Randomized Autoregressive Visual Generation
Qihang Yu, Ju He, Xueqing Deng, Xiaohui Shen, Liang-Chieh Chen
2024-11-04
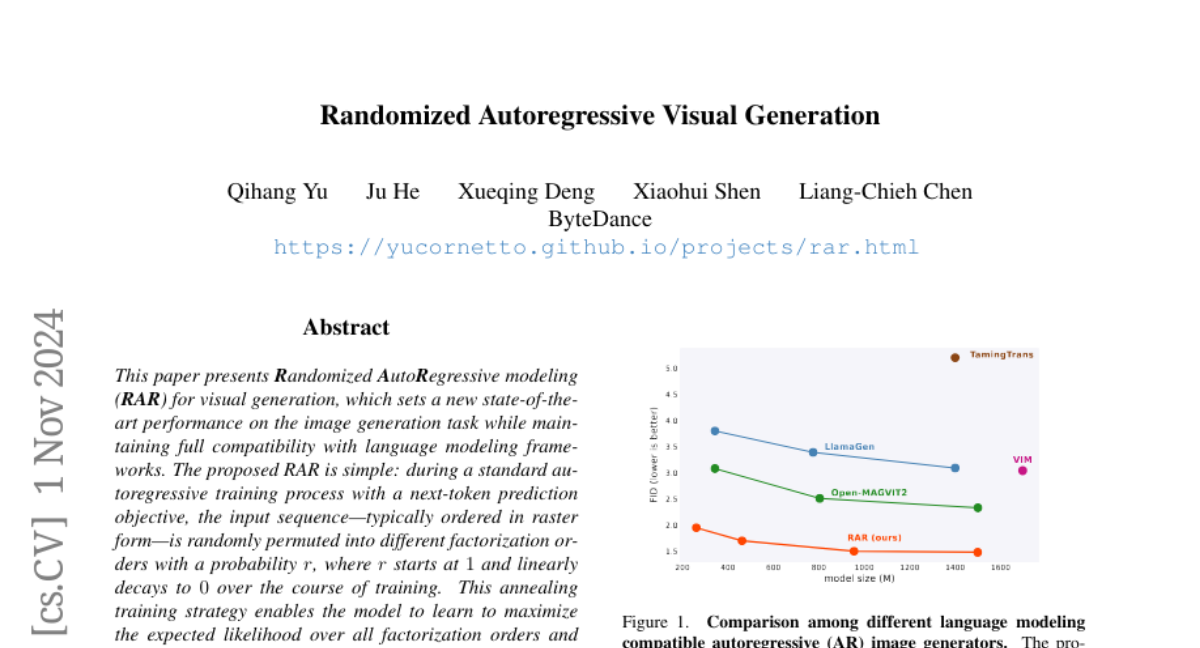
Summary
This paper introduces a new method called Randomized AutoRegressive modeling (RAR) for generating images. It improves how images are created by allowing the model to learn from different arrangements of image data, leading to better and more diverse image generation.
What's the problem?
Traditional methods for generating images often use a fixed order to process the data, which can limit the model's ability to understand complex visual patterns. This can result in images that are too similar or lack detail because the model isn't learning from all possible relationships in the data.
What's the solution?
RAR addresses this issue by introducing randomness into the training process. During training, the order of the input data is randomly changed, which helps the model learn from various arrangements. Over time, this randomness decreases, allowing the model to focus more on a standard order while still benefiting from its earlier diverse learning. This approach allows RAR to capture more complex relationships in the data and significantly improves image quality.
Why it matters?
This research is important because it sets a new standard for image generation techniques. By enhancing how models learn from visual data, RAR can produce higher-quality and more varied images, which has applications in fields like computer graphics, video games, and artificial intelligence. This advancement could lead to more realistic and creative visual content.
Abstract
This paper presents Randomized AutoRegressive modeling (RAR) for visual generation, which sets a new state-of-the-art performance on the image generation task while maintaining full compatibility with language modeling frameworks. The proposed RAR is simple: during a standard autoregressive training process with a next-token prediction objective, the input sequence-typically ordered in raster form-is randomly permuted into different factorization orders with a probability r, where r starts at 1 and linearly decays to 0 over the course of training. This annealing training strategy enables the model to learn to maximize the expected likelihood over all factorization orders and thus effectively improve the model's capability of modeling bidirectional contexts. Importantly, RAR preserves the integrity of the autoregressive modeling framework, ensuring full compatibility with language modeling while significantly improving performance in image generation. On the ImageNet-256 benchmark, RAR achieves an FID score of 1.48, not only surpassing prior state-of-the-art autoregressive image generators but also outperforming leading diffusion-based and masked transformer-based methods. Code and models will be made available at https://github.com/bytedance/1d-tokenizer