PartCraft: Crafting Creative Objects by Parts
Kam Woh Ng, Xiatian Zhu, Yi-Zhe Song, Tao Xiang
2024-07-09
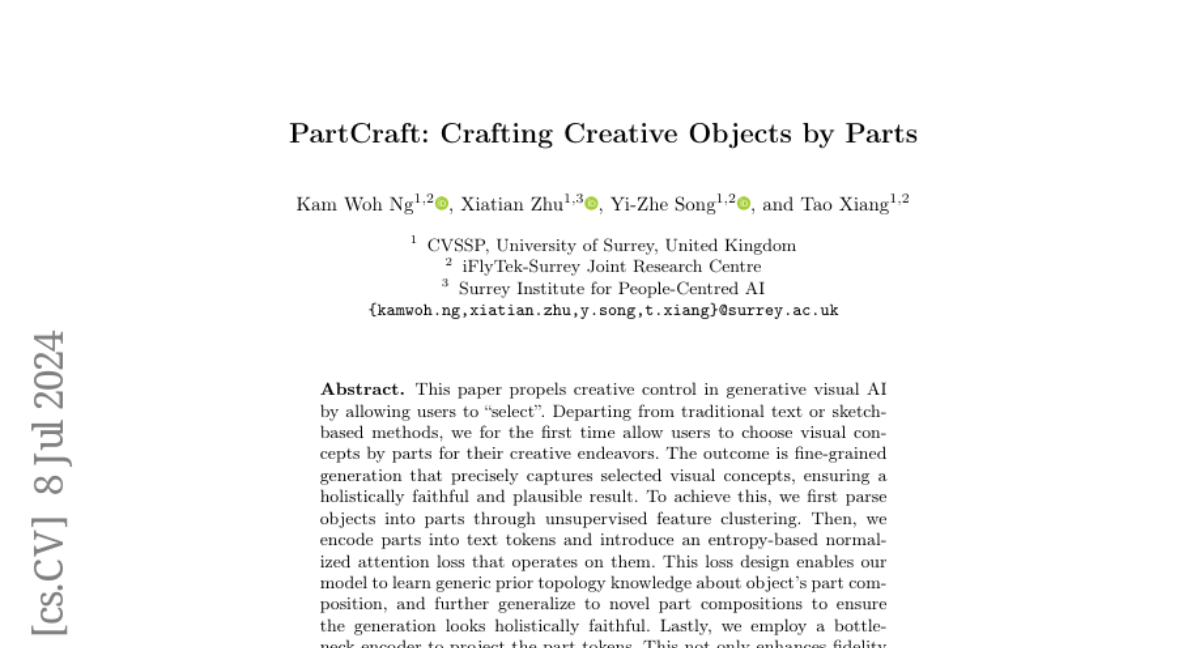
Summary
This paper talks about PartCraft, a new system that allows users to create visual objects by selecting specific parts instead of just using text descriptions or sketches. This approach enables more detailed and accurate generation of creative designs.
What's the problem?
The main problem is that traditional methods for generating images often rely on broad text prompts or rough sketches, which can limit the user's ability to control the specific details of the objects they want to create. This can lead to results that don't fully match the user's vision or expectations.
What's the solution?
To solve this issue, the authors developed PartCraft, which lets users choose visual parts of objects for their designs. They first break down objects into smaller parts using a technique called unsupervised feature clustering. Then, these parts are turned into text tokens, and an advanced loss function helps the model learn how different parts can fit together. This process allows the model to generate new objects that look realistic and cohesive based on the selected parts. Additionally, a bottleneck encoder is used to improve the quality of the generated images and speed up learning by sharing knowledge between different parts.
Why it matters?
This research is important because it enhances creative control in generative visual AI, allowing users to create highly customized and innovative designs. By enabling detailed part selection, PartCraft can be particularly useful in fields like product design, art, and animation, where precise customization is essential for achieving desired outcomes.
Abstract
This paper propels creative control in generative visual AI by allowing users to "select". Departing from traditional text or sketch-based methods, we for the first time allow users to choose visual concepts by parts for their creative endeavors. The outcome is fine-grained generation that precisely captures selected visual concepts, ensuring a holistically faithful and plausible result. To achieve this, we first parse objects into parts through unsupervised feature clustering. Then, we encode parts into text tokens and introduce an entropy-based normalized attention loss that operates on them. This loss design enables our model to learn generic prior topology knowledge about object's part composition, and further generalize to novel part compositions to ensure the generation looks holistically faithful. Lastly, we employ a bottleneck encoder to project the part tokens. This not only enhances fidelity but also accelerates learning, by leveraging shared knowledge and facilitating information exchange among instances. Visual results in the paper and supplementary material showcase the compelling power of PartCraft in crafting highly customized, innovative creations, exemplified by the "charming" and creative birds. Code is released at https://github.com/kamwoh/partcraft.