Personalize Anything for Free with Diffusion Transformer
Haoran Feng, Zehuan Huang, Lin Li, Hairong Lv, Lu Sheng
2025-03-18
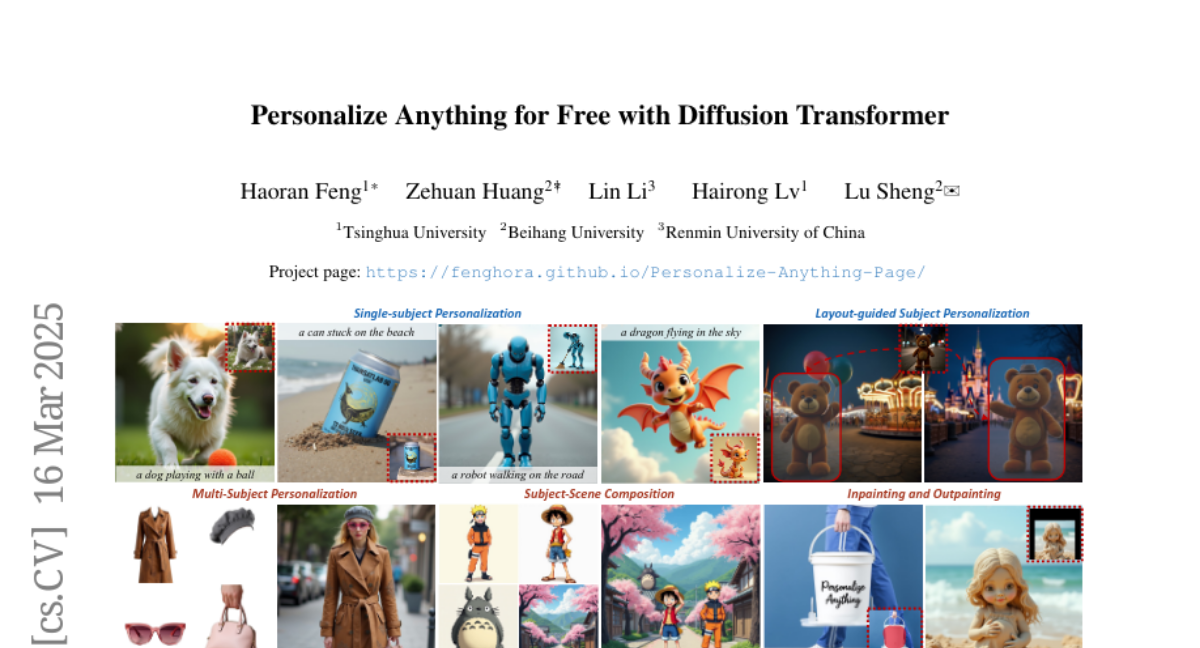
Summary
This paper presents Personalize Anything, a new method for creating personalized images using AI models called Diffusion Transformers (DiTs). It allows users to generate images of specific subjects and edit them in various ways without needing extensive training.
What's the problem?
Existing methods for personalized image generation often struggle with maintaining the subject's identity, have limited applicability, or are not compatible with Diffusion Transformer models. Training-based methods are also computationally expensive.
What's the solution?
Personalize Anything leverages the DiT architecture and introduces a technique where tokens from a reference subject are used to replace denoising tokens, enabling zero-shot subject reconstruction. It uses timestep-adaptive token replacement to ensure subject consistency and patch perturbation strategies to increase structural diversity. This allows for layout-guided generation, multi-subject personalization, and mask-controlled editing.
Why it matters?
This work matters because it offers a more efficient and versatile way to personalize image generation using DiTs, allowing users to easily create and edit images of specific subjects with greater control and fidelity.
Abstract
Personalized image generation aims to produce images of user-specified concepts while enabling flexible editing. Recent training-free approaches, while exhibit higher computational efficiency than training-based methods, struggle with identity preservation, applicability, and compatibility with diffusion transformers (DiTs). In this paper, we uncover the untapped potential of DiT, where simply replacing denoising tokens with those of a reference subject achieves zero-shot subject reconstruction. This simple yet effective feature injection technique unlocks diverse scenarios, from personalization to image editing. Building upon this observation, we propose Personalize Anything, a training-free framework that achieves personalized image generation in DiT through: 1) timestep-adaptive token replacement that enforces subject consistency via early-stage injection and enhances flexibility through late-stage regularization, and 2) patch perturbation strategies to boost structural diversity. Our method seamlessly supports layout-guided generation, multi-subject personalization, and mask-controlled editing. Evaluations demonstrate state-of-the-art performance in identity preservation and versatility. Our work establishes new insights into DiTs while delivering a practical paradigm for efficient personalization.