PIG: Physics-Informed Gaussians as Adaptive Parametric Mesh Representations
Namgyu Kang, Jaemin Oh, Youngjoon Hong, Eunbyung Park
2024-12-13
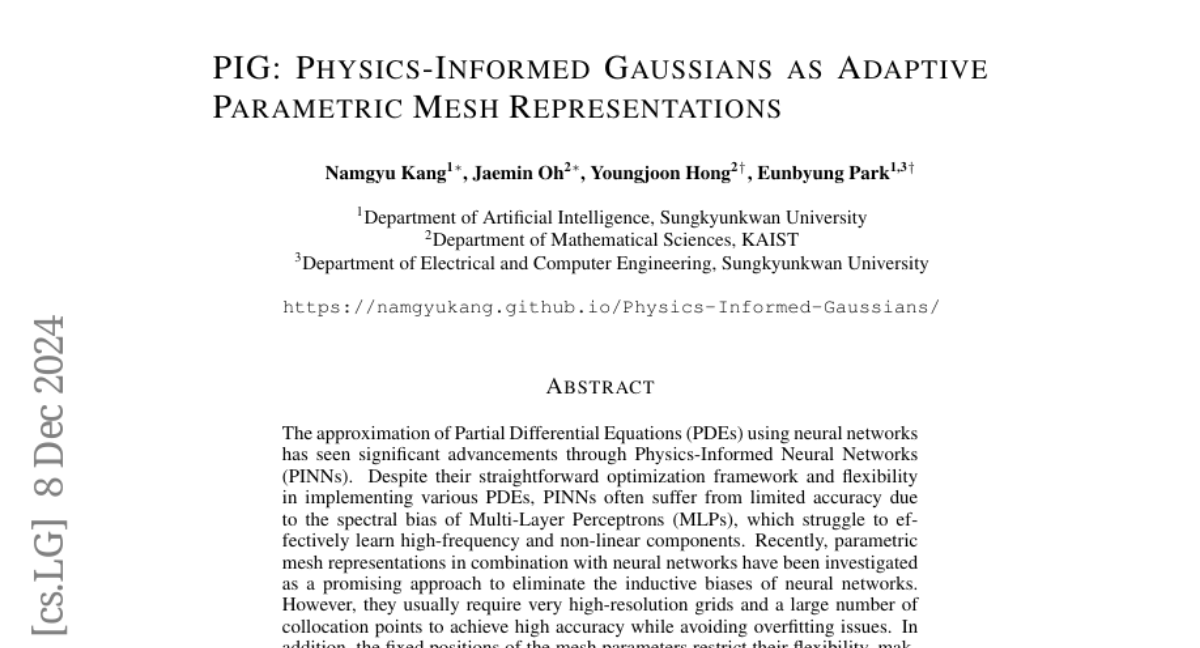
Summary
This paper introduces PIG, which stands for Physics-Informed Gaussians, a new method for solving complex mathematical equations called Partial Differential Equations (PDEs) using adaptive mesh representations.
What's the problem?
Traditional methods for solving PDEs using neural networks often struggle with accuracy because they rely on fixed grid structures and can’t adapt well to the complexities of the equations. This leads to problems like being unable to learn high-frequency details or requiring very high-resolution grids that are computationally expensive and inefficient.
What's the solution?
PIG addresses these issues by using Gaussian functions that can adjust their positions and shapes during the training process. This means that instead of having a static grid, the model can dynamically change how it represents the data based on what it learns. The authors also maintain the same optimization framework used in previous methods, allowing them to benefit from existing techniques while improving accuracy and efficiency. Experimental results show that PIG performs well across various PDEs, demonstrating its effectiveness as a robust tool for solving these complex equations.
Why it matters?
This research is important because it offers a more flexible and efficient way to solve PDEs, which are crucial in many fields like physics, engineering, and finance. By improving how these equations are approximated, PIG can enhance our understanding and modeling of real-world phenomena, leading to better predictions and solutions in various scientific applications.
Abstract
The approximation of Partial Differential Equations (PDEs) using neural networks has seen significant advancements through Physics-Informed Neural Networks (PINNs). Despite their straightforward optimization framework and flexibility in implementing various PDEs, PINNs often suffer from limited accuracy due to the spectral bias of Multi-Layer Perceptrons (MLPs), which struggle to effectively learn high-frequency and non-linear components. Recently, parametric mesh representations in combination with neural networks have been investigated as a promising approach to eliminate the inductive biases of neural networks. However, they usually require very high-resolution grids and a large number of collocation points to achieve high accuracy while avoiding overfitting issues. In addition, the fixed positions of the mesh parameters restrict their flexibility, making it challenging to accurately approximate complex PDEs. To overcome these limitations, we propose Physics-Informed Gaussians (PIGs), which combine feature embeddings using Gaussian functions with a lightweight neural network. Our approach uses trainable parameters for the mean and variance of each Gaussian, allowing for dynamic adjustment of their positions and shapes during training. This adaptability enables our model to optimally approximate PDE solutions, unlike models with fixed parameter positions. Furthermore, the proposed approach maintains the same optimization framework used in PINNs, allowing us to benefit from their excellent properties. Experimental results show the competitive performance of our model across various PDEs, demonstrating its potential as a robust tool for solving complex PDEs. Our project page is available at https://namgyukang.github.io/Physics-Informed-Gaussians/