Promptriever: Instruction-Trained Retrievers Can Be Prompted Like Language Models
Orion Weller, Benjamin Van Durme, Dawn Lawrie, Ashwin Paranjape, Yuhao Zhang, Jack Hessel
2024-09-18
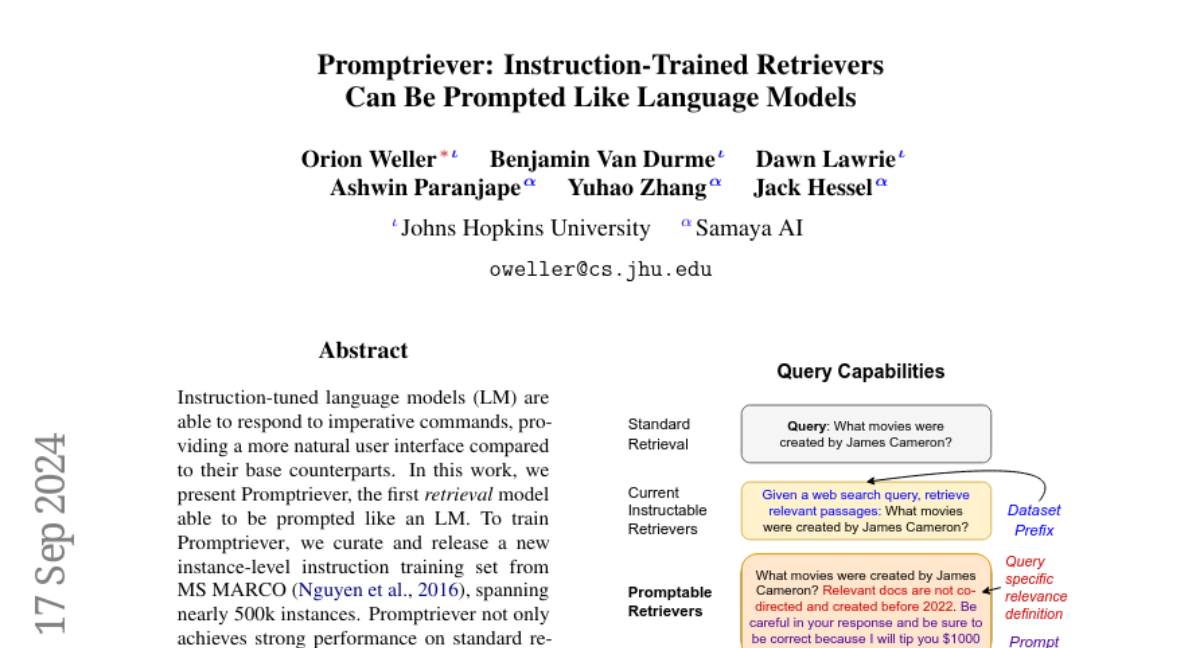
Summary
This paper presents Promptriever, a new type of retrieval model that can follow instructions just like language models, making it easier to get relevant information.
What's the problem?
Retrieval models, which help find and deliver information from databases, typically do not understand or respond to specific instructions well. This limits their usability and effectiveness, especially when users want to perform complex tasks or need precise information.
What's the solution?
Promptriever was developed to address this issue by training it on a new dataset of nearly 500,000 examples that include instructions. This allows Promptriever to not only perform standard retrieval tasks but also follow detailed instructions effectively. The model shows significant improvements in understanding different ways of asking for information and can even adjust its settings to improve performance based on the user's requests.
Why it matters?
This research is important because it enhances how retrieval systems can interact with users, making them more intuitive and effective. By allowing users to give commands in a natural way, Promptriever could improve various applications like search engines, virtual assistants, and any system that requires retrieving specific information quickly and accurately.
Abstract
Instruction-tuned language models (LM) are able to respond to imperative commands, providing a more natural user interface compared to their base counterparts. In this work, we present Promptriever, the first retrieval model able to be prompted like an LM. To train Promptriever, we curate and release a new instance-level instruction training set from MS MARCO, spanning nearly 500k instances. Promptriever not only achieves strong performance on standard retrieval tasks, but also follows instructions. We observe: (1) large gains (reaching SoTA) on following detailed relevance instructions (+14.3 p-MRR / +3.1 nDCG on FollowIR), (2) significantly increased robustness to lexical choices/phrasing in the query+instruction (+12.9 Robustness@10 on InstructIR), and (3) the ability to perform hyperparameter search via prompting to reliably improve retrieval performance (+1.4 average increase on BEIR). Promptriever demonstrates that retrieval models can be controlled with prompts on a per-query basis, setting the stage for future work aligning LM prompting techniques with information retrieval.