ReCLAP: Improving Zero Shot Audio Classification by Describing Sounds
Sreyan Ghosh, Sonal Kumar, Chandra Kiran Reddy Evuru, Oriol Nieto, Ramani Duraiswami, Dinesh Manocha
2024-09-17
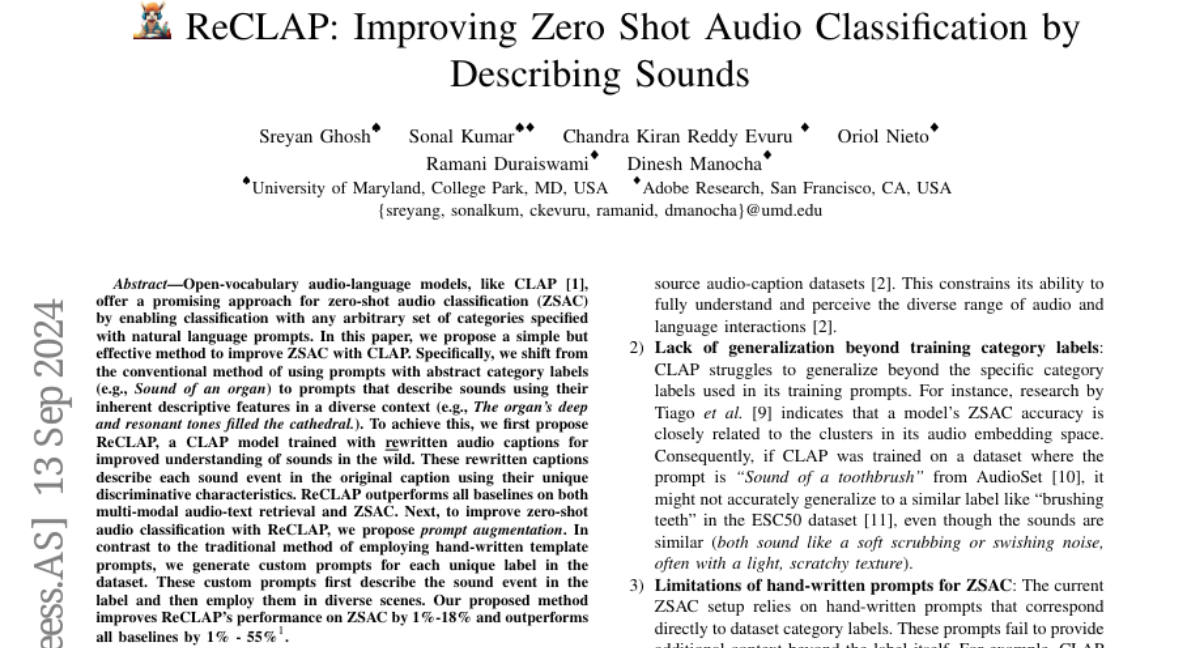
Summary
This paper introduces ReCLAP, a new model that improves the ability to classify sounds without needing specific examples by using descriptive natural language prompts.
What's the problem?
Zero-shot audio classification (ZSAC) is challenging because traditional methods often rely on vague category labels, like 'sound of an organ,' which do not provide enough detail for the model to understand the actual sound characteristics. This can lead to inaccurate classifications when the model encounters new sounds it hasn't been trained on.
What's the solution?
ReCLAP enhances ZSAC by using rewritten audio captions that describe sounds in detail, focusing on their unique features and contexts. Instead of just using basic labels, it generates custom prompts that explain the sounds more vividly, like describing the organ's 'deep and resonant tones.' This approach helps the model learn better and perform more accurately when classifying sounds it has never encountered before. ReCLAP has shown improvements in performance by 1% to 55% compared to previous methods.
Why it matters?
This research is important because it allows AI models to understand and classify sounds more effectively, even when they haven't been explicitly trained on those sounds. This capability can be useful in various applications, such as music recognition, environmental sound monitoring, and improving accessibility for hearing-impaired individuals by providing better sound descriptions.
Abstract
Open-vocabulary audio-language models, like CLAP, offer a promising approach for zero-shot audio classification (ZSAC) by enabling classification with any arbitrary set of categories specified with natural language prompts. In this paper, we propose a simple but effective method to improve ZSAC with CLAP. Specifically, we shift from the conventional method of using prompts with abstract category labels (e.g., Sound of an organ) to prompts that describe sounds using their inherent descriptive features in a diverse context (e.g.,The organ's deep and resonant tones filled the cathedral.). To achieve this, we first propose ReCLAP, a CLAP model trained with rewritten audio captions for improved understanding of sounds in the wild. These rewritten captions describe each sound event in the original caption using their unique discriminative characteristics. ReCLAP outperforms all baselines on both multi-modal audio-text retrieval and ZSAC. Next, to improve zero-shot audio classification with ReCLAP, we propose prompt augmentation. In contrast to the traditional method of employing hand-written template prompts, we generate custom prompts for each unique label in the dataset. These custom prompts first describe the sound event in the label and then employ them in diverse scenes. Our proposed method improves ReCLAP's performance on ZSAC by 1%-18% and outperforms all baselines by 1% - 55%.