Prompting Depth Anything for 4K Resolution Accurate Metric Depth Estimation
Haotong Lin, Sida Peng, Jingxiao Chen, Songyou Peng, Jiaming Sun, Minghuan Liu, Hujun Bao, Jiashi Feng, Xiaowei Zhou, Bingyi Kang
2024-12-19
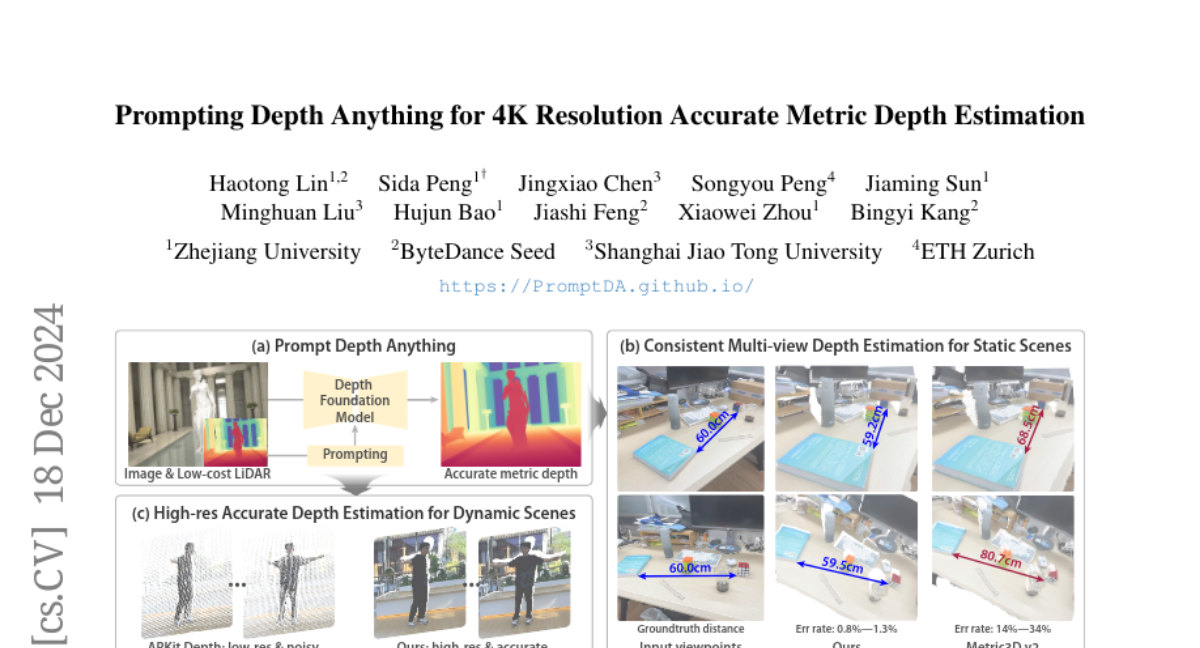
Summary
This paper talks about Prompt Depth Anything, a new method for accurately estimating depth in images using a low-cost LiDAR device as a guide, achieving high-resolution outputs up to 4K.
What's the problem?
Accurately determining how far away objects are in images (depth estimation) is challenging, especially when using existing methods that may not provide precise results. Many depth estimation models struggle with limited data and often produce inaccurate depth information, which can lead to poor performance in applications like 3D reconstruction and robotics.
What's the solution?
The authors introduce Prompt Depth Anything, which uses a low-cost LiDAR sensor to provide additional information for depth estimation. This method integrates the LiDAR data at different levels within the model to improve accuracy. They also developed a scalable data pipeline to create synthetic data for training and generate high-quality depth information from real-world data. This combination allows the model to achieve better depth estimation results on popular datasets compared to previous methods.
Why it matters?
This research is important because it enhances the accuracy of depth estimation, which is crucial for various applications such as virtual reality, robotics, and 3D modeling. By improving how AI models understand distance in images, Prompt Depth Anything can lead to more effective technologies in fields like autonomous driving and augmented reality.
Abstract
Prompts play a critical role in unleashing the power of language and vision foundation models for specific tasks. For the first time, we introduce prompting into depth foundation models, creating a new paradigm for metric depth estimation termed Prompt Depth Anything. Specifically, we use a low-cost LiDAR as the prompt to guide the Depth Anything model for accurate metric depth output, achieving up to 4K resolution. Our approach centers on a concise prompt fusion design that integrates the LiDAR at multiple scales within the depth decoder. To address training challenges posed by limited datasets containing both LiDAR depth and precise GT depth, we propose a scalable data pipeline that includes synthetic data LiDAR simulation and real data pseudo GT depth generation. Our approach sets new state-of-the-arts on the ARKitScenes and ScanNet++ datasets and benefits downstream applications, including 3D reconstruction and generalized robotic grasping.