Policy Filtration in RLHF to Fine-Tune LLM for Code Generation
Wei Shen, Chuheng Zhang
2024-09-17
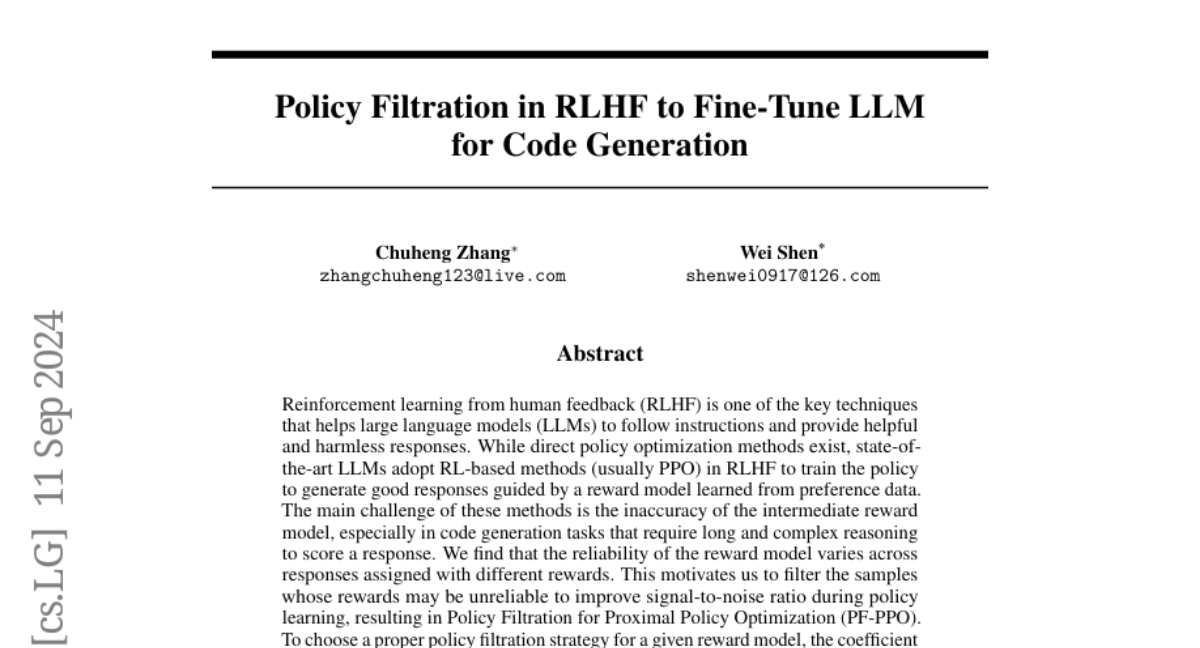
Summary
This paper discusses a method called Policy Filtration for improving how large language models (LLMs) are fine-tuned to generate code using reinforcement learning from human feedback (RLHF).
What's the problem?
Fine-tuning LLMs for tasks like code generation can be difficult because the reward models that guide the learning process are often inaccurate. This inaccuracy is especially problematic in code generation, where the reasoning needed can be complex and lengthy. As a result, the model may not learn effectively, leading to poorer performance.
What's the solution?
The authors propose a new approach called Policy Filtration for Proximal Policy Optimization (PF-PPO). This method filters out samples with unreliable rewards to improve the quality of the feedback the model receives during training. By focusing on more accurate data, PF-PPO helps the model learn better and achieve higher performance in generating code. The paper also provides extensive experiments showing that this method significantly improves results on various coding benchmarks.
Why it matters?
This research is important because it enhances the ability of AI models to generate accurate and functional code, which is crucial for applications in software development and programming education. By improving how these models learn from human feedback, PF-PPO can lead to more reliable AI tools that assist developers and automate coding tasks.
Abstract
Reinforcement learning from human feedback (RLHF) is one of the key techniques that helps large language models (LLMs) to follow instructions and provide helpful and harmless responses. While direct policy optimization methods exist, state-of-the-art LLMs adopt RL-based methods (usually PPO) in RLHF to train the policy to generate good responses guided by a reward model learned from preference data. The main challenge of these methods is the inaccuracy of the intermediate reward model, especially in code generation tasks that require long and complex reasoning to score a response. We find that the reliability of the reward model varies across responses assigned with different rewards. This motivates us to filter the samples whose rewards may be unreliable to improve signal-to-noise ratio during policy learning, resulting in Policy Filtration for Proximal Policy Optimization (PF-PPO). To choose a proper policy filtration strategy for a given reward model, the coefficient of determination (R^2) between rewards and actual scores on filtered samples serves as a good metrics and helps us find several promising strategies. We provide extensive experiments to validate the effectiveness of PF-PPO in code generation tasks, and find that some variants of PF-PPO are highly effective and achieve new state-of-the-art performance across 7-billion-parameter models on HumanEval, MBPP, and a new and more challenging LeetCode Contest benchmark.