OpenVid-1M: A Large-Scale High-Quality Dataset for Text-to-video Generation
Kepan Nan, Rui Xie, Penghao Zhou, Tiehan Fan, Zhenheng Yang, Zhijie Chen, Xiang Li, Jian Yang, Ying Tai
2024-07-03
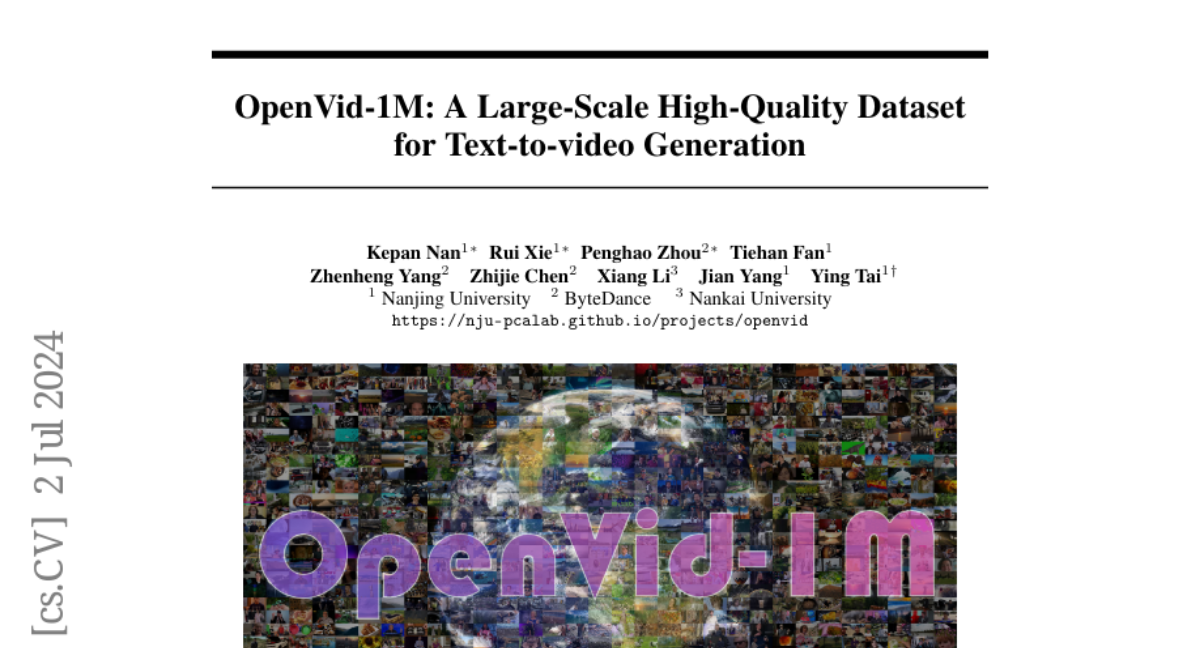
Summary
This paper talks about OpenVid-1M, a new dataset designed to improve text-to-video (T2V) generation by providing high-quality text-video pairs that researchers can use to create better videos from text descriptions.
What's the problem?
The main problem is that existing datasets for T2V generation are either low quality or too large for most researchers to use effectively. This makes it hard to find good examples of text-video pairs that can help train AI models to generate videos accurately. Additionally, many current methods do not fully utilize the information in the text prompts, leading to less effective video generation.
What's the solution?
To solve these issues, the authors created OpenVid-1M, which includes over 1 million high-quality text-video pairs with detailed captions. They also developed a new model called the Multi-modal Video Diffusion Transformer (MVDiT), which can better understand both the visual and textual information needed for generating videos. This dataset and model allow researchers to create clearer and more accurate videos from text descriptions.
Why it matters?
This research is important because it provides a much-needed resource for improving T2V generation, which has many applications, such as in education, entertainment, and marketing. By offering a high-quality dataset and an advanced model, it helps push the boundaries of what AI can achieve in video generation, making it easier for developers to create engaging content.
Abstract
Text-to-video (T2V) generation has recently garnered significant attention thanks to the large multi-modality model Sora. However, T2V generation still faces two important challenges: 1) Lacking a precise open sourced high-quality dataset. The previous popular video datasets, e.g. WebVid-10M and Panda-70M, are either with low quality or too large for most research institutions. Therefore, it is challenging but crucial to collect a precise high-quality text-video pairs for T2V generation. 2) Ignoring to fully utilize textual information. Recent T2V methods have focused on vision transformers, using a simple cross attention module for video generation, which falls short of thoroughly extracting semantic information from text prompt. To address these issues, we introduce OpenVid-1M, a precise high-quality dataset with expressive captions. This open-scenario dataset contains over 1 million text-video pairs, facilitating research on T2V generation. Furthermore, we curate 433K 1080p videos from OpenVid-1M to create OpenVidHD-0.4M, advancing high-definition video generation. Additionally, we propose a novel Multi-modal Video Diffusion Transformer (MVDiT) capable of mining both structure information from visual tokens and semantic information from text tokens. Extensive experiments and ablation studies verify the superiority of OpenVid-1M over previous datasets and the effectiveness of our MVDiT.