RACER: Rich Language-Guided Failure Recovery Policies for Imitation Learning
Yinpei Dai, Jayjun Lee, Nima Fazeli, Joyce Chai
2024-09-24
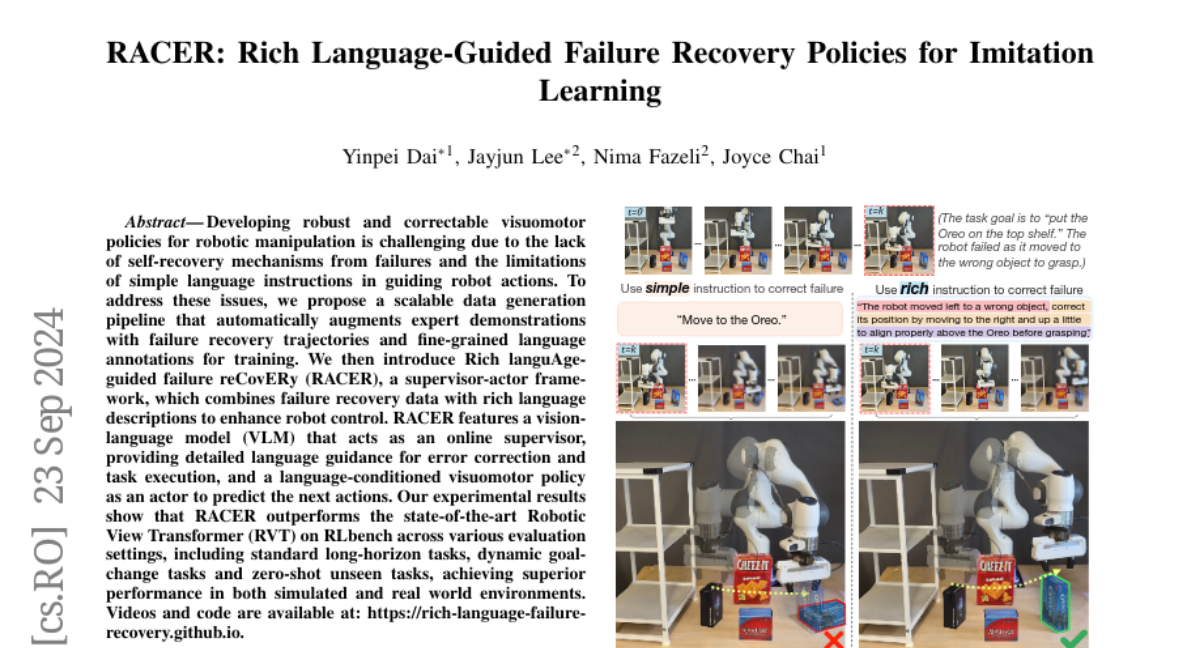
Summary
This paper presents RACER, a new method that helps robots learn how to recover from mistakes using rich language instructions. It focuses on improving how robots perform tasks by combining visual information and detailed language guidance.
What's the problem?
Robots often struggle to recover from errors during tasks because they lack effective self-recovery mechanisms. Additionally, simple language instructions may not provide enough detail for the robot to understand how to correct its actions, leading to poor performance in complex situations.
What's the solution?
To solve these issues, the researchers developed a scalable data generation pipeline that enhances expert demonstrations with examples of failure recovery and detailed language annotations. They introduced RACER, which combines failure recovery data with rich language descriptions. This framework includes a vision-language model that acts as an online supervisor, giving the robot specific guidance on how to fix errors and complete tasks. The model has been tested and shown to outperform existing methods in various challenging scenarios.
Why it matters?
This research is important because it improves the ability of robots to learn from their mistakes and perform tasks more effectively. By integrating detailed language instructions with visual information, RACER helps robots become more capable and reliable in real-world applications, such as manufacturing, healthcare, and service industries.
Abstract
Developing robust and correctable visuomotor policies for robotic manipulation is challenging due to the lack of self-recovery mechanisms from failures and the limitations of simple language instructions in guiding robot actions. To address these issues, we propose a scalable data generation pipeline that automatically augments expert demonstrations with failure recovery trajectories and fine-grained language annotations for training. We then introduce Rich languAge-guided failure reCovERy (RACER), a supervisor-actor framework, which combines failure recovery data with rich language descriptions to enhance robot control. RACER features a vision-language model (VLM) that acts as an online supervisor, providing detailed language guidance for error correction and task execution, and a language-conditioned visuomotor policy as an actor to predict the next actions. Our experimental results show that RACER outperforms the state-of-the-art Robotic View Transformer (RVT) on RLbench across various evaluation settings, including standard long-horizon tasks, dynamic goal-change tasks and zero-shot unseen tasks, achieving superior performance in both simulated and real world environments. Videos and code are available at: https://rich-language-failure-recovery.github.io.