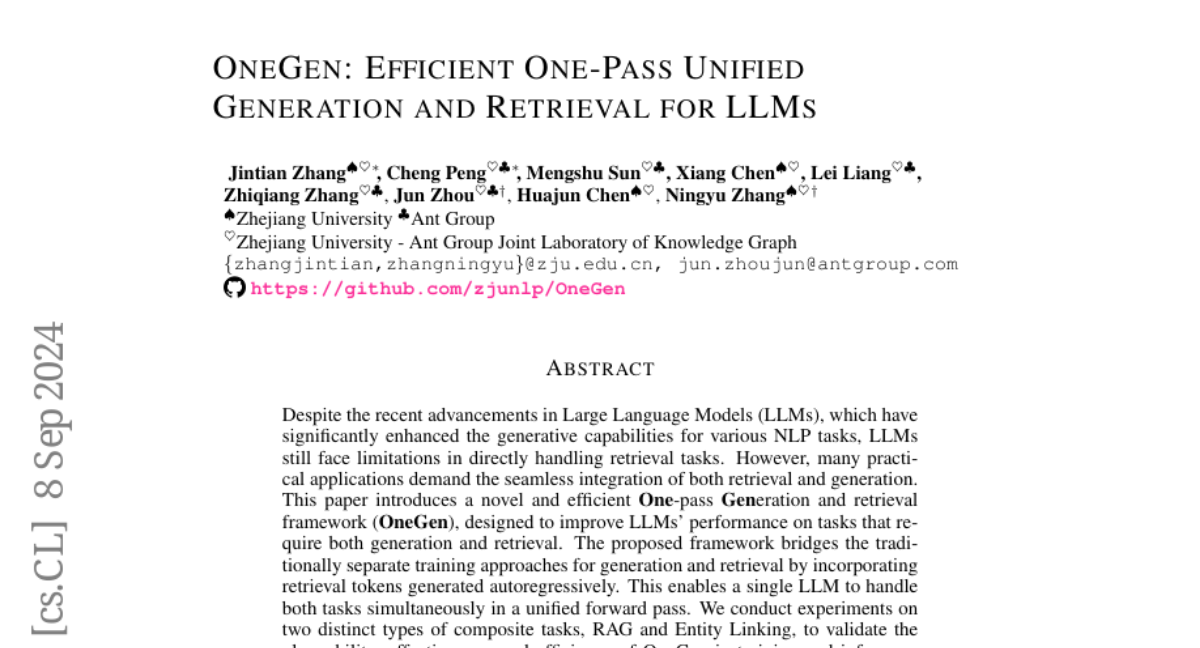
Summary
This paper talks about OneGen, a new framework that allows large language models (LLMs) to efficiently generate and retrieve information in a single step.
What's the problem?
Even though LLMs have improved a lot in generating text, they still struggle with tasks that require both generating new content and retrieving specific information. Many applications need these two functions to work together seamlessly, but current systems treat them separately, which can slow down performance and make it less effective.
What's the solution?
The authors introduce OneGen, which combines generation and retrieval into one process. This framework uses special tokens that help the model perform both tasks at the same time without needing to switch between different methods. They tested OneGen on two types of tasks—RAG (Retrieval-Augmented Generation) and Entity Linking—to show how well it works. Their results indicate that this unified approach not only maintains the model's ability to generate text but also improves its retrieval performance.
Why it matters?
This research is important because it enhances how LLMs can be used in real-world applications where both generating and retrieving information are needed. By making these processes more efficient, OneGen can help improve tools for search engines, chatbots, and other AI applications that rely on understanding and generating text.
Abstract
Despite the recent advancements in Large Language Models (LLMs), which have significantly enhanced the generative capabilities for various NLP tasks, LLMs still face limitations in directly handling retrieval tasks. However, many practical applications demand the seamless integration of both retrieval and generation. This paper introduces a novel and efficient One-pass Generation and retrieval framework (OneGen), designed to improve LLMs' performance on tasks that require both generation and retrieval. The proposed framework bridges the traditionally separate training approaches for generation and retrieval by incorporating retrieval tokens generated autoregressively. This enables a single LLM to handle both tasks simultaneously in a unified forward pass. We conduct experiments on two distinct types of composite tasks, RAG and Entity Linking, to validate the pluggability, effectiveness, and efficiency of OneGen in training and inference. Furthermore, our results show that integrating generation and retrieval within the same context preserves the generative capabilities of LLMs while improving retrieval performance. To the best of our knowledge, OneGen is the first to enable LLMs to conduct vector retrieval during the generation.