Phidias: A Generative Model for Creating 3D Content from Text, Image, and 3D Conditions with Reference-Augmented Diffusion
Zhenwei Wang, Tengfei Wang, Zexin He, Gerhard Hancke, Ziwei Liu, Rynson W. H. Lau
2024-09-18
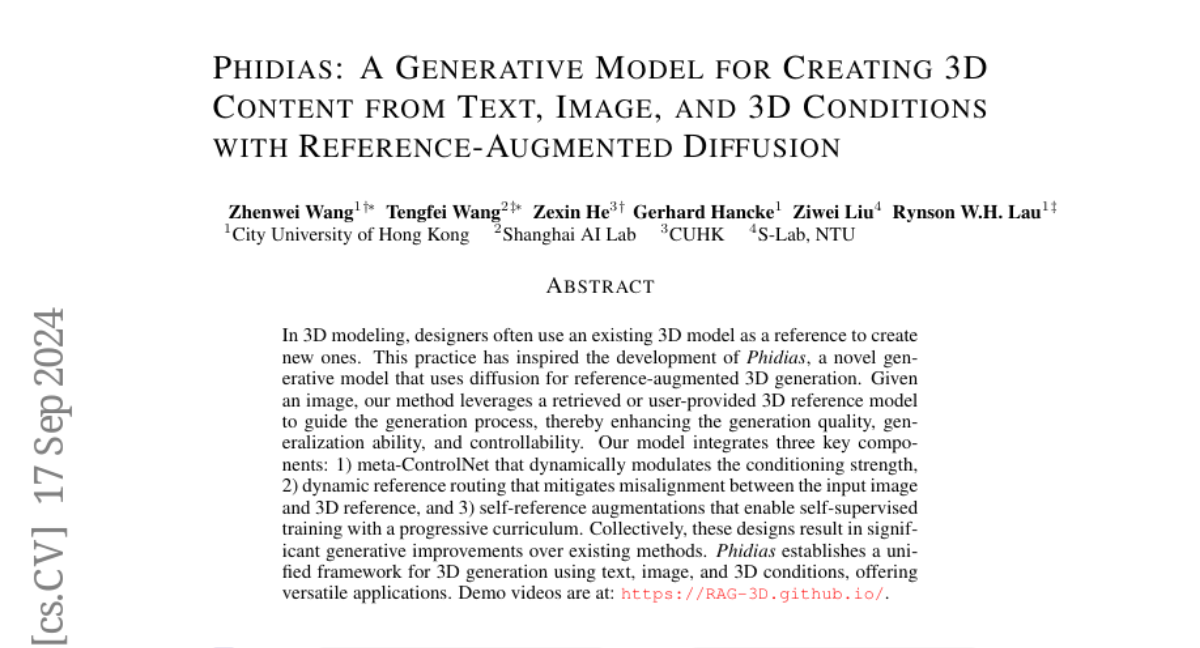
Summary
This paper introduces Phidias, a new model that generates 3D content from text, images, and existing 3D models using a technique called reference-augmented diffusion.
What's the problem?
Creating new 3D models can be challenging, especially when designers need to ensure that the new models look accurate and consistent. Existing methods often require complex processes and may not effectively use reference models, which can lead to issues with quality and alignment between the input and the generated output.
What's the solution?
Phidias improves the process by integrating a reference model that designers can provide or retrieve. It uses three main components: a meta-ControlNet to adjust how much influence the reference has, dynamic reference routing to ensure the input image aligns well with the 3D reference, and self-reference augmentations for better training. This allows Phidias to generate high-quality 3D models more efficiently and accurately than previous methods.
Why it matters?
This research is significant because it simplifies the creation of 3D models while enhancing their quality and versatility. By allowing users to generate 3D content from multiple input types, Phidias can be used in various applications such as video games, animation, and virtual reality, making it a valuable tool for designers and artists.
Abstract
In 3D modeling, designers often use an existing 3D model as a reference to create new ones. This practice has inspired the development of Phidias, a novel generative model that uses diffusion for reference-augmented 3D generation. Given an image, our method leverages a retrieved or user-provided 3D reference model to guide the generation process, thereby enhancing the generation quality, generalization ability, and controllability. Our model integrates three key components: 1) meta-ControlNet that dynamically modulates the conditioning strength, 2) dynamic reference routing that mitigates misalignment between the input image and 3D reference, and 3) self-reference augmentations that enable self-supervised training with a progressive curriculum. Collectively, these designs result in a clear improvement over existing methods. Phidias establishes a unified framework for 3D generation using text, image, and 3D conditions with versatile applications.