ReCapture: Generative Video Camera Controls for User-Provided Videos using Masked Video Fine-Tuning
David Junhao Zhang, Roni Paiss, Shiran Zada, Nikhil Karnad, David E. Jacobs, Yael Pritch, Inbar Mosseri, Mike Zheng Shou, Neal Wadhwa, Nataniel Ruiz
2024-11-08
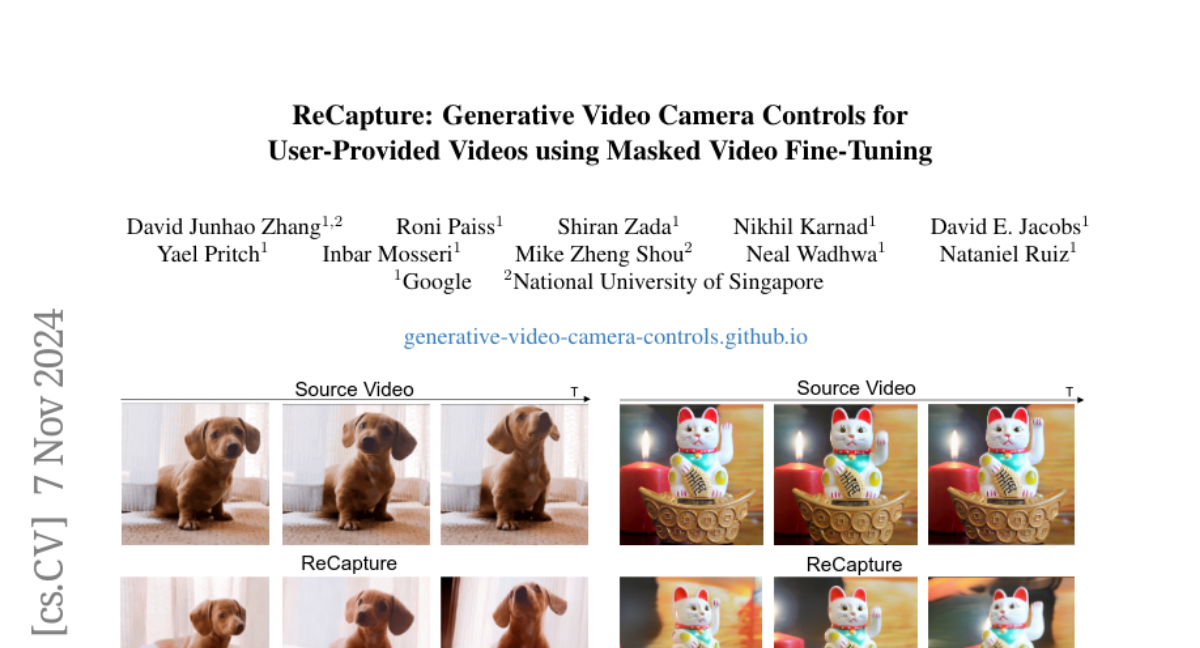
Summary
This paper introduces ReCapture, a method that allows users to create new videos from their own footage by changing the camera angles and movements, while also filling in missing parts of the scene.
What's the problem?
Existing video generation techniques can create new videos with controlled camera movements, but they can't easily apply these techniques to videos provided by users. This means that if someone has a video they want to modify, current methods can't help them change the perspective or add missing details effectively.
What's the solution?
ReCapture works in two main steps. First, it generates a rough version of the new video (called an anchor video) from the original user-provided video, but from a different camera angle. This anchor video may have some errors or missing parts. Then, it uses a technique called masked video fine-tuning to clean up this anchor video, correcting mistakes and filling in gaps to create a polished final video that looks good and maintains the original motion of the scene.
Why it matters?
This research is important because it provides a way for people to creatively modify their videos without needing advanced skills or tools. By allowing users to change how their videos look and feel, ReCapture can enhance storytelling in personal videos, filmmaking, and other visual media, making it more accessible for everyone.
Abstract
Recently, breakthroughs in video modeling have allowed for controllable camera trajectories in generated videos. However, these methods cannot be directly applied to user-provided videos that are not generated by a video model. In this paper, we present ReCapture, a method for generating new videos with novel camera trajectories from a single user-provided video. Our method allows us to re-generate the reference video, with all its existing scene motion, from vastly different angles and with cinematic camera motion. Notably, using our method we can also plausibly hallucinate parts of the scene that were not observable in the reference video. Our method works by (1) generating a noisy anchor video with a new camera trajectory using multiview diffusion models or depth-based point cloud rendering and then (2) regenerating the anchor video into a clean and temporally consistent reangled video using our proposed masked video fine-tuning technique.