Preference Tuning with Human Feedback on Language, Speech, and Vision Tasks: A Survey
Genta Indra Winata, Hanyang Zhao, Anirban Das, Wenpin Tang, David D. Yao, Shi-Xiong Zhang, Sambit Sahu
2024-09-19
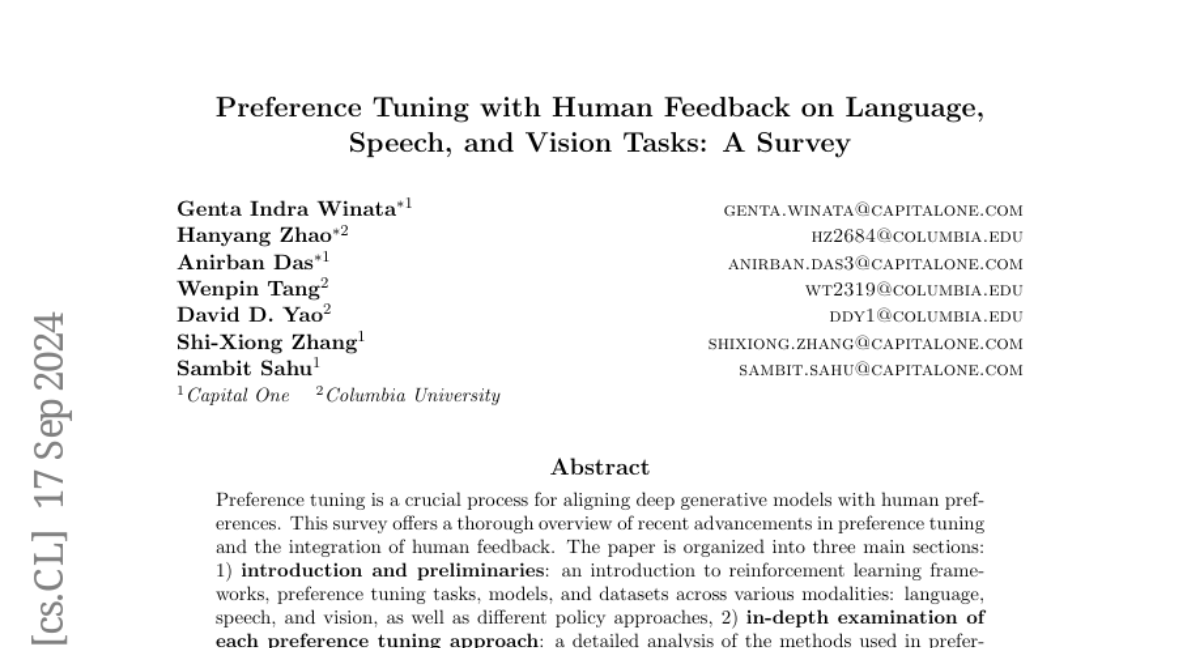
Summary
This paper surveys recent advancements in preference tuning, which is a method used to align language models with human preferences by incorporating human feedback across various tasks like language, speech, and vision.
What's the problem?
As language models become more advanced, it's important to ensure they generate outputs that reflect what users actually want. However, aligning these models with human preferences can be challenging because traditional methods may not effectively capture the nuances of user feedback or may require extensive resources to personalize each model for individual users.
What's the solution?
The paper is organized into three main sections: it starts with an introduction to reinforcement learning frameworks and preference tuning tasks; then it provides a detailed analysis of different methods used for preference tuning; and finally, it explores applications and future directions for this research. By reviewing over 100 papers and evaluating various models and datasets, the authors aim to present the latest techniques in preference tuning, enhancing understanding in this field.
Why it matters?
This research is significant because it helps improve how AI models interact with users by making their responses more aligned with human values and preferences. This can lead to better user experiences in applications such as virtual assistants, customer service bots, and other AI-driven technologies that rely on understanding human input.
Abstract
Preference tuning is a crucial process for aligning deep generative models with human preferences. This survey offers a thorough overview of recent advancements in preference tuning and the integration of human feedback. The paper is organized into three main sections: 1) introduction and preliminaries: an introduction to reinforcement learning frameworks, preference tuning tasks, models, and datasets across various modalities: language, speech, and vision, as well as different policy approaches, 2) in-depth examination of each preference tuning approach: a detailed analysis of the methods used in preference tuning, and 3) applications, discussion, and future directions: an exploration of the applications of preference tuning in downstream tasks, including evaluation methods for different modalities, and an outlook on future research directions. Our objective is to present the latest methodologies in preference tuning and model alignment, enhancing the understanding of this field for researchers and practitioners. We hope to encourage further engagement and innovation in this area.