OSV: One Step is Enough for High-Quality Image to Video Generation
Xiaofeng Mao, Zhengkai Jiang, Fu-Yun Wang, Wenbing Zhu, Jiangning Zhang, Hao Chen, Mingmin Chi, Yabiao Wang
2024-09-18
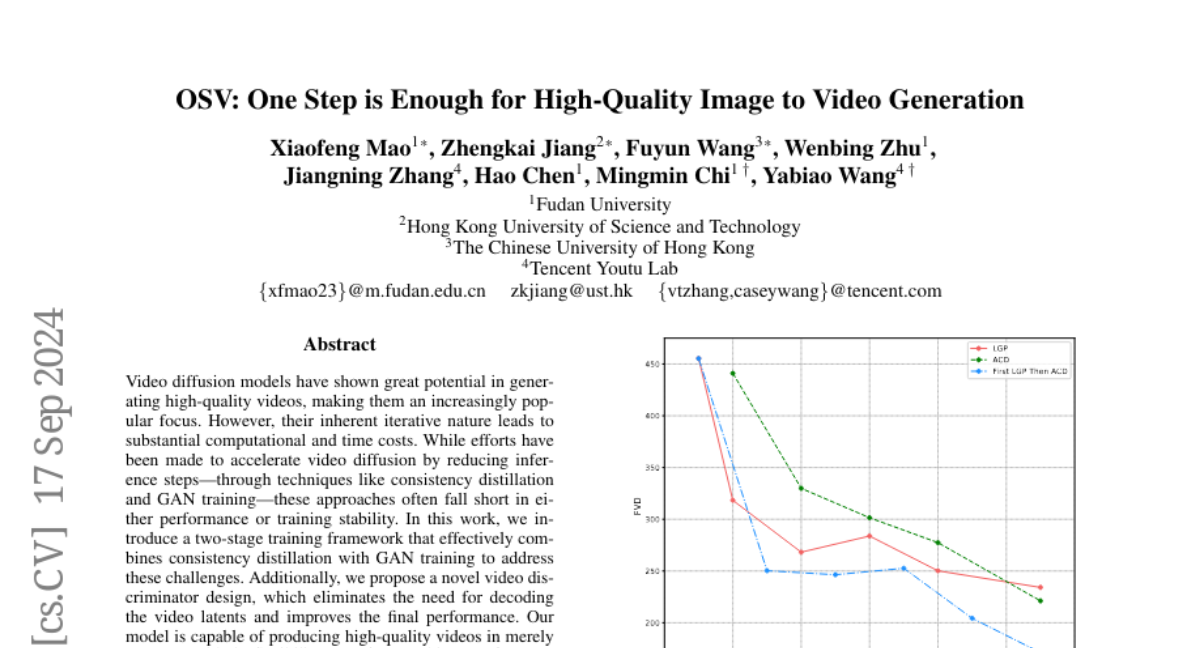
Summary
This paper introduces OSV, a new model that can generate high-quality videos from images in just one step, making the video creation process faster and more efficient.
What's the problem?
Traditional video generation models often require multiple steps to create a video, which can be slow and resource-intensive. This iterative process can lead to high computational costs and longer wait times for users, making it less practical for real-time applications.
What's the solution?
OSV addresses these challenges by using a two-stage training framework that combines techniques from consistency distillation and GAN (Generative Adversarial Network) training. This allows the model to generate high-quality videos in a single step while still having the option to refine the output further if needed. Additionally, OSV introduces a new design for evaluating video quality that eliminates unnecessary steps in the process, resulting in faster and better video generation.
Why it matters?
This research is significant because it simplifies the video creation process, enabling faster production of high-quality videos. By reducing the time and resources needed for video generation, OSV has the potential to benefit various fields such as filmmaking, gaming, and online content creation, making advanced video technology more accessible.
Abstract
Video diffusion models have shown great potential in generating high-quality videos, making them an increasingly popular focus. However, their inherent iterative nature leads to substantial computational and time costs. While efforts have been made to accelerate video diffusion by reducing inference steps (through techniques like consistency distillation) and GAN training (these approaches often fall short in either performance or training stability). In this work, we introduce a two-stage training framework that effectively combines consistency distillation with GAN training to address these challenges. Additionally, we propose a novel video discriminator design, which eliminates the need for decoding the video latents and improves the final performance. Our model is capable of producing high-quality videos in merely one-step, with the flexibility to perform multi-step refinement for further performance enhancement. Our quantitative evaluation on the OpenWebVid-1M benchmark shows that our model significantly outperforms existing methods. Notably, our 1-step performance(FVD 171.15) exceeds the 8-step performance of the consistency distillation based method, AnimateLCM (FVD 184.79), and approaches the 25-step performance of advanced Stable Video Diffusion (FVD 156.94).