Panoramic Interests: Stylistic-Content Aware Personalized Headline Generation
Junhong Lian, Xiang Ao, Xinyu Liu, Yang Liu, Qing He
2025-01-22
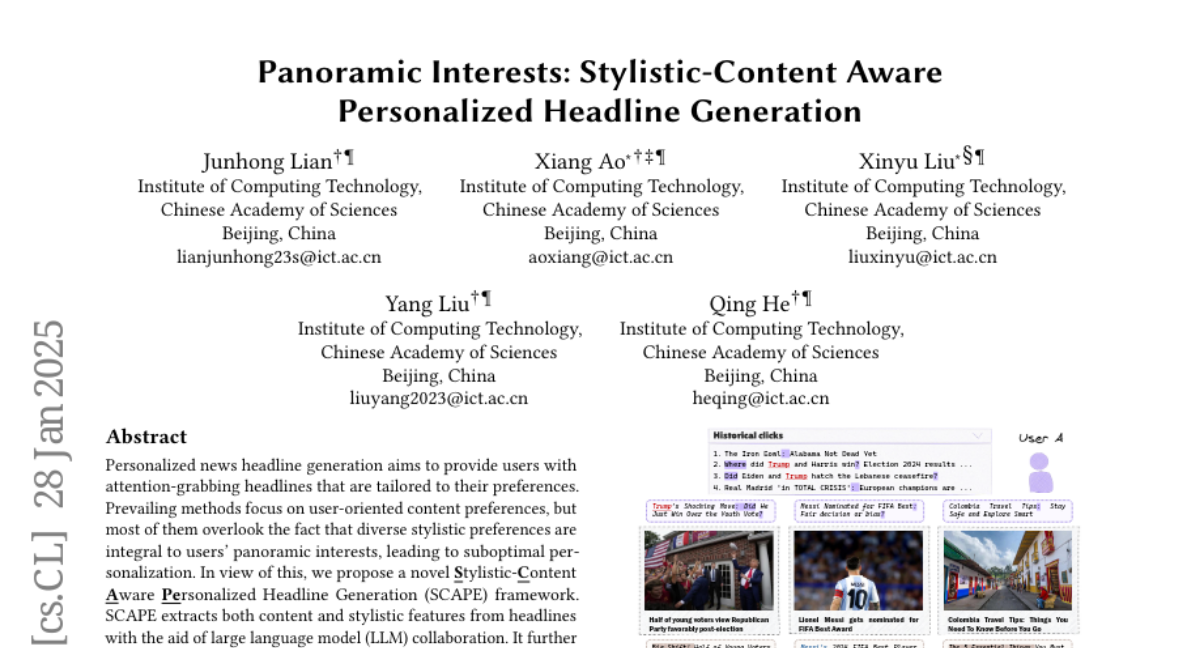
Summary
This paper talks about a new way to test if AI systems that understand both images and text (called Vision-Language Models or VLMs) are safe to use. The researchers created a special test called MSTS to check if these AI systems might give dangerous advice when shown certain combinations of pictures and words.
What's the problem?
VLMs are becoming more common in things like chat assistants, but they might accidentally give harmful advice or encourage unsafe behavior if they're not properly designed. Until now, there hasn't been a good way to test if these AI systems are safe, especially when it comes to understanding both images and text together.
What's the solution?
The researchers created MSTS, which stands for Multimodal Safety Test Suite. It's like a big quiz for AI with 400 questions that combine pictures and text in tricky ways. These questions cover 40 different types of potential dangers. They used MSTS to test several VLMs and found that many of them had safety problems. They also translated the test into ten different languages and found that the AI systems were more likely to give unsafe answers in languages other than English.
Why it matters?
This matters because as AI systems that can understand both images and text become more common in our everyday lives, we need to make sure they're safe to use. MSTS gives developers a way to check their AI for potential dangers before releasing it to the public. It also shows that current safety measures might not be good enough, especially when it comes to different languages or when combining images and text. This research could help make AI assistants and other applications safer and more trustworthy for everyone to use.
Abstract
Personalized news headline generation aims to provide users with attention-grabbing headlines that are tailored to their preferences. Prevailing methods focus on user-oriented content preferences, but most of them overlook the fact that diverse stylistic preferences are integral to users' panoramic interests, leading to suboptimal personalization. In view of this, we propose a novel Stylistic-Content Aware Personalized Headline Generation (SCAPE) framework. SCAPE extracts both content and stylistic features from headlines with the aid of large language model (LLM) collaboration. It further adaptively integrates users' long- and short-term interests through a contrastive learning-based hierarchical fusion network. By incorporating the panoramic interests into the headline generator, SCAPE reflects users' stylistic-content preferences during the generation process. Extensive experiments on the real-world dataset PENS demonstrate the superiority of SCAPE over baselines.