Physics of Language Models: Part 2.2, How to Learn From Mistakes on Grade-School Math Problems
Tian Ye, Zicheng Xu, Yuanzhi Li, Zeyuan Allen-Zhu
2024-08-30
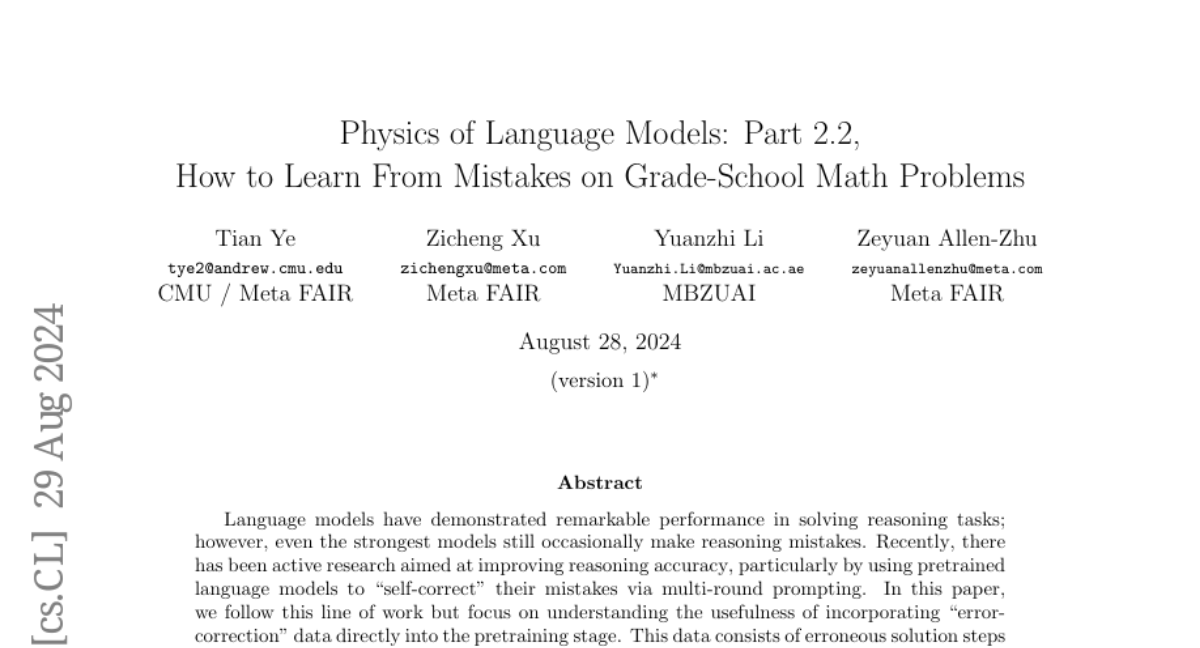
Summary
This paper discusses how to improve language models' ability to solve grade-school math problems by teaching them to learn from their mistakes using error-correction data.
What's the problem?
Even the best language models can make mistakes when solving reasoning tasks, like math problems. They often struggle with accuracy, which can lead to incorrect answers and misunderstandings in more complex tasks.
What's the solution?
The authors propose a method that incorporates error-correction data directly into the training process. This data includes wrong solution steps followed by the correct ones. By using this approach on a synthetic math dataset, they found that models trained with error-correction data performed better at reasoning tasks than those trained only with correct data. This means the models can learn to correct themselves without needing multiple rounds of prompting.
Why it matters?
This research is important because it can lead to more accurate language models, which are used in many applications like tutoring programs, educational tools, and AI assistants. Improving their reasoning skills helps make these technologies more reliable and effective for users.
Abstract
Language models have demonstrated remarkable performance in solving reasoning tasks; however, even the strongest models still occasionally make reasoning mistakes. Recently, there has been active research aimed at improving reasoning accuracy, particularly by using pretrained language models to "self-correct" their mistakes via multi-round prompting. In this paper, we follow this line of work but focus on understanding the usefulness of incorporating "error-correction" data directly into the pretraining stage. This data consists of erroneous solution steps immediately followed by their corrections. Using a synthetic math dataset, we show promising results: this type of pretrain data can help language models achieve higher reasoning accuracy directly (i.e., through simple auto-regression, without multi-round prompting) compared to pretraining on the same amount of error-free data. We also delve into many details, such as (1) how this approach differs from beam search, (2) how such data can be prepared, (3) whether masking is needed on the erroneous tokens, (4) the amount of error required, (5) whether such data can be deferred to the fine-tuning stage, and many others.