PIN: A Knowledge-Intensive Dataset for Paired and Interleaved Multimodal Documents
Junjie Wang, Yin Zhang, Yatai Ji, Yuxiang Zhang, Chunyang Jiang, Yubo Wang, Kang Zhu, Zekun Wang, Tiezhen Wang, Wenhao Huang, Jie Fu, Bei Chen, Qunshu Lin, Minghao Liu, Ge Zhang, Wenhu Chen
2024-06-21
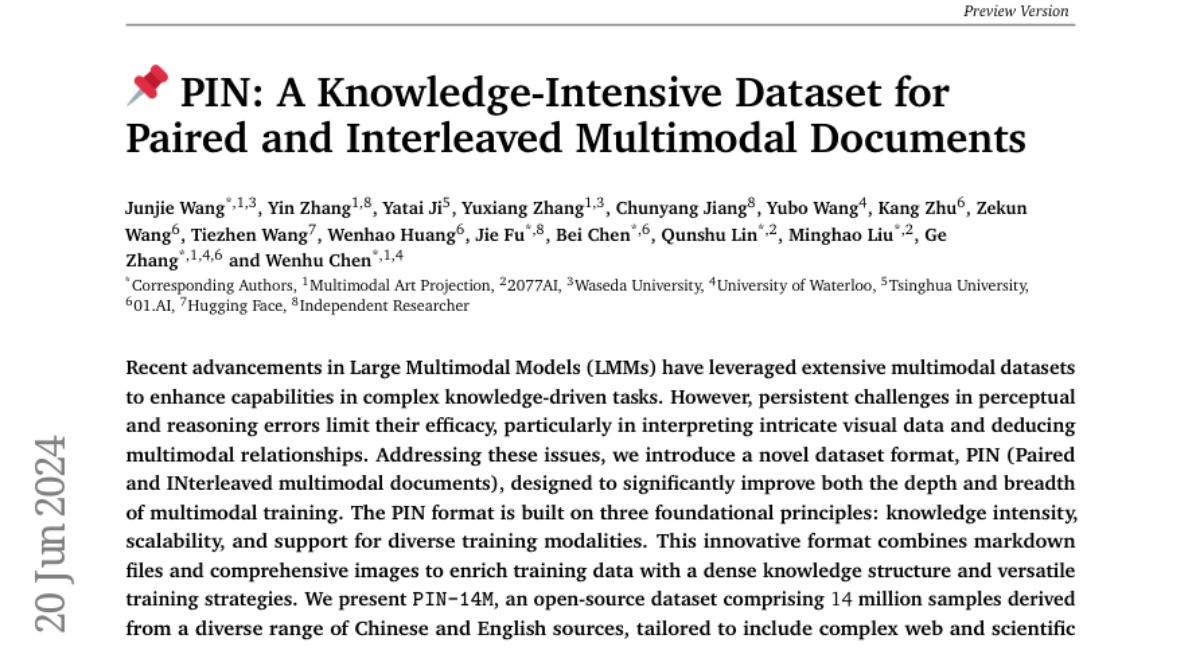
Summary
This paper introduces a new dataset format called PIN (Paired and Interleaved multimodal documents) designed to improve how large multimodal models (LMMs) learn from complex data that includes both text and images.
What's the problem?
While recent advancements in LMMs have made them better at understanding and processing different types of information, there are still challenges. These models often struggle with perceptual errors (how they interpret visual data) and reasoning errors (how they understand relationships between different types of information). This limits their effectiveness in tasks that require deep understanding, especially when dealing with intricate visual data.
What's the solution?
The researchers created the PIN dataset format, which combines markdown text files with detailed images. This combination helps provide rich, structured information that models can learn from. The PIN format is built on three key principles: it emphasizes knowledge intensity (having lots of useful information), scalability (being able to grow and adapt), and support for various training methods. They introduced an open-source dataset called PIN-14M, which contains 14 million samples from diverse sources in Chinese and English, including complex web pages and scientific documents. This dataset is designed to enhance the training of models, making them more robust against common issues.
Why it matters?
This research is important because it offers a new way to train AI models that can handle complex tasks involving both text and images. By improving how these models learn from multimodal data, we can enhance their performance in real-world applications like image recognition, automated content creation, and more advanced AI systems that require a deep understanding of different types of information.
Abstract
Recent advancements in Large Multimodal Models (LMMs) have leveraged extensive multimodal datasets to enhance capabilities in complex knowledge-driven tasks. However, persistent challenges in perceptual and reasoning errors limit their efficacy, particularly in interpreting intricate visual data and deducing multimodal relationships. Addressing these issues, we introduce a novel dataset format, PIN (Paired and INterleaved multimodal documents), designed to significantly improve both the depth and breadth of multimodal training. The PIN format is built on three foundational principles: knowledge intensity, scalability, and support for diverse training modalities. This innovative format combines markdown files and comprehensive images to enrich training data with a dense knowledge structure and versatile training strategies. We present PIN-14M, an open-source dataset comprising 14 million samples derived from a diverse range of Chinese and English sources, tailored to include complex web and scientific content. This dataset is constructed meticulously to ensure data quality and ethical integrity, aiming to facilitate advanced training strategies and improve model robustness against common multimodal training pitfalls. Our initial results, forming the basis of this technical report, suggest significant potential for the PIN format in refining LMM performance, with plans for future expansions and detailed evaluations of its impact on model capabilities.