Pairwise RM: Perform Best-of-N Sampling with Knockout Tournament
Yantao Liu, Zijun Yao, Rui Min, Yixin Cao, Lei Hou, Juanzi Li
2025-01-23
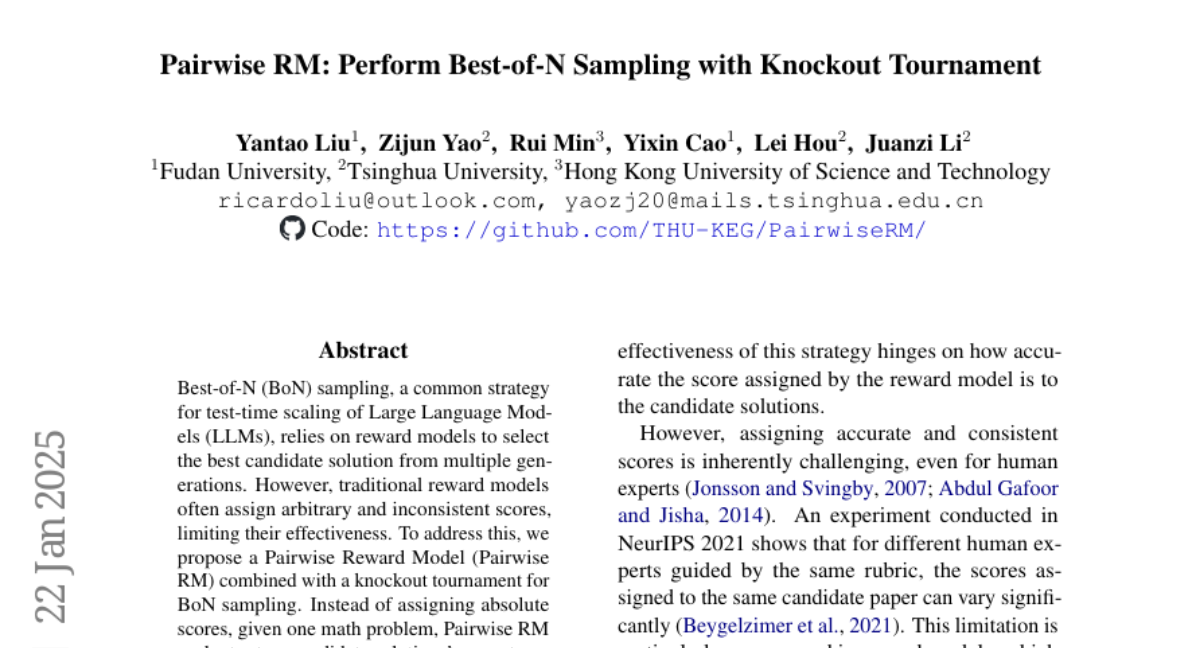
Summary
This paper talks about a new way to make AI language models better at solving math problems. It's called Pairwise RM, and it works by having the AI compare two possible answers to a math problem instead of trying to score each answer individually.
What's the problem?
Current methods for improving AI's math skills use something called Best-of-N sampling, where the AI generates multiple answers and then tries to pick the best one. But the way these AIs judge which answer is best isn't very reliable. It's like having a student solve a math problem multiple times and then guessing which of their answers is correct without really understanding the math.
What's the solution?
The researchers created Pairwise RM, which is like a smart referee for math problems. Instead of trying to grade each answer, it looks at two answers side by side and decides which one is better. They also set up a tournament system where wrong answers get eliminated until only the best one is left. To teach this AI referee, they created a huge set of math problems with pairs of answers for it to practice on. They used another AI called Gemini to help create this practice set.
Why it matters?
This matters because it could make AI much better at solving complex math problems. Better math-solving AI could help in fields like science, engineering, and finance where accurate calculations are crucial. It's also a step towards making AI think more like humans do when solving problems - comparing options rather than just guessing at scores. This could lead to smarter AI assistants that can help students learn math or help professionals tackle difficult mathematical challenges.
Abstract
Best-of-N (BoN) sampling, a common strategy for test-time scaling of Large Language Models (LLMs), relies on reward models to select the best candidate solution from multiple generations. However, traditional reward models often assign arbitrary and inconsistent scores, limiting their effectiveness. To address this, we propose a Pairwise Reward Model (Pairwise RM) combined with a knockout tournament for BoN sampling. Instead of assigning absolute scores, given one math problem, Pairwise RM evaluates two candidate solutions' correctness simultaneously. This approach eliminates the need for arbitrary scoring and enables cross-validation of solutions through parallel comparison. In the knockout tournament, Pairwise RM conducts pairwise comparisons between candidate solutions and eliminates the incorrect ones iteratively. We construct \ourdataset, a large-scale dataset of 443K pairwise comparisons derived from NumiaMath and annotated using gemini-1.5-flash, and train the Pairwise RM via supervised fine-tuning. Experiments on MATH-500 and the Olympiad Bench demonstrate significant improvements over traditional discriminative reward models. And a 40\% to 60\% relative improvement is achieved on the top 50\% challenging problems.