PeriodWave: Multi-Period Flow Matching for High-Fidelity Waveform Generation
Sang-Hoon Lee, Ha-Yeong Choi, Seong-Whan Lee
2024-08-15
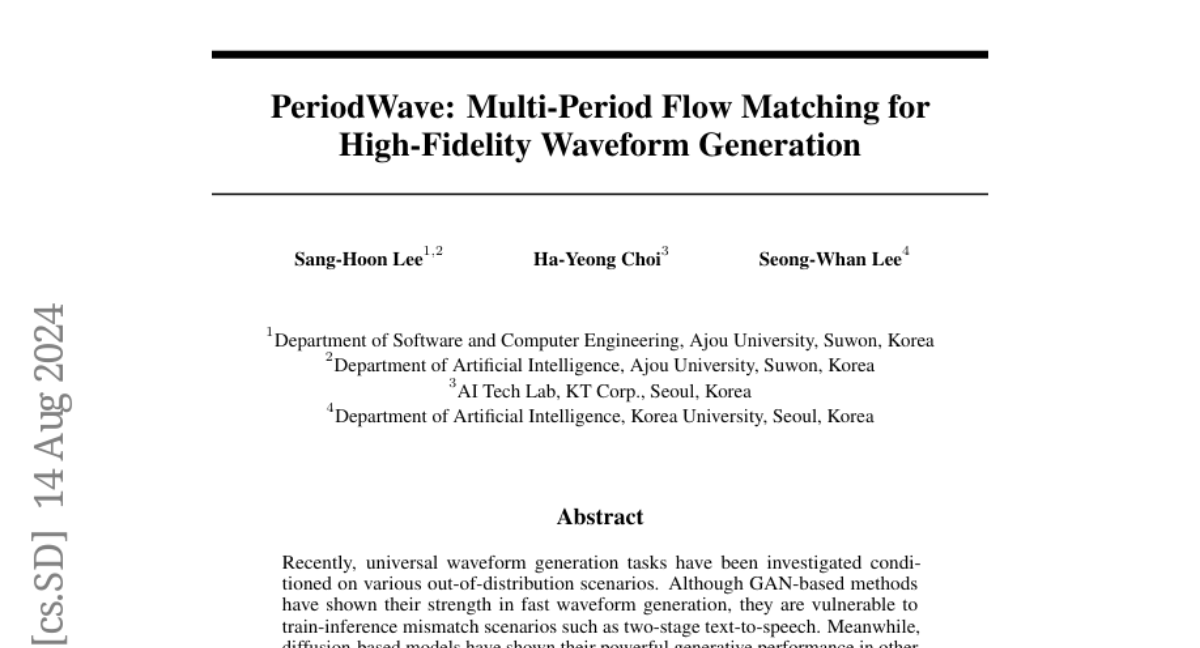
Summary
This paper introduces PeriodWave, a new model for generating high-quality waveforms that improve the way sound is created and manipulated in audio applications.
What's the problem?
Current methods for generating waveforms, especially in tasks like text-to-speech, often face challenges. While some techniques are fast, they can produce poor quality when faced with different scenarios. Other methods may generate high-quality results but are slow and inefficient, making them less practical for real-time applications.
What's the solution?
PeriodWave addresses these issues by using a unique approach that focuses on the periodic features of waveforms. It includes a special estimator that captures these features effectively and allows for the generation of waveforms based on user input. The model also introduces strategies to manage computational costs while improving quality, such as using a single period-conditional estimator and discrete wavelet transforms to enhance detail without losing clarity.
Why it matters?
This research is significant because it enhances the ability to generate realistic audio waveforms quickly and efficiently. This can lead to better applications in areas like music production, virtual assistants, and any technology that relies on high-quality sound generation.
Abstract
Recently, universal waveform generation tasks have been investigated conditioned on various out-of-distribution scenarios. Although GAN-based methods have shown their strength in fast waveform generation, they are vulnerable to train-inference mismatch scenarios such as two-stage text-to-speech. Meanwhile, diffusion-based models have shown their powerful generative performance in other domains; however, they stay out of the limelight due to slow inference speed in waveform generation tasks. Above all, there is no generator architecture that can explicitly disentangle the natural periodic features of high-resolution waveform signals. In this paper, we propose PeriodWave, a novel universal waveform generation model. First, we introduce a period-aware flow matching estimator that can capture the periodic features of the waveform signal when estimating the vector fields. Additionally, we utilize a multi-period estimator that avoids overlaps to capture different periodic features of waveform signals. Although increasing the number of periods can improve the performance significantly, this requires more computational costs. To reduce this issue, we also propose a single period-conditional universal estimator that can feed-forward parallel by period-wise batch inference. Additionally, we utilize discrete wavelet transform to losslessly disentangle the frequency information of waveform signals for high-frequency modeling, and introduce FreeU to reduce the high-frequency noise for waveform generation. The experimental results demonstrated that our model outperforms the previous models both in Mel-spectrogram reconstruction and text-to-speech tasks. All source code will be available at https://github.com/sh-lee-prml/PeriodWave.