Pixel-Space Post-Training of Latent Diffusion Models
Christina Zhang, Simran Motwani, Matthew Yu, Ji Hou, Felix Juefei-Xu, Sam Tsai, Peter Vajda, Zijian He, Jialiang Wang
2024-09-27
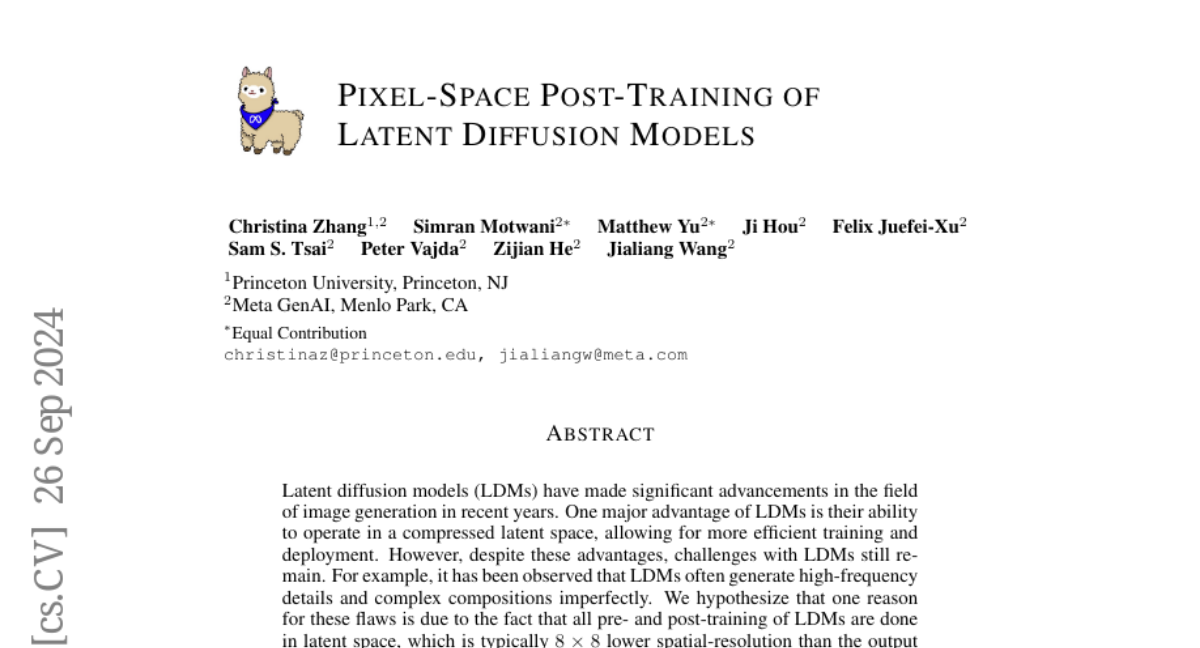
Summary
This paper talks about a new method called Lotus, which improves how diffusion models generate images by focusing on high-quality dense predictions. It aims to enhance the models' ability to create detailed images while being more efficient.
What's the problem?
Diffusion models have become popular for generating images, but they often struggle with producing fine details and complex images. This is partly because these models work in a compressed space that doesn't capture high-resolution information well. Additionally, the traditional approach of predicting noise can lead to inaccuracies in the final images, making it difficult to achieve high-quality results.
What's the solution?
To solve these problems, the researchers introduced Lotus, a model that changes how diffusion processes are handled. Instead of predicting noise, Lotus directly predicts the details needed for image generation, which helps maintain high-frequency details and improves overall image quality. They also simplified the diffusion process into a single-step procedure, which makes it faster and easier to optimize. Furthermore, they developed a tuning strategy called 'detail preserver' that enhances the accuracy of the predictions without needing more training data or larger models.
Why it matters?
This research is important because it significantly enhances the performance of diffusion models in generating detailed and accurate images. By improving the efficiency and quality of image generation, Lotus can be applied in various fields such as computer graphics, virtual reality, and medical imaging, where high-quality visuals are essential.
Abstract
Latent diffusion models (LDMs) have made significant advancements in the field of image generation in recent years. One major advantage of LDMs is their ability to operate in a compressed latent space, allowing for more efficient training and deployment. However, despite these advantages, challenges with LDMs still remain. For example, it has been observed that LDMs often generate high-frequency details and complex compositions imperfectly. We hypothesize that one reason for these flaws is due to the fact that all pre- and post-training of LDMs are done in latent space, which is typically 8 times 8 lower spatial-resolution than the output images. To address this issue, we propose adding pixel-space supervision in the post-training process to better preserve high-frequency details. Experimentally, we show that adding a pixel-space objective significantly improves both supervised quality fine-tuning and preference-based post-training by a large margin on a state-of-the-art DiT transformer and U-Net diffusion models in both visual quality and visual flaw metrics, while maintaining the same text alignment quality.