Redefining Temporal Modeling in Video Diffusion: The Vectorized Timestep Approach
Yaofang Liu, Yumeng Ren, Xiaodong Cun, Aitor Artola, Yang Liu, Tieyong Zeng, Raymond H. Chan, Jean-michel Morel
2024-10-08
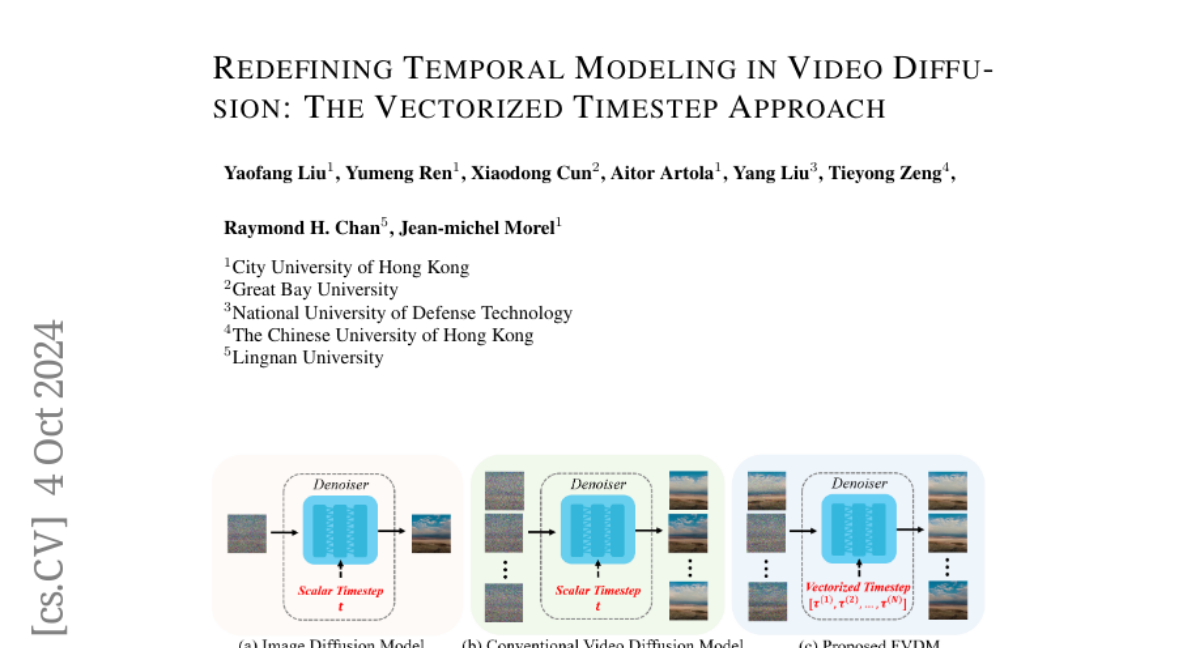
Summary
This paper introduces a new approach called the Vectorized Timestep to improve how video diffusion models generate videos. This method allows for better modeling of how video frames change over time.
What's the problem?
Current video diffusion models use a single value (scalar timestep) to represent time for an entire video clip, which limits their ability to accurately capture the complex changes that happen between individual frames. This makes it difficult to create realistic videos, especially for tasks like turning images into videos or generating long video sequences.
What's the solution?
The authors propose a frame-aware video diffusion model (FVDM) that uses a vectorized timestep variable (VTV). This means each frame can have its own unique way of changing over time, allowing the model to capture detailed relationships between frames. The new approach improves video quality and coherence by letting each frame evolve independently while still maintaining connections with neighboring frames. They tested this method across various tasks and found that it produced better results than existing models.
Why it matters?
This research is important because it enhances the ability of AI to generate high-quality videos, which can be used in many applications like film, gaming, and virtual reality. By addressing the limitations of previous methods, this new framework could lead to significant advancements in how we create and interact with video content.
Abstract
Diffusion models have revolutionized image generation, and their extension to video generation has shown promise. However, current video diffusion models~(VDMs) rely on a scalar timestep variable applied at the clip level, which limits their ability to model complex temporal dependencies needed for various tasks like image-to-video generation. To address this limitation, we propose a frame-aware video diffusion model~(FVDM), which introduces a novel vectorized timestep variable~(VTV). Unlike conventional VDMs, our approach allows each frame to follow an independent noise schedule, enhancing the model's capacity to capture fine-grained temporal dependencies. FVDM's flexibility is demonstrated across multiple tasks, including standard video generation, image-to-video generation, video interpolation, and long video synthesis. Through a diverse set of VTV configurations, we achieve superior quality in generated videos, overcoming challenges such as catastrophic forgetting during fine-tuning and limited generalizability in zero-shot methods.Our empirical evaluations show that FVDM outperforms state-of-the-art methods in video generation quality, while also excelling in extended tasks. By addressing fundamental shortcomings in existing VDMs, FVDM sets a new paradigm in video synthesis, offering a robust framework with significant implications for generative modeling and multimedia applications.