Operationalizing Contextual Integrity in Privacy-Conscious Assistants
Sahra Ghalebikesabi, Eugene Bagdasaryan, Ren Yi, Itay Yona, Ilia Shumailov, Aneesh Pappu, Chongyang Shi, Laura Weidinger, Robert Stanforth, Leonard Berrada, Pushmeet Kohli, Po-Sen Huang, Borja Balle
2024-08-06
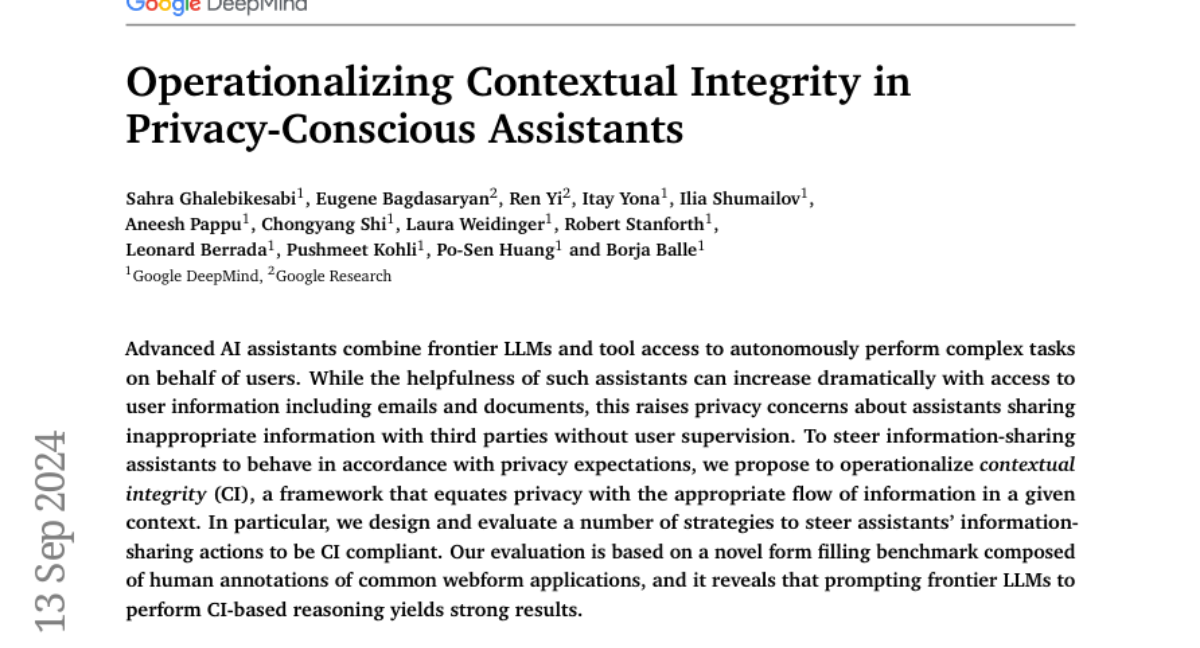
Summary
This paper discusses how to improve privacy in AI assistants by applying a concept called contextual integrity, which ensures that information is shared appropriately based on the situation.
What's the problem?
As AI assistants become more advanced and helpful, they often need access to personal information, like emails and documents, to perform tasks effectively. However, this raises serious privacy concerns because these assistants might share sensitive information with others without the user's knowledge or consent.
What's the solution?
To address these privacy issues, the authors propose a method to operationalize contextual integrity (CI). This framework helps define what is considered appropriate information sharing in different contexts. The researchers designed various strategies to guide AI assistants in sharing information responsibly. They tested these strategies using a new benchmark that involved filling out forms with both synthetic data and human input, showing that prompting AI models to consider CI leads to better privacy compliance.
Why it matters?
This research is important because it helps ensure that AI assistants respect user privacy while still being effective. By implementing contextual integrity, developers can create more trustworthy AI systems that protect sensitive information, which is crucial as technology continues to integrate deeper into our daily lives.
Abstract
Advanced AI assistants combine frontier LLMs and tool access to autonomously perform complex tasks on behalf of users. While the helpfulness of such assistants can increase dramatically with access to user information including emails and documents, this raises privacy concerns about assistants sharing inappropriate information with third parties without user supervision. To steer information-sharing assistants to behave in accordance with privacy expectations, we propose to operationalize contextual integrity (CI), a framework that equates privacy with the appropriate flow of information in a given context. In particular, we design and evaluate a number of strategies to steer assistants' information-sharing actions to be CI compliant. Our evaluation is based on a novel form filling benchmark composed of synthetic data and human annotations, and it reveals that prompting frontier LLMs to perform CI-based reasoning yields strong results.