Phi-3 Safety Post-Training: Aligning Language Models with a "Break-Fix" Cycle
Emman Haider, Daniel Perez-Becker, Thomas Portet, Piyush Madan, Amit Garg, David Majercak, Wen Wen, Dongwoo Kim, Ziyi Yang, Jianwen Zhang, Hiteshi Sharma, Blake Bullwinkel, Martin Pouliot, Amanda Minnich, Shiven Chawla, Solianna Herrera, Shahed Warreth, Maggie Engler, Gary Lopez, Nina Chikanov, Raja Sekhar Rao Dheekonda, Bolor-Erdene Jagdagdorj
2024-07-22
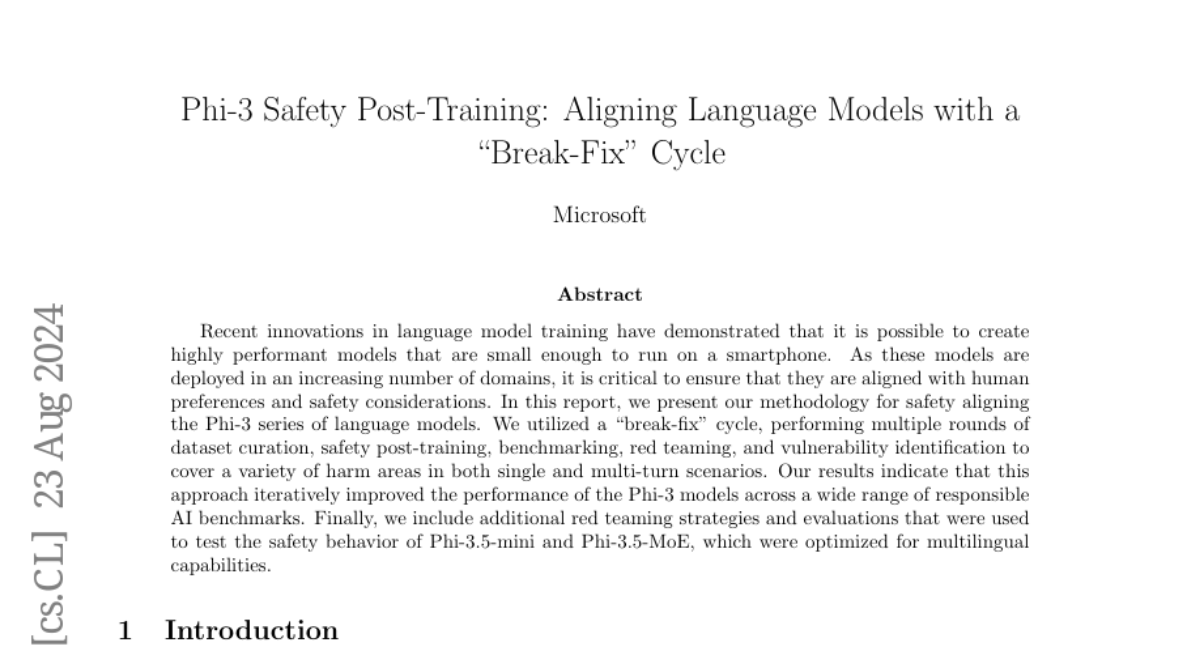
Summary
This paper discusses a method called 'break-fix' cycle used to improve the safety and alignment of the Phi-3 series of language models. It focuses on ensuring that these models operate in ways that are safe and aligned with human preferences as they are deployed in various applications.
What's the problem?
As language models become more advanced and are used in many different areas, it is crucial to make sure they behave safely and according to human values. However, ensuring this alignment is challenging because models can produce unexpected or harmful outputs if not properly trained and evaluated.
What's the solution?
The authors implemented a 'break-fix' cycle, which involves repeatedly refining the model through several steps: curating datasets for safety, performing safety post-training, benchmarking performance, conducting red teaming (where teams test the model for vulnerabilities), and identifying potential risks. This iterative process allows for continuous improvement, helping the Phi-3 models learn to generate safer responses across various scenarios.
Why it matters?
This research is important because it enhances the reliability of language models in real-world applications. By focusing on safety and alignment, the study contributes to developing AI systems that users can trust, which is essential for their acceptance and effectiveness in sensitive areas like healthcare, education, and customer service.
Abstract
Recent innovations in language model training have demonstrated that it is possible to create highly performant models that are small enough to run on a smartphone. As these models are deployed in an increasing number of domains, it is critical to ensure that they are aligned with human preferences and safety considerations. In this report, we present our methodology for safety aligning the Phi-3 series of language models. We utilized a "break-fix" cycle, performing multiple rounds of dataset curation, safety post-training, benchmarking, red teaming, and vulnerability identification to cover a variety of harm areas in both single and multi-turn scenarios. Our results indicate that this approach iteratively improved the performance of the Phi-3 models across a wide range of responsible AI benchmarks.