PiTe: Pixel-Temporal Alignment for Large Video-Language Model
Yang Liu, Pengxiang Ding, Siteng Huang, Min Zhang, Han Zhao, Donglin Wang
2024-09-13
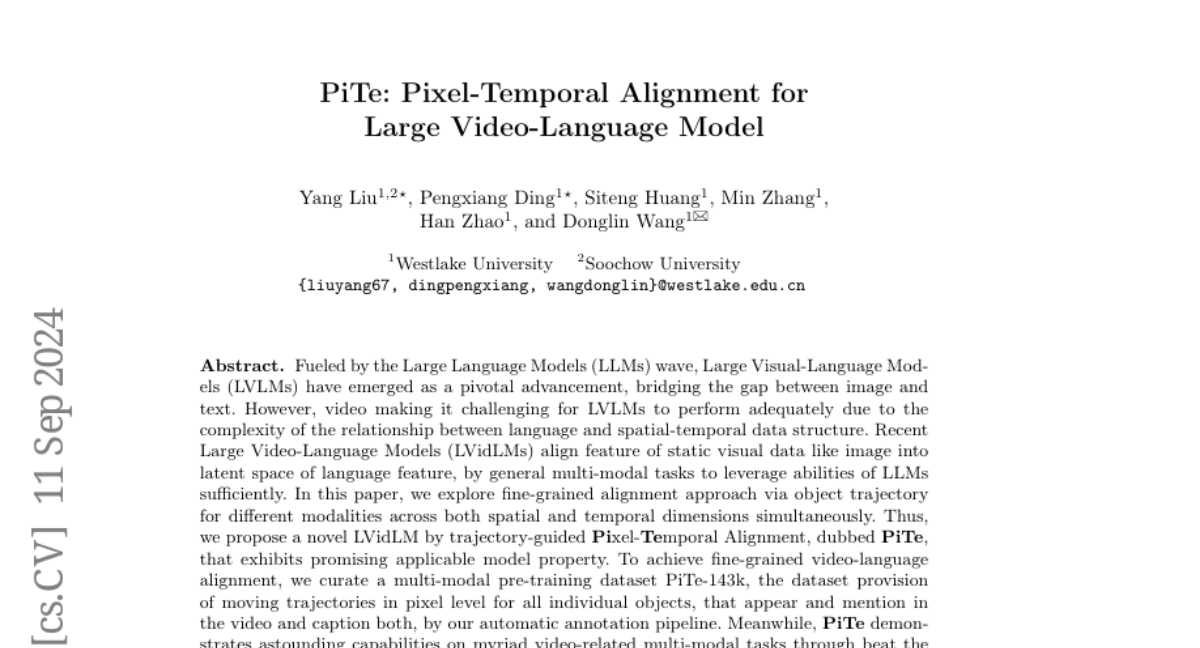
Summary
This paper introduces PiTe, a new method designed to help large video-language models better understand videos by aligning visual data with text descriptions.
What's the problem?
Large video-language models (LVidLMs) struggle to effectively connect the details in videos with the corresponding text because videos contain complex relationships between what is shown and said. This makes it hard for these models to accurately process and analyze video content.
What's the solution?
The authors propose a technique called Pixel-Temporal Alignment, which aligns the movement of objects in videos with their descriptions in text. They created a special dataset called PiTe-143k that includes detailed information about how objects move in videos. This helps the model learn to connect visual elements with language more precisely. Their approach shows improved performance on various tasks related to understanding videos, outperforming previous methods significantly.
Why it matters?
This research is important because it enhances the ability of AI models to analyze and understand video content, which has many real-world applications, such as in video search engines, content creation, and educational tools. By improving how AI interprets video and language together, it opens up new possibilities for technology that can better assist users in accessing and understanding video information.
Abstract
Fueled by the Large Language Models (LLMs) wave, Large Visual-Language Models (LVLMs) have emerged as a pivotal advancement, bridging the gap between image and text. However, video making it challenging for LVLMs to perform adequately due to the complexity of the relationship between language and spatial-temporal data structure. Recent Large Video-Language Models (LVidLMs) align feature of static visual data like image into latent space of language feature, by general multi-modal tasks to leverage abilities of LLMs sufficiently. In this paper, we explore fine-grained alignment approach via object trajectory for different modalities across both spatial and temporal dimensions simultaneously. Thus, we propose a novel LVidLM by trajectory-guided Pixel-Temporal Alignment, dubbed PiTe, that exhibits promising applicable model property. To achieve fine-grained video-language alignment, we curate a multi-modal pre-training dataset PiTe-143k, the dataset provision of moving trajectories in pixel level for all individual objects, that appear and mention in the video and caption both, by our automatic annotation pipeline. Meanwhile, PiTe demonstrates astounding capabilities on myriad video-related multi-modal tasks through beat the state-of-the-art methods by a large margin.