RaTEScore: A Metric for Radiology Report Generation
Weike Zhao, Chaoyi Wu, Xiaoman Zhang, Ya Zhang, Yanfeng Wang, Weidi Xie
2024-07-01
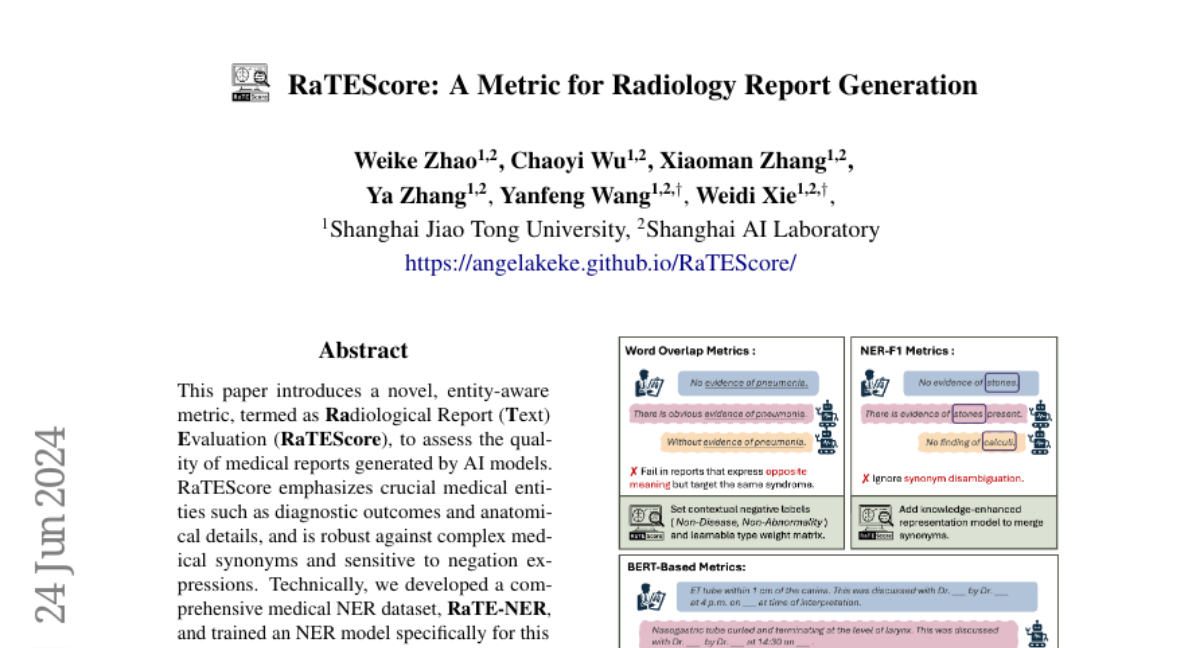
Summary
This paper talks about RaTEScore, a new way to evaluate the quality of medical reports created by AI models. It focuses on important medical details and how well the AI understands and represents these details in its reports.
What's the problem?
As AI becomes more involved in generating medical reports, it's essential to have a reliable way to measure how good these reports are. Existing evaluation methods often fail to capture the nuances of medical language and might not accurately reflect what healthcare professionals consider important in a report. This can lead to AI-generated reports that don't meet the needs of doctors or patients.
What's the solution?
To solve this problem, the authors developed RaTEScore, which is an entity-aware metric designed specifically for radiology reports. This metric focuses on key medical entities like diagnoses and anatomical parts, ensuring that it accurately assesses the quality of the generated text. The authors also created a dataset called RaTE-NER to train a model that breaks down complex medical reports into their essential components. By comparing how similar these components are to what experts would expect, RaTEScore provides a more accurate evaluation of AI-generated reports.
Why it matters?
This research is important because it helps improve the quality of AI-generated medical reports, making them more useful for healthcare professionals. By ensuring that these reports accurately reflect critical medical information, RaTEScore can enhance patient care and support better decision-making in clinical settings. This advancement is crucial as AI continues to play a larger role in healthcare.
Abstract
This paper introduces a novel, entity-aware metric, termed as Radiological Report (Text) Evaluation (RaTEScore), to assess the quality of medical reports generated by AI models. RaTEScore emphasizes crucial medical entities such as diagnostic outcomes and anatomical details, and is robust against complex medical synonyms and sensitive to negation expressions. Technically, we developed a comprehensive medical NER dataset, RaTE-NER, and trained an NER model specifically for this purpose. This model enables the decomposition of complex radiological reports into constituent medical entities. The metric itself is derived by comparing the similarity of entity embeddings, obtained from a language model, based on their types and relevance to clinical significance. Our evaluations demonstrate that RaTEScore aligns more closely with human preference than existing metrics, validated both on established public benchmarks and our newly proposed RaTE-Eval benchmark.