Open-Vocabulary Audio-Visual Semantic Segmentation
Ruohao Guo, Liao Qu, Dantong Niu, Yanyu Qi, Wenzhen Yue, Ji Shi, Bowei Xing, Xianghua Ying
2024-08-01
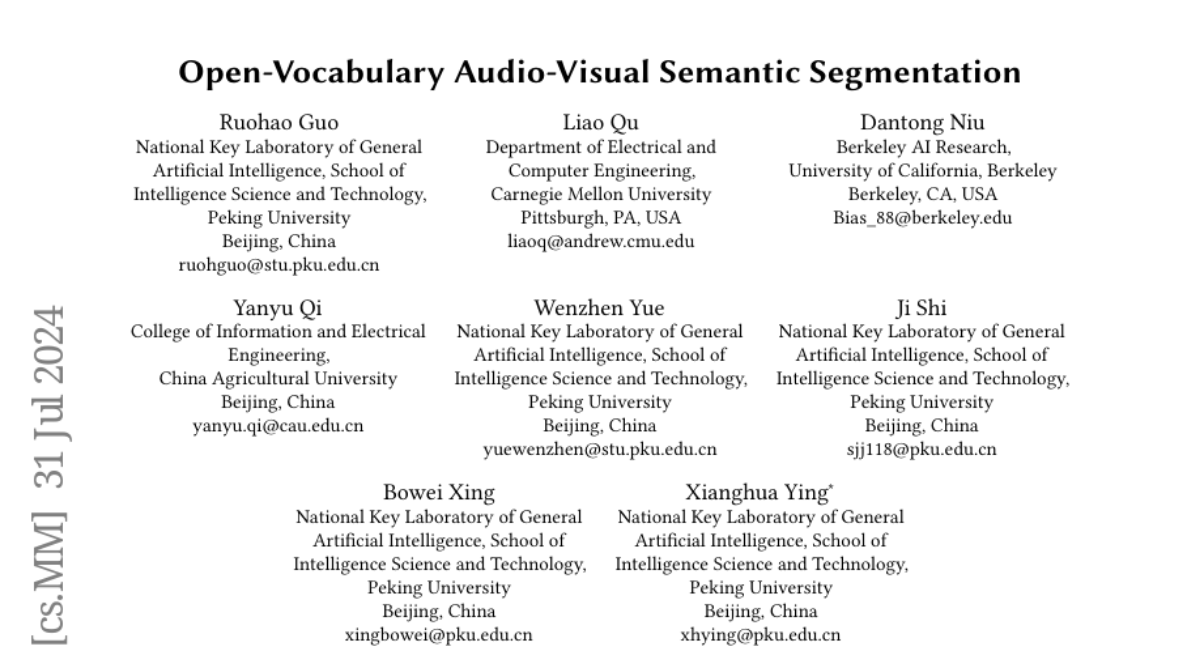
Summary
This paper introduces a new approach called open-vocabulary audio-visual semantic segmentation (AVSS), which aims to identify and classify objects that make sounds in videos, even if those objects were not part of the training data.
What's the problem?
Most current methods for audio-visual semantic segmentation can only recognize specific categories that they were trained on. This means they struggle to identify new or unexpected objects in videos, which limits their usefulness in real-world situations where they might encounter unfamiliar sounds or visuals.
What's the solution?
To overcome this limitation, the authors developed a framework called OV-AVSS. This framework includes two main parts: a module that locates sounds in videos and combines audio and visual information, and a classification module that predicts what those sounds are using knowledge from large pre-trained models. This allows the system to recognize a wider range of objects, including those it has never seen before during training.
Why it matters?
This research is important because it enhances the ability of AI systems to understand complex video content in a more flexible way. By enabling models to recognize new categories of objects based on sound, OV-AVSS can improve applications like video indexing, content retrieval, and accessibility services for people with hearing impairments. This advancement could lead to smarter AI systems that better understand the world around them.
Abstract
Audio-visual semantic segmentation (AVSS) aims to segment and classify sounding objects in videos with acoustic cues. However, most approaches operate on the close-set assumption and only identify pre-defined categories from training data, lacking the generalization ability to detect novel categories in practical applications. In this paper, we introduce a new task: open-vocabulary audio-visual semantic segmentation, extending AVSS task to open-world scenarios beyond the annotated label space. This is a more challenging task that requires recognizing all categories, even those that have never been seen nor heard during training. Moreover, we propose the first open-vocabulary AVSS framework, OV-AVSS, which mainly consists of two parts: 1) a universal sound source localization module to perform audio-visual fusion and locate all potential sounding objects and 2) an open-vocabulary classification module to predict categories with the help of the prior knowledge from large-scale pre-trained vision-language models. To properly evaluate the open-vocabulary AVSS, we split zero-shot training and testing subsets based on the AVSBench-semantic benchmark, namely AVSBench-OV. Extensive experiments demonstrate the strong segmentation and zero-shot generalization ability of our model on all categories. On the AVSBench-OV dataset, OV-AVSS achieves 55.43% mIoU on base categories and 29.14% mIoU on novel categories, exceeding the state-of-the-art zero-shot method by 41.88%/20.61% and open-vocabulary method by 10.2%/11.6%. The code is available at https://github.com/ruohaoguo/ovavss.