OpenAI o1 System Card
OpenAI, Aaron Jaech, Adam Kalai, Adam Lerer, Adam Richardson, Ahmed El-Kishky, Aiden Low, Alec Helyar, Aleksander Madry, Alex Beutel, Alex Carney, Alex Iftimie, Alex Karpenko, Alex Tachard Passos, Alexander Neitz, Alexander Prokofiev, Alexander Wei, Allison Tam, Ally Bennett, Ananya Kumar, Andre Saraiva, Andrea Vallone
2024-12-24
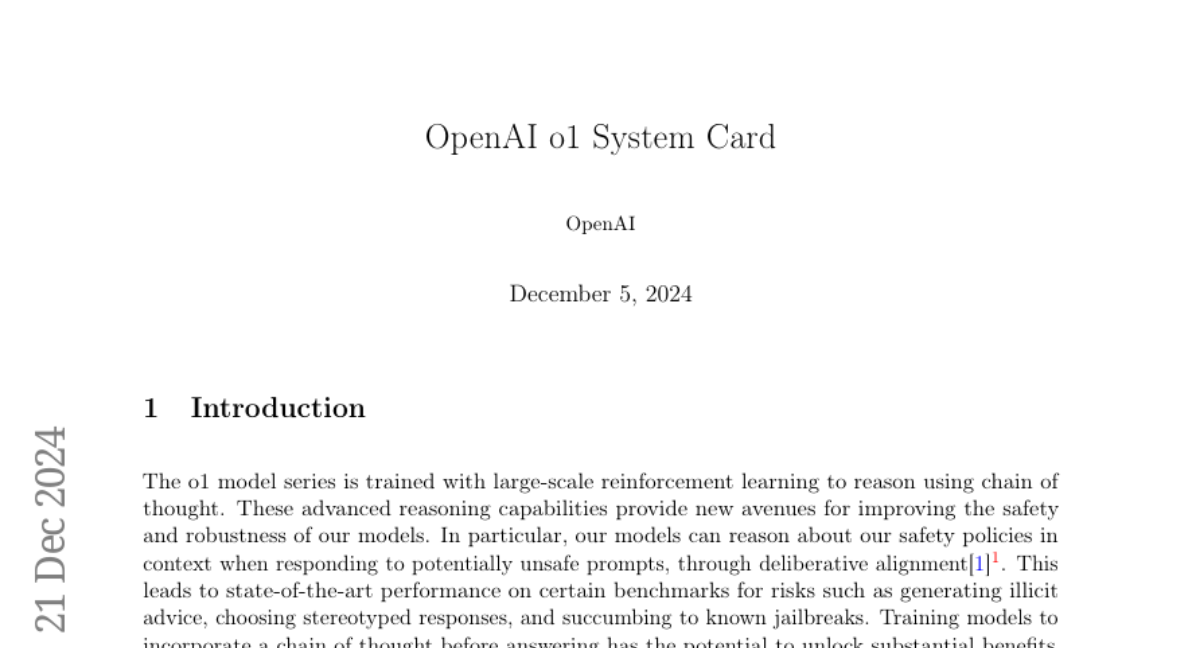
Summary
This paper talks about the OpenAI o1 model, a new large language model designed to improve reasoning abilities by using a method called chain-of-thought processing, which allows it to think through problems step-by-step before responding.
What's the problem?
Many existing language models struggle with complex reasoning tasks, such as math problems or coding challenges. They often provide quick answers without fully understanding the question, which can lead to errors or less accurate responses. Additionally, these models may generate inappropriate or unsafe content due to a lack of careful reasoning.
What's the solution?
The o1 model addresses these issues by incorporating advanced reasoning capabilities through reinforcement learning. This means it learns to think carefully and logically about problems before answering, similar to how a human would approach a difficult question. The model has been trained to evaluate its own responses and reduce mistakes, leading to better performance on complex tasks. It also includes measures to improve safety and reduce harmful outputs when responding to sensitive prompts.
Why it matters?
This research is important because it represents a significant step forward in developing AI that can reason more like humans. By enhancing the ability of models like o1 to tackle complex problems accurately and safely, OpenAI is paving the way for better applications in fields such as education, programming, and scientific research, where precise reasoning is crucial.
Abstract
The o1 model series is trained with large-scale reinforcement learning to reason using chain of thought. These advanced reasoning capabilities provide new avenues for improving the safety and robustness of our models. In particular, our models can reason about our safety policies in context when responding to potentially unsafe prompts, through deliberative alignment. This leads to state-of-the-art performance on certain benchmarks for risks such as generating illicit advice, choosing stereotyped responses, and succumbing to known jailbreaks. Training models to incorporate a chain of thought before answering has the potential to unlock substantial benefits, while also increasing potential risks that stem from heightened intelligence. Our results underscore the need for building robust alignment methods, extensively stress-testing their efficacy, and maintaining meticulous risk management protocols. This report outlines the safety work carried out for the OpenAI o1 and OpenAI o1-mini models, including safety evaluations, external red teaming, and Preparedness Framework evaluations.