Predicting the Original Appearance of Damaged Historical Documents
Zhenhua Yang, Dezhi Peng, Yongxin Shi, Yuyi Zhang, Chongyu Liu, Lianwen Jin
2024-12-19
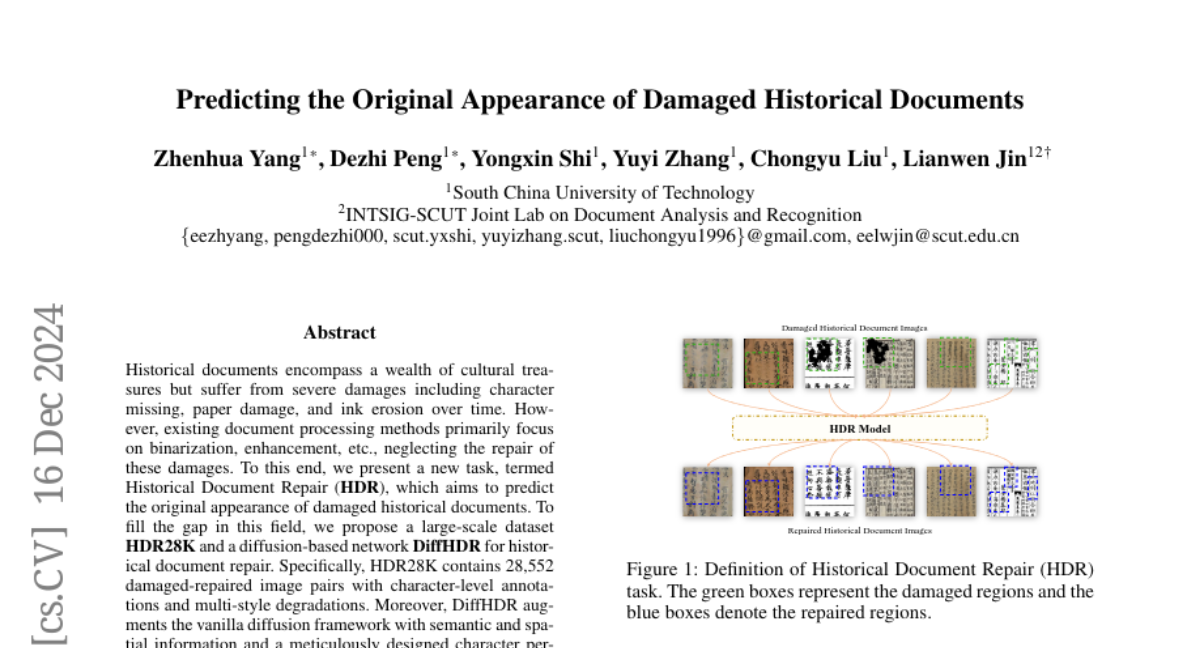
Summary
This paper discusses a new method for restoring damaged historical documents by predicting what they originally looked like. It introduces a task called Historical Document Repair (HDR) and presents a dataset and a specialized network to achieve this.
What's the problem?
Historical documents often suffer from various types of damage, such as missing text, paper tears, and faded ink, making it hard to read and understand them. Current methods mainly focus on enhancing the appearance of these documents but do not effectively restore their original look.
What's the solution?
To tackle this issue, the authors created a large dataset called HDR28K, which includes over 28,000 pairs of damaged and repaired images. They developed a network named DiffHDR that uses advanced techniques to predict the original appearance of these documents. This network improves upon existing methods by incorporating semantic information and specific loss functions to ensure the restored documents look coherent and accurate.
Why it matters?
This research is important because it helps preserve cultural heritage by making damaged historical documents readable again. By accurately restoring these documents, we can better understand our history and maintain valuable cultural artifacts for future generations.
Abstract
Historical documents encompass a wealth of cultural treasures but suffer from severe damages including character missing, paper damage, and ink erosion over time. However, existing document processing methods primarily focus on binarization, enhancement, etc., neglecting the repair of these damages. To this end, we present a new task, termed Historical Document Repair (HDR), which aims to predict the original appearance of damaged historical documents. To fill the gap in this field, we propose a large-scale dataset HDR28K and a diffusion-based network DiffHDR for historical document repair. Specifically, HDR28K contains 28,552 damaged-repaired image pairs with character-level annotations and multi-style degradations. Moreover, DiffHDR augments the vanilla diffusion framework with semantic and spatial information and a meticulously designed character perceptual loss for contextual and visual coherence. Experimental results demonstrate that the proposed DiffHDR trained using HDR28K significantly surpasses existing approaches and exhibits remarkable performance in handling real damaged documents. Notably, DiffHDR can also be extended to document editing and text block generation, showcasing its high flexibility and generalization capacity. We believe this study could pioneer a new direction of document processing and contribute to the inheritance of invaluable cultures and civilizations. The dataset and code is available at https://github.com/yeungchenwa/HDR.