Open Language Data Initiative: Advancing Low-Resource Machine Translation for Karakalpak
Mukhammadsaid Mamasaidov, Abror Shopulatov
2024-09-10
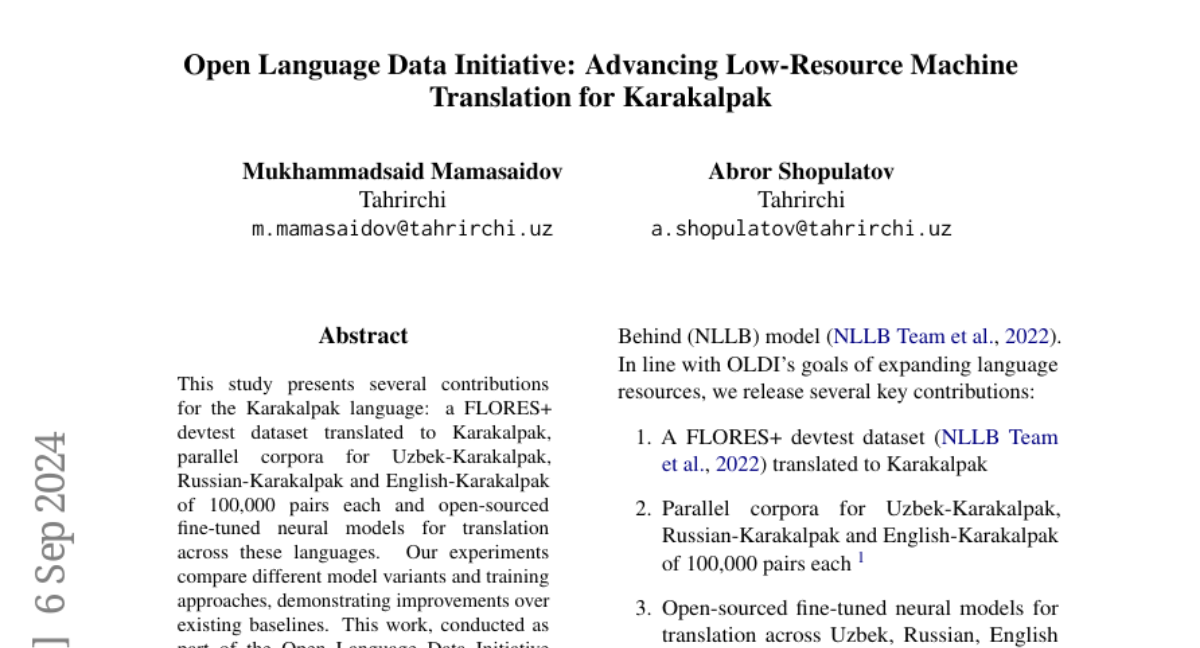
Summary
This paper talks about the Open Language Data Initiative, which aims to improve machine translation for the Karakalpak language by providing new datasets and translation models.
What's the problem?
The Karakalpak language, like many low-resource languages, lacks sufficient high-quality translation tools and datasets. This makes it difficult for speakers to access information in their language and limits the development of technology that can support it.
What's the solution?
To address this issue, the authors created several resources: a new dataset for evaluating translations called FLORES+ translated into Karakalpak, and parallel corpora (sets of translated texts) for Uzbek-Karakalpak, Russian-Karakalpak, and English-Karakalpak, each containing 100,000 translation pairs. They also developed fine-tuned neural models that can translate between these languages effectively. Their experiments showed that these new models perform better than existing ones.
Why it matters?
This research is important because it helps expand the availability of machine translation for the Karakalpak language. By providing better tools and resources, it supports linguistic diversity in technology and helps speakers of low-resource languages access information more easily.
Abstract
This study presents several contributions for the Karakalpak language: a FLORES+ devtest dataset translated to Karakalpak, parallel corpora for Uzbek-Karakalpak, Russian-Karakalpak and English-Karakalpak of 100,000 pairs each and open-sourced fine-tuned neural models for translation across these languages. Our experiments compare different model variants and training approaches, demonstrating improvements over existing baselines. This work, conducted as part of the Open Language Data Initiative (OLDI) shared task, aims to advance machine translation capabilities for Karakalpak and contribute to expanding linguistic diversity in NLP technologies.