Reconstructing Humans with a Biomechanically Accurate Skeleton
Yan Xia, Xiaowei Zhou, Etienne Vouga, Qixing Huang, Georgios Pavlakos
2025-03-31
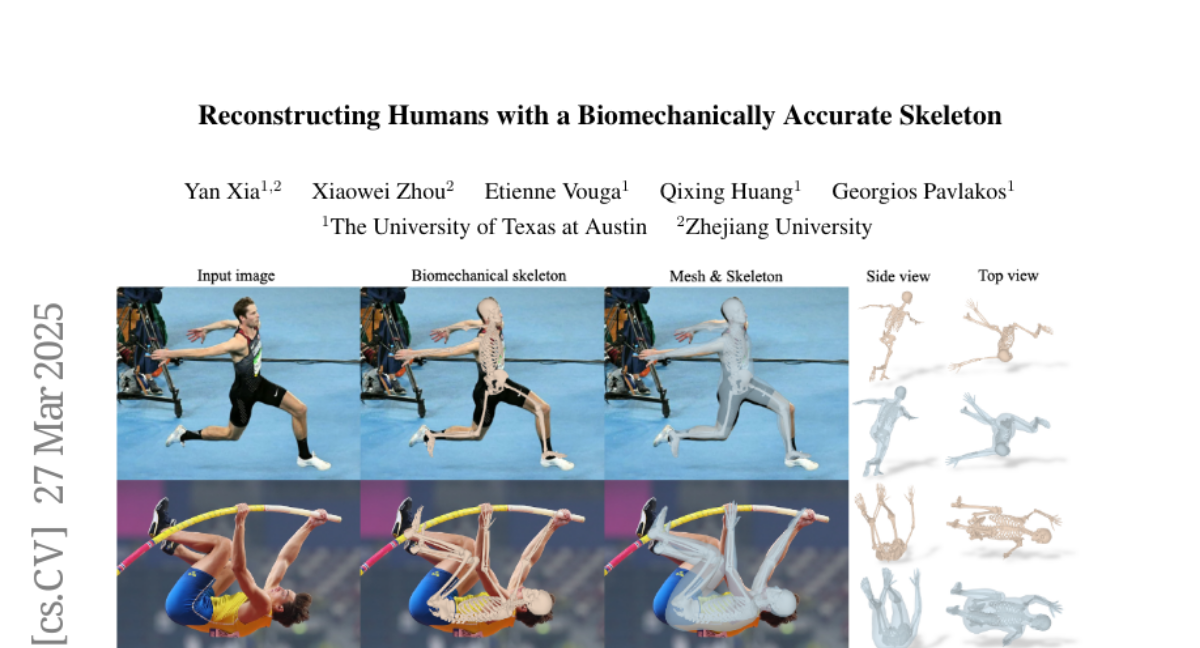
Summary
This paper is about creating 3D models of people from just one picture, making sure the models move like real humans.
What's the problem?
It's hard to make realistic 3D models of people from a single image, especially when they're in unusual poses, and existing models often make impossible movements.
What's the solution?
The researchers trained an AI model to estimate the pose of a person in a picture and create a 3D model that moves in a realistic way, avoiding unnatural joint angles.
Why it matters?
This work matters because it can be used to create more realistic avatars, improve motion capture in games and movies, and help with other applications that involve understanding human movement.
Abstract
In this paper, we introduce a method for reconstructing 3D humans from a single image using a biomechanically accurate skeleton model. To achieve this, we train a transformer that takes an image as input and estimates the parameters of the model. Due to the lack of training data for this task, we build a pipeline to produce pseudo ground truth model parameters for single images and implement a training procedure that iteratively refines these pseudo labels. Compared to state-of-the-art methods for 3D human mesh recovery, our model achieves competitive performance on standard benchmarks, while it significantly outperforms them in settings with extreme 3D poses and viewpoints. Additionally, we show that previous reconstruction methods frequently violate joint angle limits, leading to unnatural rotations. In contrast, our approach leverages the biomechanically plausible degrees of freedom making more realistic joint rotation estimates. We validate our approach across multiple human pose estimation benchmarks. We make the code, models and data available at: https://isshikihugh.github.io/HSMR/