Pre-training Distillation for Large Language Models: A Design Space Exploration
Hao Peng, Xin Lv, Yushi Bai, Zijun Yao, Jiajie Zhang, Lei Hou, Juanzi Li
2024-10-22
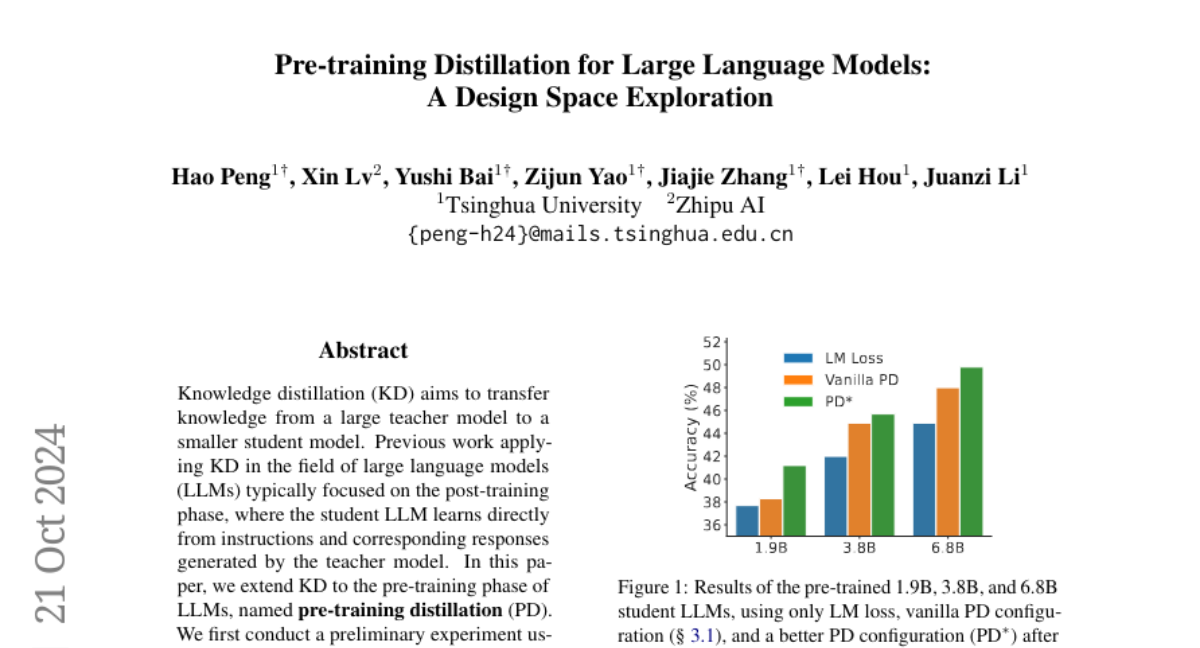
Summary
This paper discusses pre-training distillation (PD), a new approach that helps smaller language models learn from larger ones before they are fully trained, making them more efficient and effective.
What's the problem?
When training large language models (LLMs), it's common to use a process called knowledge distillation (KD) to transfer knowledge from a big 'teacher' model to a smaller 'student' model. However, most methods only apply this technique after the models are fully trained, which can limit the effectiveness of the smaller model, especially when there isn't enough data available for training.
What's the solution?
The authors propose pre-training distillation (PD), which allows the student model to learn from the teacher model during the initial training phase. They conducted experiments using a large teacher model called GLM-4-9B to train a smaller student model with 1.9 billion parameters. The paper explores several important factors that affect how well PD works, including how to process the teacher's outputs, which loss functions to use, and whether to use data from the teacher's training process in real-time or afterward. Their findings show that larger student models benefit more from this method, while simply having a larger teacher model doesn’t always guarantee better results.
Why it matters?
This research is significant because it provides a new way to enhance the training of smaller language models, making them more capable and efficient. By introducing pre-training distillation, the authors aim to improve how we train AI systems, potentially leading to better performance in various applications where language understanding is crucial.
Abstract
Knowledge distillation (KD) aims to transfer knowledge from a large teacher model to a smaller student model. Previous work applying KD in the field of large language models (LLMs) typically focused on the post-training phase, where the student LLM learns directly from instructions and corresponding responses generated by the teacher model. In this paper, we extend KD to the pre-training phase of LLMs, named pre-training distillation (PD). We first conduct a preliminary experiment using GLM-4-9B as the teacher LLM to distill a 1.9B parameter student LLM, validating the effectiveness of PD. Considering the key impact factors of distillation, we systematically explore the design space of pre-training distillation across four aspects: logits processing, loss selection, scaling law, and offline or online logits. We conduct extensive experiments to explore the design space of pre-training distillation and find better configurations and interesting conclusions, such as larger student LLMs generally benefiting more from pre-training distillation, while a larger teacher LLM does not necessarily guarantee better results. We hope our exploration of the design space will inform future practices in pre-training distillation.