Open-RAG: Enhanced Retrieval-Augmented Reasoning with Open-Source Large Language Models
Shayekh Bin Islam, Md Asib Rahman, K S M Tozammel Hossain, Enamul Hoque, Shafiq Joty, Md Rizwan Parvez
2024-10-04
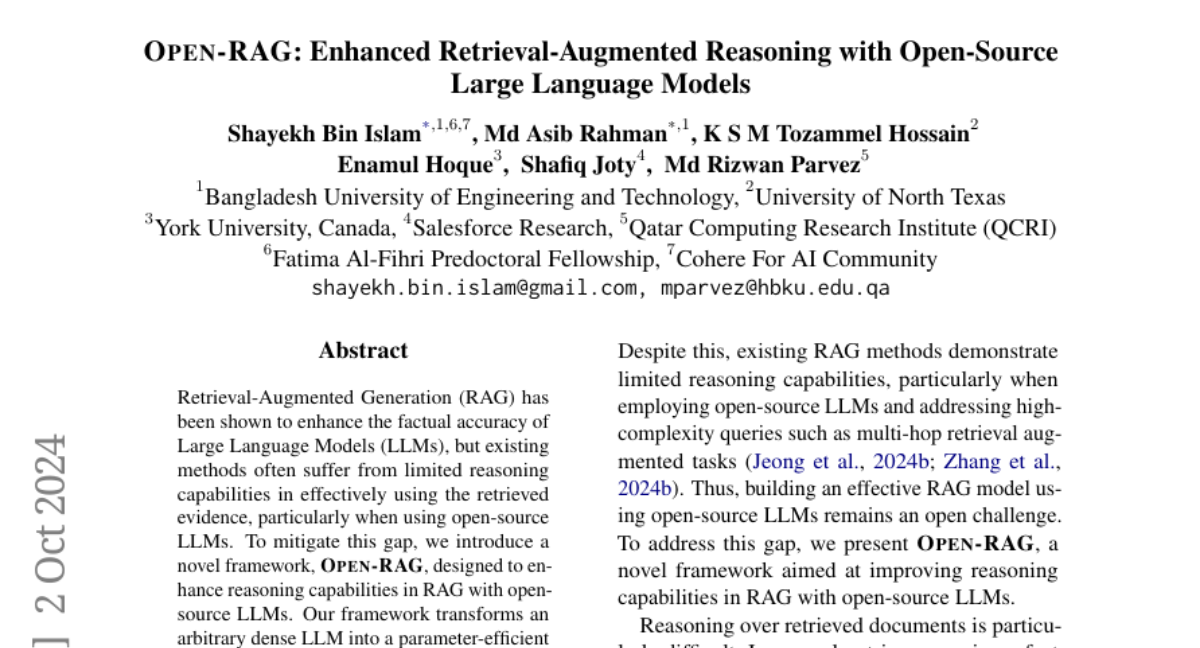
Summary
This paper presents Open-RAG, a new framework designed to improve how open-source large language models (LLMs) reason and retrieve information, making them more effective for complex tasks.
What's the problem?
While Retrieval-Augmented Generation (RAG) methods help LLMs provide accurate information by retrieving relevant data, they often struggle with reasoning effectively, especially when using open-source models. This can lead to difficulties in understanding and using the retrieved evidence correctly, which limits their performance on complex questions that require deeper reasoning.
What's the solution?
To address this issue, the authors developed Open-RAG, which transforms standard LLMs into a more efficient model called a sparse mixture of experts (MoE). This new approach allows the model to better handle complex reasoning tasks by dynamically selecting the most relevant 'experts' or parts of the model to use for each specific task. Open-RAG also trains the model to recognize and navigate misleading information that may seem relevant but is actually incorrect. Additionally, it includes a hybrid method for deciding when to retrieve information, balancing speed and performance.
Why it matters?
This research is important because it enhances the capabilities of LLMs in reasoning and retrieving information, allowing them to provide more accurate and contextually relevant responses. By improving how these models work, Open-RAG can significantly benefit applications that require detailed knowledge and reasoning, such as question answering systems, educational tools, and more advanced AI applications.
Abstract
Retrieval-Augmented Generation (RAG) has been shown to enhance the factual accuracy of Large Language Models (LLMs), but existing methods often suffer from limited reasoning capabilities in effectively using the retrieved evidence, particularly when using open-source LLMs. To mitigate this gap, we introduce a novel framework, Open-RAG, designed to enhance reasoning capabilities in RAG with open-source LLMs. Our framework transforms an arbitrary dense LLM into a parameter-efficient sparse mixture of experts (MoE) model capable of handling complex reasoning tasks, including both single- and multi-hop queries. Open-RAG uniquely trains the model to navigate challenging distractors that appear relevant but are misleading. As a result, Open-RAG leverages latent learning, dynamically selecting relevant experts and integrating external knowledge effectively for more accurate and contextually relevant responses. In addition, we propose a hybrid adaptive retrieval method to determine retrieval necessity and balance the trade-off between performance gain and inference speed. Experimental results show that the Llama2-7B-based Open-RAG outperforms state-of-the-art LLMs and RAG models such as ChatGPT, Self-RAG, and Command R+ in various knowledge-intensive tasks. We open-source our code and models at https://openragmoe.github.io/