Open Materials 2024 (OMat24) Inorganic Materials Dataset and Models
Luis Barroso-Luque, Muhammed Shuaibi, Xiang Fu, Brandon M. Wood, Misko Dzamba, Meng Gao, Ammar Rizvi, C. Lawrence Zitnick, Zachary W. Ulissi
2024-10-18
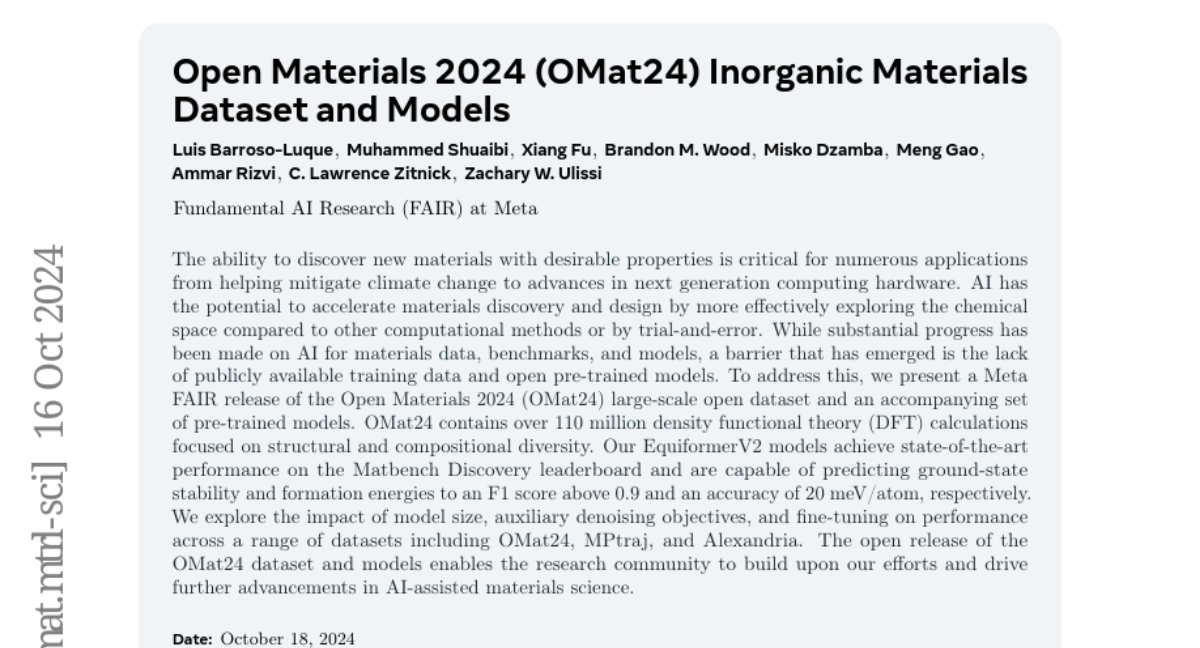
Summary
This paper introduces Open Materials 2024 (OMat24), a large dataset and models designed to help researchers discover new materials more efficiently using artificial intelligence (AI).
What's the problem?
Finding new materials with useful properties is important for many applications, like improving batteries or creating sustainable fuels. However, traditional methods of discovering materials can be slow and expensive because they often rely on trial-and-error or require a lot of computing power. Additionally, there is a lack of publicly available data and models that researchers can use to accelerate this process.
What's the solution?
To address these challenges, the authors created OMat24, which includes over 110 million calculations based on density functional theory (DFT). This dataset provides a wide range of information about different inorganic materials. Along with the dataset, they developed pre-trained models called EquiformerV2 that can predict important material properties effectively. By making this data and these models publicly available, researchers can build upon their work and advance the field of materials science more rapidly.
Why it matters?
This research is significant because it opens up access to valuable resources that can speed up the discovery of new materials. By providing a large, high-quality dataset and powerful models for free, OMat24 encourages collaboration and innovation in materials science. This could lead to breakthroughs in technology that help tackle global challenges like climate change and improve computing hardware.
Abstract
The ability to discover new materials with desirable properties is critical for numerous applications from helping mitigate climate change to advances in next generation computing hardware. AI has the potential to accelerate materials discovery and design by more effectively exploring the chemical space compared to other computational methods or by trial-and-error. While substantial progress has been made on AI for materials data, benchmarks, and models, a barrier that has emerged is the lack of publicly available training data and open pre-trained models. To address this, we present a Meta FAIR release of the Open Materials 2024 (OMat24) large-scale open dataset and an accompanying set of pre-trained models. OMat24 contains over 110 million density functional theory (DFT) calculations focused on structural and compositional diversity. Our EquiformerV2 models achieve state-of-the-art performance on the Matbench Discovery leaderboard and are capable of predicting ground-state stability and formation energies to an F1 score above 0.9 and an accuracy of 20 meV/atom, respectively. We explore the impact of model size, auxiliary denoising objectives, and fine-tuning on performance across a range of datasets including OMat24, MPtraj, and Alexandria. The open release of the OMat24 dataset and models enables the research community to build upon our efforts and drive further advancements in AI-assisted materials science.