Pangea: A Fully Open Multilingual Multimodal LLM for 39 Languages
Xiang Yue, Yueqi Song, Akari Asai, Seungone Kim, Jean de Dieu Nyandwi, Simran Khanuja, Anjali Kantharuban, Lintang Sutawika, Sathyanarayanan Ramamoorthy, Graham Neubig
2024-10-22
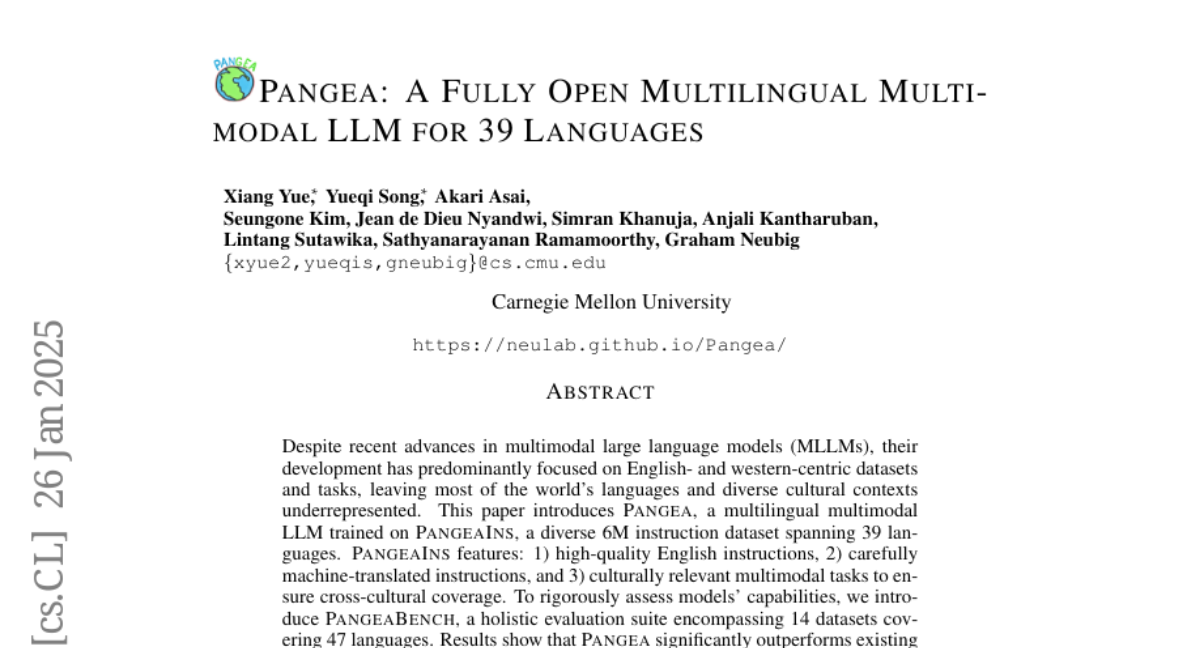
Summary
This paper introduces Pangea, a fully open multilingual multimodal language model designed to understand and generate content in 39 different languages, addressing the lack of representation for non-English languages in AI.
What's the problem?
Most existing language models focus heavily on English and Western contexts, leaving many languages and cultures underrepresented. This can lead to biases in AI applications, making them less effective or useful for people who speak other languages or come from different cultural backgrounds.
What's the solution?
To tackle this issue, the authors developed Pangea, which is trained on a diverse dataset called PangeaIns that includes 6 million instructions in 39 languages. This dataset combines high-quality English instructions, carefully machine-translated instructions, and culturally relevant tasks to ensure that the model understands various cultures. The authors also created a benchmark called PangeaBench to evaluate the model's performance across different languages and tasks. Pangea significantly outperforms existing models in both multilingual and multicultural contexts.
Why it matters?
This research is important because it promotes inclusivity and accessibility in AI technologies. By making Pangea open-source, the authors allow researchers and developers worldwide to use and improve upon this model, helping to ensure that advanced language understanding is available to a broader audience. This can lead to more equitable access to technology and better representation of diverse cultures in AI applications.
Abstract
Despite recent advances in multimodal large language models (MLLMs), their development has predominantly focused on English- and western-centric datasets and tasks, leaving most of the world's languages and diverse cultural contexts underrepresented. This paper introduces Pangea, a multilingual multimodal LLM trained on PangeaIns, a diverse 6M instruction dataset spanning 39 languages. PangeaIns features: 1) high-quality English instructions, 2) carefully machine-translated instructions, and 3) culturally relevant multimodal tasks to ensure cross-cultural coverage. To rigorously assess models' capabilities, we introduce PangeaBench, a holistic evaluation suite encompassing 14 datasets covering 47 languages. Results show that Pangea significantly outperforms existing open-source models in multilingual settings and diverse cultural contexts. Ablation studies further reveal the importance of English data proportions, language popularity, and the number of multimodal training samples on overall performance. We fully open-source our data, code, and trained checkpoints, to facilitate the development of inclusive and robust multilingual MLLMs, promoting equity and accessibility across a broader linguistic and cultural spectrum.