Openstory++: A Large-scale Dataset and Benchmark for Instance-aware Open-domain Visual Storytelling
Zilyu Ye, Jinxiu Liu, Ruotian Peng, Jinjin Cao, Zhiyang Chen, Yiyang Zhang, Ziwei Xuan, Mingyuan Zhou, Xiaoqian Shen, Mohamed Elhoseiny, Qi Liu, Guo-Jun Qi
2024-08-08
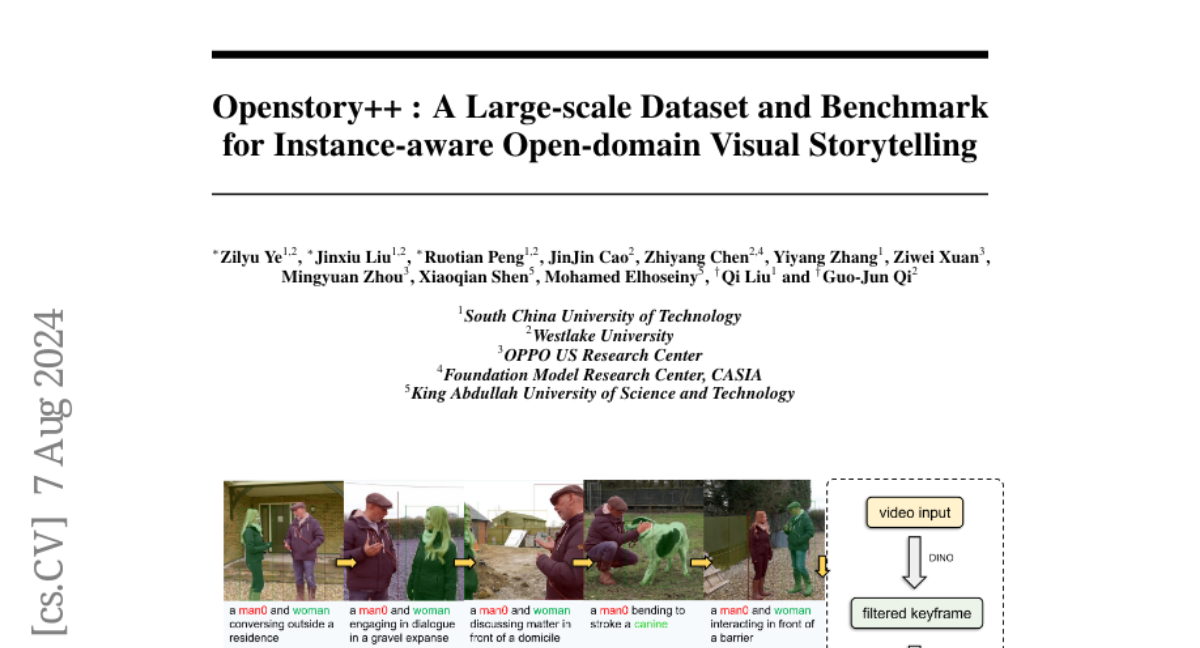
Summary
This paper introduces Openstory++, a large-scale dataset and evaluation framework designed to improve visual storytelling by providing detailed annotations for images and text.
What's the problem?
While recent image generation models can create high-quality images from short captions, they often struggle to maintain consistency across multiple images when telling longer stories. This inconsistency happens because existing datasets lack detailed labels that describe specific features of different instances in the images, making it hard for models to generate coherent narratives.
What's the solution?
To address these issues, the authors created Openstory++, which includes extensive instance-level annotations for both images and text. This dataset allows models to learn how to combine visual and textual information more effectively. They also developed a method for extracting keyframes from videos and generating captions that ensure the storytelling remains consistent. Additionally, they introduced a new benchmark called Cohere-Bench to evaluate how well models perform in generating coherent stories over longer contexts.
Why it matters?
This research is significant because it provides a valuable resource for training AI models that can create detailed and consistent visual stories. By improving how models understand and generate narratives, Openstory++ can enhance applications in creative writing, video summarization, and other fields where storytelling is important.
Abstract
Recent image generation models excel at creating high-quality images from brief captions. However, they fail to maintain consistency of multiple instances across images when encountering lengthy contexts. This inconsistency is largely due to in existing training datasets the absence of granular instance feature labeling in existing training datasets. To tackle these issues, we introduce Openstory++, a large-scale dataset combining additional instance-level annotations with both images and text. Furthermore, we develop a training methodology that emphasizes entity-centric image-text generation, ensuring that the models learn to effectively interweave visual and textual information. Specifically, Openstory++ streamlines the process of keyframe extraction from open-domain videos, employing vision-language models to generate captions that are then polished by a large language model for narrative continuity. It surpasses previous datasets by offering a more expansive open-domain resource, which incorporates automated captioning, high-resolution imagery tailored for instance count, and extensive frame sequences for temporal consistency. Additionally, we present Cohere-Bench, a pioneering benchmark framework for evaluating the image generation tasks when long multimodal context is provided, including the ability to keep the background, style, instances in the given context coherent. Compared to existing benchmarks, our work fills critical gaps in multi-modal generation, propelling the development of models that can adeptly generate and interpret complex narratives in open-domain environments. Experiments conducted within Cohere-Bench confirm the superiority of Openstory++ in nurturing high-quality visual storytelling models, enhancing their ability to address open-domain generation tasks. More details can be found at https://openstorypp.github.io/