Pandora: Towards General World Model with Natural Language Actions and Video States
Jiannan Xiang, Guangyi Liu, Yi Gu, Qiyue Gao, Yuting Ning, Yuheng Zha, Zeyu Feng, Tianhua Tao, Shibo Hao, Yemin Shi, Zhengzhong Liu, Eric P. Xing, Zhiting Hu
2024-06-18
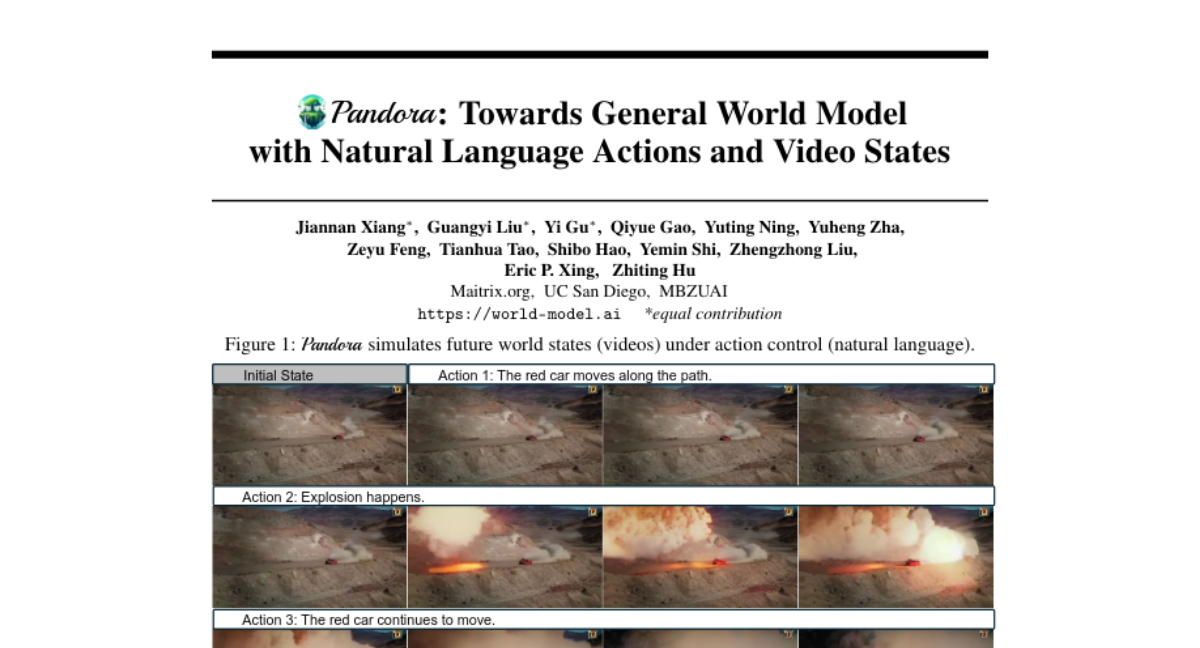
Summary
This paper introduces Pandora, a new model designed to create a general world model that can simulate different environments and respond to actions described in natural language. It combines video generation with the ability to control these simulations in real-time.
What's the problem?
Current models that understand the world either focus on language or video but struggle to combine both effectively. Large language models (LLMs) are limited in their understanding of physical environments, while video models cannot easily control actions within those environments. This makes it difficult to create interactive experiences that feel realistic and responsive.
What's the solution?
To address these issues, the authors developed Pandora, which is a hybrid model that uses both autoregressive and diffusion techniques to generate videos based on user-defined actions. Pandora can simulate different scenarios—like indoor or outdoor settings—and allows users to control what happens next by typing commands in natural language. The model was built by combining a pretrained language model with a pretrained video model, which saves time and resources compared to starting from scratch. It also underwent extensive training to ensure it could handle a wide range of situations and maintain consistency in the videos it generates.
Why it matters?
This research is important because it represents a significant step toward creating more advanced AI systems that can simulate real-world environments and interact with users in meaningful ways. By enabling real-time control over video simulations through natural language, Pandora could enhance applications in gaming, virtual reality, education, and training simulations, making them more engaging and realistic.
Abstract
World models simulate future states of the world in response to different actions. They facilitate interactive content creation and provides a foundation for grounded, long-horizon reasoning. Current foundation models do not fully meet the capabilities of general world models: large language models (LLMs) are constrained by their reliance on language modality and their limited understanding of the physical world, while video models lack interactive action control over the world simulations. This paper makes a step towards building a general world model by introducing Pandora, a hybrid autoregressive-diffusion model that simulates world states by generating videos and allows real-time control with free-text actions. Pandora achieves domain generality, video consistency, and controllability through large-scale pretraining and instruction tuning. Crucially, Pandora bypasses the cost of training-from-scratch by integrating a pretrained LLM (7B) and a pretrained video model, requiring only additional lightweight finetuning. We illustrate extensive outputs by Pandora across diverse domains (indoor/outdoor, natural/urban, human/robot, 2D/3D, etc.). The results indicate great potential of building stronger general world models with larger-scale training.