Reducing the Footprint of Multi-Vector Retrieval with Minimal Performance Impact via Token Pooling
Benjamin Clavié, Antoine Chaffin, Griffin Adams
2024-09-27
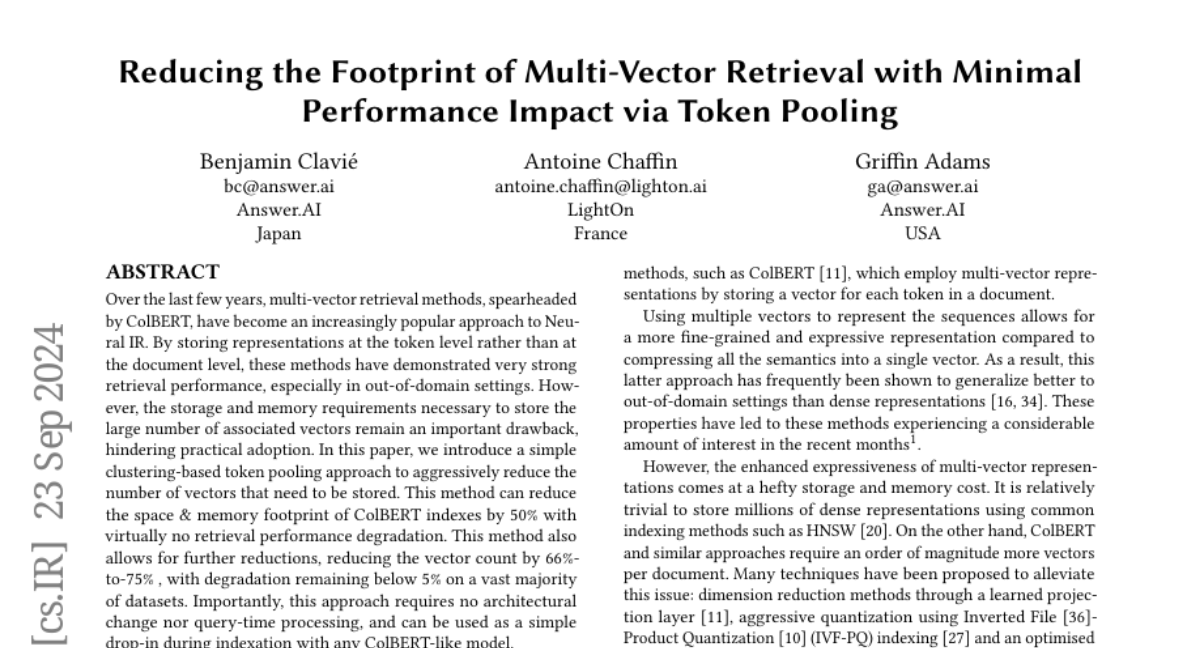
Summary
This paper talks about a new method called token pooling that helps improve multi-vector retrieval systems, like ColBERT, by reducing the number of stored vectors while keeping performance high. This makes it easier and more efficient to search through large amounts of data.
What's the problem?
Multi-vector retrieval methods, which break down documents into smaller parts (tokens) for better searching, can require a lot of storage and memory because they generate many vectors for each token. This can make it difficult to use these methods in practice, as they become resource-intensive and slow. The challenge is to find a way to reduce the number of vectors without sacrificing the quality of search results.
What's the solution?
The researchers propose a token pooling approach that clusters similar tokens together and averages their representations. By doing this, they can significantly reduce the number of vectors that need to be stored—by up to 75%—while maintaining a high level of accuracy in search results. This method does not require any changes to the existing models or complex processing during searches, making it easy to implement.
Why it matters?
This research is important because it enhances the efficiency of multi-vector retrieval systems, allowing them to handle larger datasets without needing excessive resources. By improving storage and memory usage while keeping performance high, this method can make advanced search technologies more accessible and practical for various applications, such as information retrieval and data analysis.
Abstract
Over the last few years, multi-vector retrieval methods, spearheaded by ColBERT, have become an increasingly popular approach to Neural IR. By storing representations at the token level rather than at the document level, these methods have demonstrated very strong retrieval performance, especially in out-of-domain settings. However, the storage and memory requirements necessary to store the large number of associated vectors remain an important drawback, hindering practical adoption. In this paper, we introduce a simple clustering-based token pooling approach to aggressively reduce the number of vectors that need to be stored. This method can reduce the space & memory footprint of ColBERT indexes by 50% with virtually no retrieval performance degradation. This method also allows for further reductions, reducing the vector count by 66%-to-75% , with degradation remaining below 5% on a vast majority of datasets. Importantly, this approach requires no architectural change nor query-time processing, and can be used as a simple drop-in during indexation with any ColBERT-like model.