Portrait Video Editing Empowered by Multimodal Generative Priors
Xuan Gao, Haiyao Xiao, Chenglai Zhong, Shimin Hu, Yudong Guo, Juyong Zhang
2024-09-23
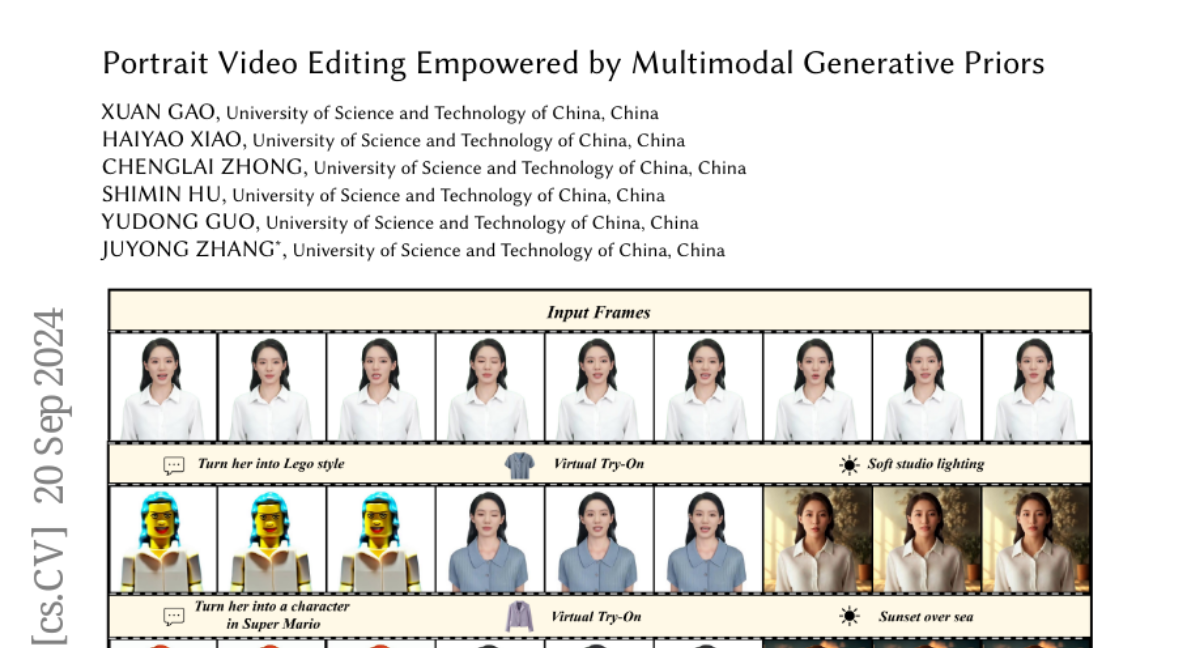
Summary
This paper presents PortraitGen, an advanced method for editing portrait videos that allows for consistent and creative changes using various input types. It addresses common challenges in video editing, like maintaining quality and coherence across frames.
What's the problem?
Traditional methods for editing portrait videos often struggle with keeping the images consistent in 3D space and across time, leading to issues like poor rendering quality and slow processing speeds. These problems make it difficult to create visually appealing edits that look natural and smooth throughout the video.
What's the solution?
To solve these issues, the researchers developed PortraitGen, which transforms 2D portrait videos into a dynamic 3D Gaussian field. This approach ensures that the structure and motion of the video remain consistent. They also introduced a new technique called Neural Gaussian Texture, which allows for fast rendering speeds of over 100 frames per second while enabling complex style edits. The system can take different types of inputs, like text or images, to guide the editing process, making it versatile for various applications such as changing styles or lighting in videos.
Why it matters?
This research is important because it significantly improves how portrait videos can be edited, making it easier for creators to produce high-quality content quickly. By enhancing the editing process with advanced technology, PortraitGen opens up new possibilities for filmmakers, content creators, and artists to express their creativity in more dynamic ways.
Abstract
We introduce PortraitGen, a powerful portrait video editing method that achieves consistent and expressive stylization with multimodal prompts. Traditional portrait video editing methods often struggle with 3D and temporal consistency, and typically lack in rendering quality and efficiency. To address these issues, we lift the portrait video frames to a unified dynamic 3D Gaussian field, which ensures structural and temporal coherence across frames. Furthermore, we design a novel Neural Gaussian Texture mechanism that not only enables sophisticated style editing but also achieves rendering speed over 100FPS. Our approach incorporates multimodal inputs through knowledge distilled from large-scale 2D generative models. Our system also incorporates expression similarity guidance and a face-aware portrait editing module, effectively mitigating degradation issues associated with iterative dataset updates. Extensive experiments demonstrate the temporal consistency, editing efficiency, and superior rendering quality of our method. The broad applicability of the proposed approach is demonstrated through various applications, including text-driven editing, image-driven editing, and relighting, highlighting its great potential to advance the field of video editing. Demo videos and released code are provided in our project page: https://ustc3dv.github.io/PortraitGen/