Political DEBATE: Efficient Zero-shot and Few-shot Classifiers for Political Text
Michael Burnham, Kayla Kahn, Ryan Yank Wang, Rachel X. Peng
2024-09-05
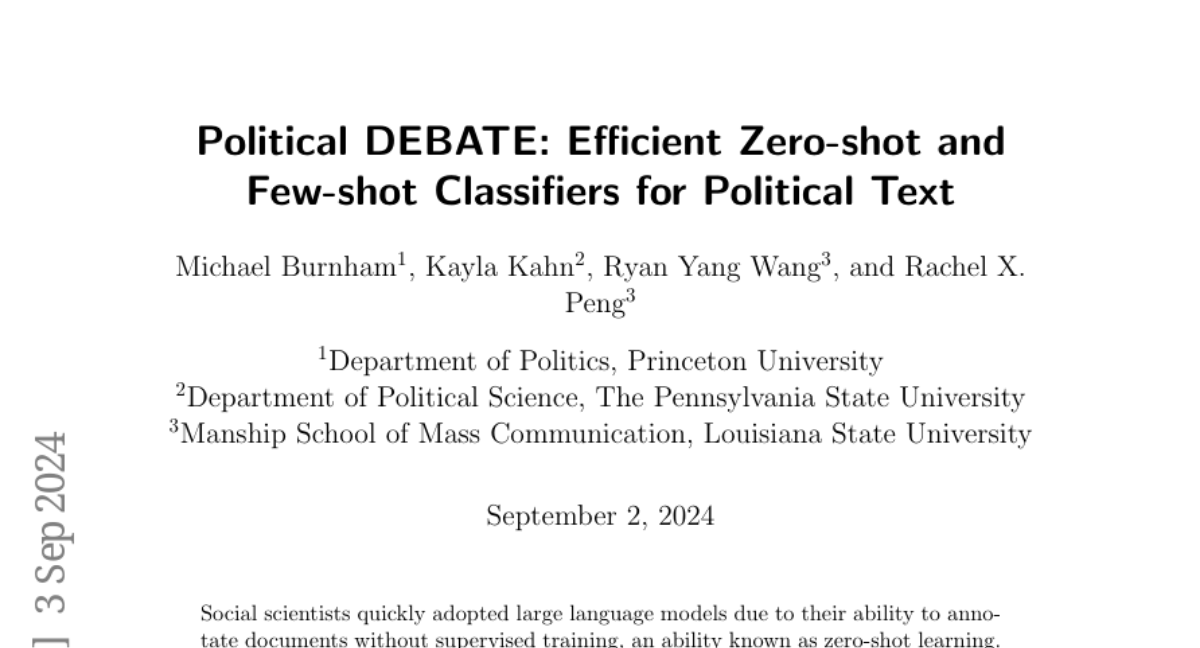
Summary
This paper talks about Political DEBATE, a new set of language models designed to classify political texts efficiently without needing a lot of training data.
What's the problem?
Many researchers want to analyze political documents using large language models, but these models are often expensive and require a lot of data to train. This makes it hard for scientists to replicate studies and use these models in open research.
What's the solution?
The authors introduce Political DEBATE, which uses a small amount of training data (only 10-25 documents) to achieve high performance in classifying political texts. These models are open source, meaning anyone can use them for free, and they outperform other advanced models that require much more data. Additionally, they provide a new dataset called PolNLI, which contains over 200,000 political documents with accurate labels for various classification tasks.
Why it matters?
This research is important because it makes it easier for social scientists and researchers to analyze political texts without needing expensive resources. By providing effective tools that are accessible to everyone, it promotes transparency and collaboration in political research.
Abstract
Social scientists quickly adopted large language models due to their ability to annotate documents without supervised training, an ability known as zero-shot learning. However, due to their compute demands, cost, and often proprietary nature, these models are often at odds with replication and open science standards. This paper introduces the Political DEBATE (DeBERTa Algorithm for Textual Entailment) language models for zero-shot and few-shot classification of political documents. These models are not only as good, or better than, state-of-the art large language models at zero and few-shot classification, but are orders of magnitude more efficient and completely open source. By training the models on a simple random sample of 10-25 documents, they can outperform supervised classifiers trained on hundreds or thousands of documents and state-of-the-art generative models with complex, engineered prompts. Additionally, we release the PolNLI dataset used to train these models -- a corpus of over 200,000 political documents with highly accurate labels across over 800 classification tasks.