Programming Every Example: Lifting Pre-training Data Quality like Experts at Scale
Fan Zhou, Zengzhi Wang, Qian Liu, Junlong Li, Pengfei Liu
2024-09-26
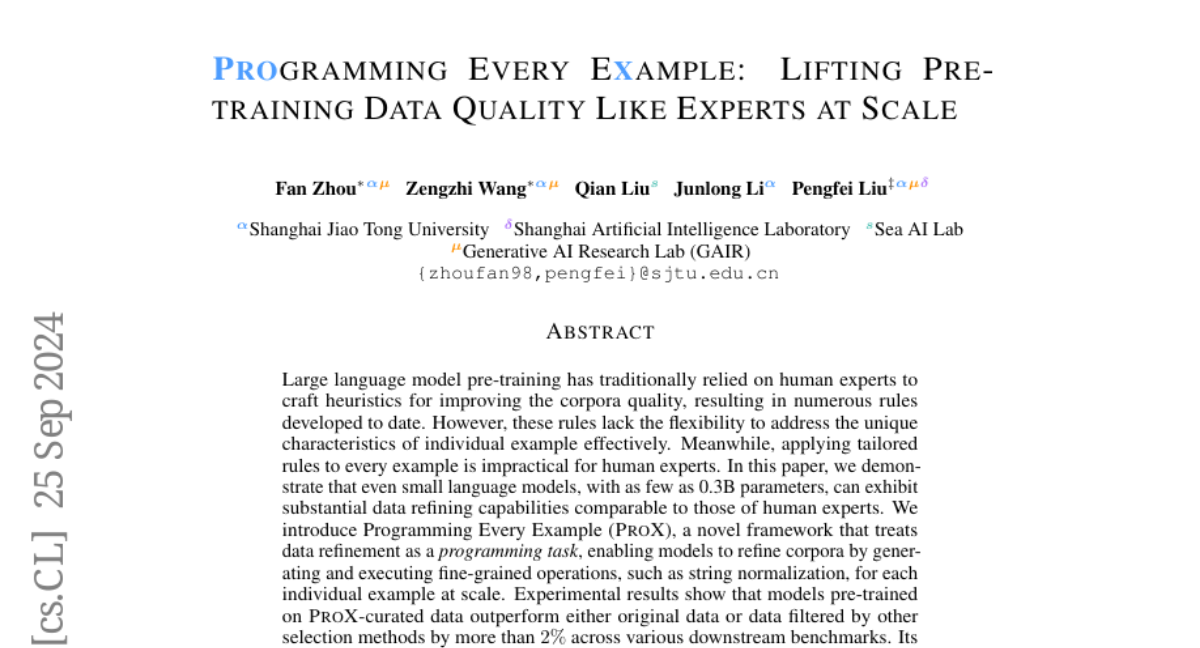
Summary
This paper discusses a new method called Programming Every Example (ProX) that improves the quality of data used to train large language models (LLMs). It treats data refinement like a programming task, allowing models to clean and enhance data more effectively and efficiently.
What's the problem?
Traditional methods for improving the quality of training data rely on human experts who create rules to refine the data. However, these rules can be inflexible and may not work well with every individual example. Additionally, it's impractical for human experts to apply tailored rules to every piece of data, which limits the effectiveness of the training process.
What's the solution?
To solve this problem, the researchers developed ProX, a framework that enables even small language models (with as few as 0.3 billion parameters) to refine data by generating and executing specific operations for each example. This approach allows the model to automatically clean and improve the data without needing extensive human intervention. The results showed that models trained on ProX-refined data performed better than those trained on raw or rule-based data, achieving over 2% improvement in various benchmarks.
Why it matters?
This research is important because it provides a more efficient way to enhance the quality of training data for language models, which is crucial for their performance. By allowing smaller models to effectively refine large datasets, ProX can save resources and time while still producing high-quality results. This advancement could lead to better AI systems that are more capable of understanding and generating human-like text.
Abstract
Large language model pre-training has traditionally relied on human experts to craft heuristics for improving the corpora quality, resulting in numerous rules developed to date. However, these rules lack the flexibility to address the unique characteristics of individual example effectively. Meanwhile, applying tailored rules to every example is impractical for human experts. In this paper, we demonstrate that even small language models, with as few as 0.3B parameters, can exhibit substantial data refining capabilities comparable to those of human experts. We introduce Programming Every Example (ProX), a novel framework that treats data refinement as a programming task, enabling models to refine corpora by generating and executing fine-grained operations, such as string normalization, for each individual example at scale. Experimental results show that models pre-trained on ProX-curated data outperform either original data or data filtered by other selection methods by more than 2% across various downstream benchmarks. Its effectiveness spans various model sizes and pre-training corpora, including C4, RedPajama-V2, and FineWeb. Furthermore, ProX exhibits significant potential in domain-specific continual pre-training: without domain specific design, models trained on OpenWebMath refined by ProX outperform human-crafted rule-based methods, improving average accuracy by 7.6% over Mistral-7B, with 14.6% for Llama-2-7B and 20.3% for CodeLlama-7B, all within 10B tokens to be comparable to models like Llemma-7B trained on 200B tokens. Further analysis highlights that ProX significantly saves training FLOPs, offering a promising path for efficient LLM pre-training.We are open-sourcing ProX with >100B corpus, models, and sharing all training and implementation details for reproducible research and future innovation. Code: https://github.com/GAIR-NLP/ProX