Putting Data at the Centre of Offline Multi-Agent Reinforcement Learning
Claude Formanek, Louise Beyers, Callum Rhys Tilbury, Jonathan P. Shock, Arnu Pretorius
2024-09-19
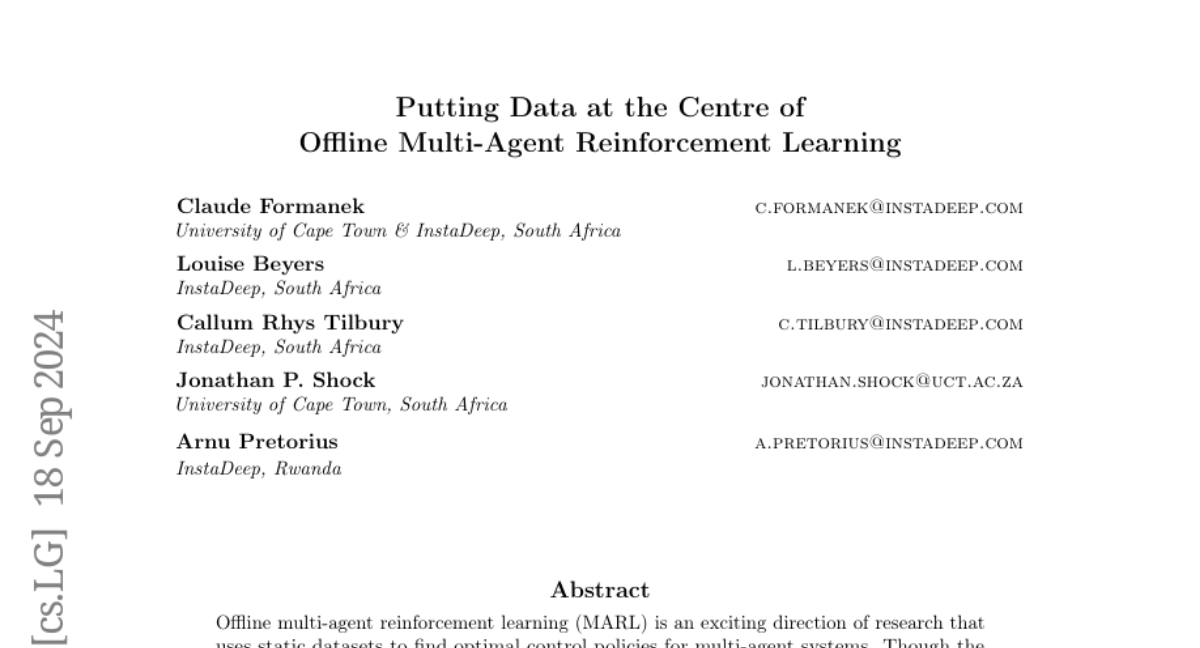
Summary
This paper discusses the importance of using data effectively in offline multi-agent reinforcement learning (MARL) and introduces new guidelines and resources to improve how researchers handle datasets in this field.
What's the problem?
In offline MARL, where multiple agents learn from pre-collected data, many researchers create their own datasets without a consistent method. This lack of standardization can lead to confusion and poor performance because the quality and characteristics of the data are not well understood. Without a clear focus on the data, it becomes difficult to compare results across different studies.
What's the solution?
The authors propose several key contributions to improve data usage in offline MARL: (1) they provide clear guidelines for generating new datasets; (2) they standardize over 80 existing datasets into a public repository with a consistent format and easy access; and (3) they develop tools for analyzing these datasets to better understand their properties. These efforts aim to create a solid foundation for future experiments in the field.
Why it matters?
This research is important because it emphasizes the need for high-quality, well-understood datasets in offline MARL. By improving how researchers handle data, it can lead to better training of multi-agent systems, which are crucial for applications like robotics, gaming, and autonomous vehicles. Creating standardized resources also helps foster collaboration and innovation within the research community.
Abstract
Offline multi-agent reinforcement learning (MARL) is an exciting direction of research that uses static datasets to find optimal control policies for multi-agent systems. Though the field is by definition data-driven, efforts have thus far neglected data in their drive to achieve state-of-the-art results. We first substantiate this claim by surveying the literature, showing how the majority of works generate their own datasets without consistent methodology and provide sparse information about the characteristics of these datasets. We then show why neglecting the nature of the data is problematic, through salient examples of how tightly algorithmic performance is coupled to the dataset used, necessitating a common foundation for experiments in the field. In response, we take a big step towards improving data usage and data awareness in offline MARL, with three key contributions: (1) a clear guideline for generating novel datasets; (2) a standardisation of over 80 existing datasets, hosted in a publicly available repository, using a consistent storage format and easy-to-use API; and (3) a suite of analysis tools that allow us to understand these datasets better, aiding further development.