ProSA: Assessing and Understanding the Prompt Sensitivity of LLMs
Jingming Zhuo, Songyang Zhang, Xinyu Fang, Haodong Duan, Dahua Lin, Kai Chen
2024-10-17
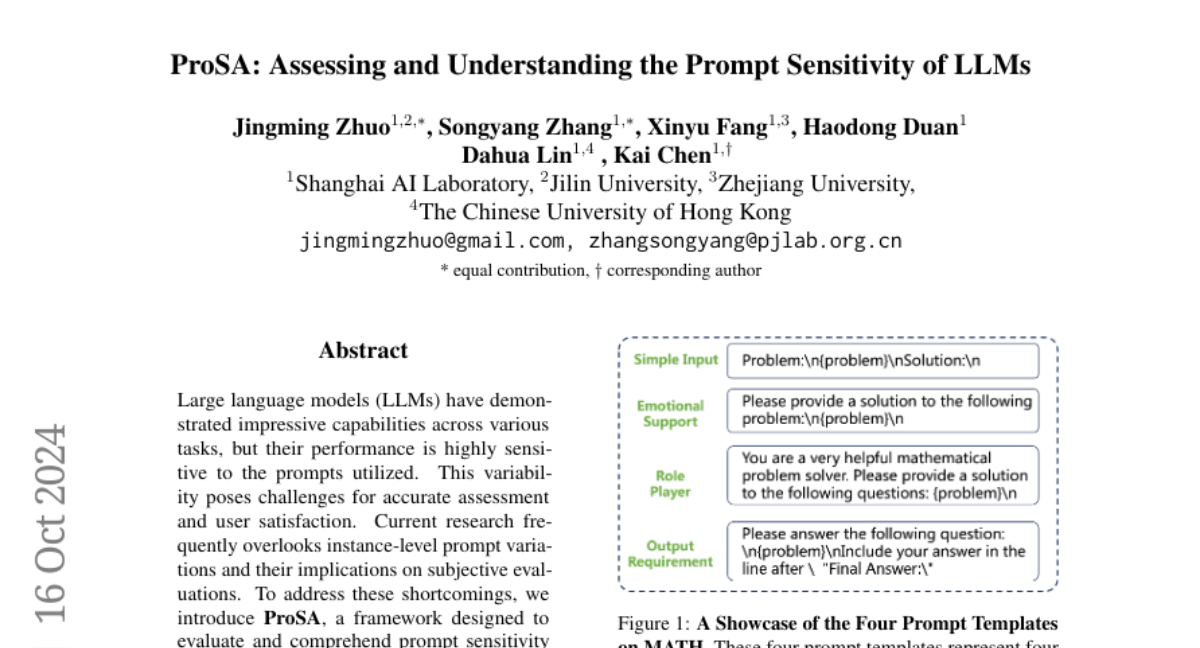
Summary
This paper introduces ProSA, a framework designed to evaluate how sensitive large language models (LLMs) are to changes in prompts, which are the instructions given to the models.
What's the problem?
Large language models can perform well on various tasks, but their results can change significantly based on the specific prompts used. This sensitivity makes it hard to accurately assess their performance and can lead to user dissatisfaction. Most research has not focused on how small changes in prompts affect the model's output at a detailed level.
What's the solution?
To address this issue, the authors developed ProSA, which includes a new metric called PromptSensiScore (PSS) that measures how sensitive LLMs are to different prompts for the same task. They conducted extensive tests across various tasks and found that larger models tend to be more robust against prompt changes. They also discovered that providing a few example prompts can help reduce this sensitivity. Additionally, they noted that subjective evaluations of model performance are also affected by prompt sensitivity, especially in complex tasks.
Why it matters?
This research is important because it helps improve our understanding of how LLMs respond to different prompts. By identifying and measuring prompt sensitivity, we can enhance the evaluation and selection of these models, leading to better user experiences and more reliable AI applications.
Abstract
Large language models (LLMs) have demonstrated impressive capabilities across various tasks, but their performance is highly sensitive to the prompts utilized. This variability poses challenges for accurate assessment and user satisfaction. Current research frequently overlooks instance-level prompt variations and their implications on subjective evaluations. To address these shortcomings, we introduce ProSA, a framework designed to evaluate and comprehend prompt sensitivity in LLMs. ProSA incorporates a novel sensitivity metric, PromptSensiScore, and leverages decoding confidence to elucidate underlying mechanisms. Our extensive study, spanning multiple tasks, uncovers that prompt sensitivity fluctuates across datasets and models, with larger models exhibiting enhanced robustness. We observe that few-shot examples can alleviate this sensitivity issue, and subjective evaluations are also susceptible to prompt sensitivities, particularly in complex, reasoning-oriented tasks. Furthermore, our findings indicate that higher model confidence correlates with increased prompt robustness. We believe this work will serve as a helpful tool in studying prompt sensitivity of LLMs. The project is released at: https://github.com/open-compass/ProSA .