Real-Time Video Generation with Pyramid Attention Broadcast
Xuanlei Zhao, Xiaolong Jin, Kai Wang, Yang You
2024-08-23
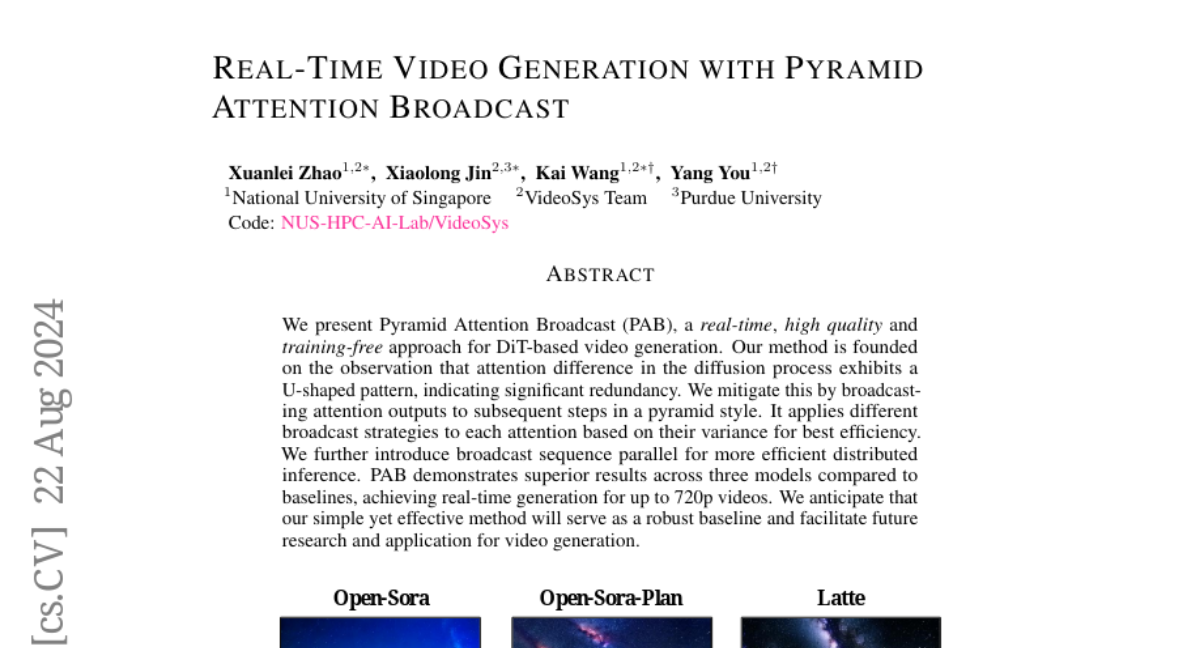
Summary
This paper presents Pyramid Attention Broadcast (PAB), a new method for generating high-quality videos in real-time without needing extensive training.
What's the problem?
Generating videos using existing methods can be slow and inefficient, especially when trying to maintain high quality. Many models also struggle with how to handle the attention given to different parts of the video, leading to wasted resources and time.
What's the solution?
The authors developed PAB, which improves video generation by using a pyramid-style approach to broadcast attention outputs across different steps in the process. This method reduces redundancy and enhances efficiency by applying different strategies based on the importance of each attention output. They also introduced a technique called broadcast sequence parallel to speed up the process even more. As a result, PAB can generate videos in real-time at resolutions up to 720p.
Why it matters?
This research is important because it allows for faster and more efficient video creation, making it accessible for various applications like streaming, gaming, and content creation. By improving the technology behind video generation, it can lead to better tools for filmmakers and creators.
Abstract
We present Pyramid Attention Broadcast (PAB), a real-time, high quality and training-free approach for DiT-based video generation. Our method is founded on the observation that attention difference in the diffusion process exhibits a U-shaped pattern, indicating significant redundancy. We mitigate this by broadcasting attention outputs to subsequent steps in a pyramid style. It applies different broadcast strategies to each attention based on their variance for best efficiency. We further introduce broadcast sequence parallel for more efficient distributed inference. PAB demonstrates superior results across three models compared to baselines, achieving real-time generation for up to 720p videos. We anticipate that our simple yet effective method will serve as a robust baseline and facilitate future research and application for video generation.