PERSONA: A Reproducible Testbed for Pluralistic Alignment
Louis Castricato, Nathan Lile, Rafael Rafailov, Jan-Philipp Fränken, Chelsea Finn
2024-07-25
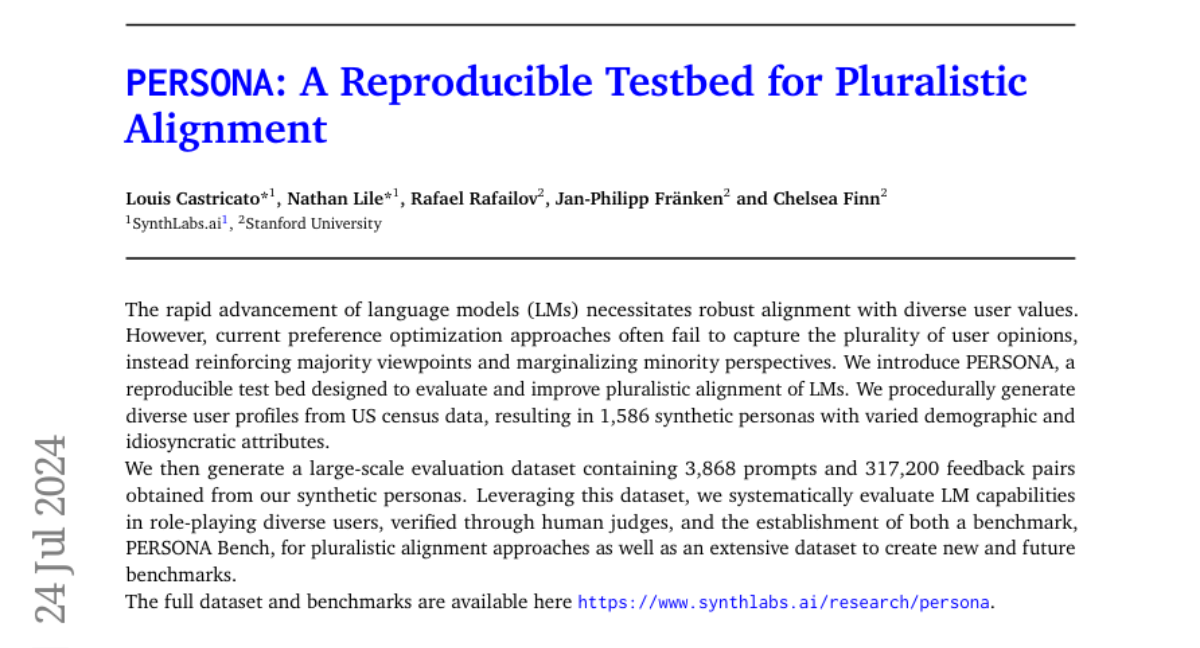
Summary
This paper introduces PERSONA, a new platform designed to improve how language models (LMs) understand and align with diverse user values. It creates a testbed to evaluate LMs by using synthetic user profiles that represent a wide range of opinions and backgrounds.
What's the problem?
As language models become more advanced, they need to better reflect the diverse values and preferences of users. However, current methods often focus on the majority opinions, which can lead to the marginalization of minority perspectives. This means that the models may not respond fairly or accurately to all users, limiting their effectiveness in real-world applications.
What's the solution?
PERSONA tackles this problem by generating a large number of synthetic user profiles based on US census data, resulting in 1,586 unique personas with different backgrounds and opinions. The researchers then create a dataset containing 3,868 prompts and 317,200 feedback pairs from these personas. This allows for systematic evaluation of how well language models can role-play different users and respond to their specific needs. The platform also includes a benchmark called PERSONA Bench for assessing pluralistic alignment in LMs.
Why it matters?
This research is important because it helps ensure that language models can engage fairly and effectively with a wide variety of users. By providing a reproducible framework for testing and improving LMs on diverse perspectives, PERSONA aims to make AI systems more inclusive and representative. This can lead to better interactions between AI and users in applications such as customer service, education, and content creation.
Abstract
The rapid advancement of language models (LMs) necessitates robust alignment with diverse user values. However, current preference optimization approaches often fail to capture the plurality of user opinions, instead reinforcing majority viewpoints and marginalizing minority perspectives. We introduce PERSONA, a reproducible test bed designed to evaluate and improve pluralistic alignment of LMs. We procedurally generate diverse user profiles from US census data, resulting in 1,586 synthetic personas with varied demographic and idiosyncratic attributes. We then generate a large-scale evaluation dataset containing 3,868 prompts and 317,200 feedback pairs obtained from our synthetic personas. Leveraging this dataset, we systematically evaluate LM capabilities in role-playing diverse users, verified through human judges, and the establishment of both a benchmark, PERSONA Bench, for pluralistic alignment approaches as well as an extensive dataset to create new and future benchmarks. The full dataset and benchmarks are available here: https://www.synthlabs.ai/research/persona.