ReferEverything: Towards Segmenting Everything We Can Speak of in Videos
Anurag Bagchi, Zhipeng Bao, Yu-Xiong Wang, Pavel Tokmakov, Martial Hebert
2024-10-31
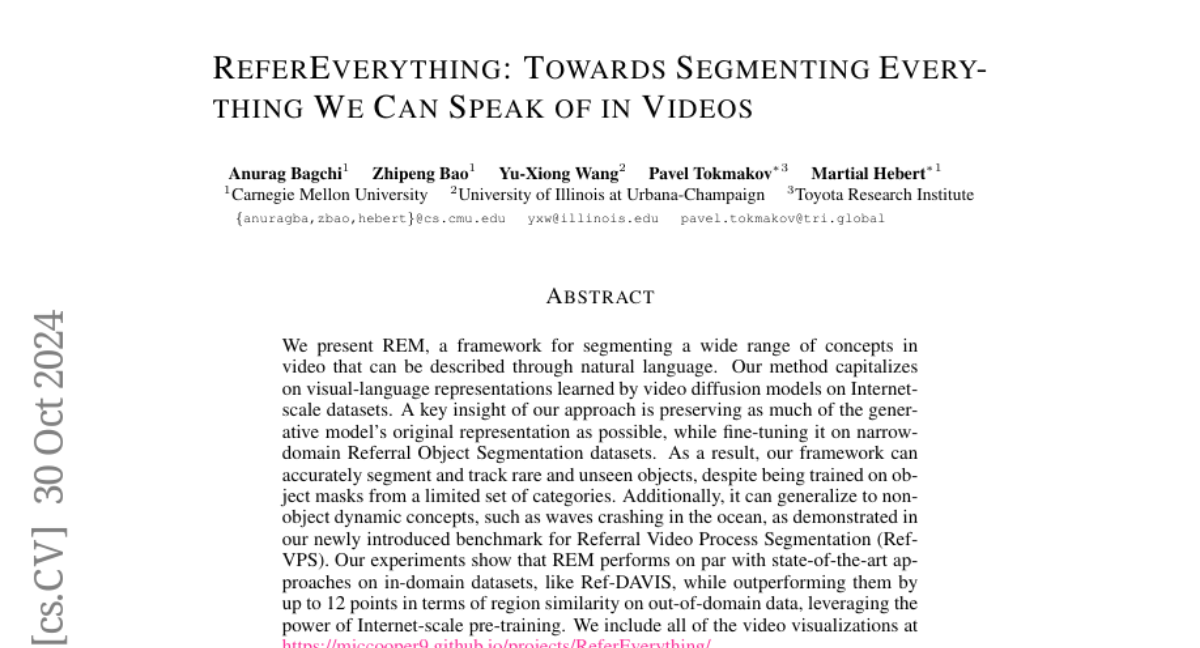
Summary
This paper introduces ReferEverything (REM), a framework designed to segment and track various concepts in videos based on natural language descriptions. It aims to improve how AI understands and identifies objects and actions in video content.
What's the problem?
Current methods for segmenting objects in videos often struggle to accurately identify and track rare or unseen items, especially when trained on limited categories. Additionally, many existing systems do not generalize well to dynamic concepts that aren't strictly defined as objects, making it difficult for AI to understand complex scenes in videos.
What's the solution?
REM tackles these challenges by using visual-language representations learned from large datasets. It fine-tunes the model on specific datasets for Referral Object Segmentation to enhance its accuracy. The framework can segment not only traditional objects but also dynamic concepts like waves or movements, which are harder to categorize. The authors tested REM against state-of-the-art methods and found it performed well on both familiar and unfamiliar tasks, showing significant improvements in tracking and identifying objects across various video scenarios.
Why it matters?
This research is important because it enhances the capabilities of AI systems in video analysis, making them more versatile and effective. By improving how AI segments and understands both objects and dynamic actions in videos, REM can be applied in areas such as video editing, surveillance, and content creation, leading to better user experiences and more accurate information retrieval.
Abstract
We present REM, a framework for segmenting a wide range of concepts in video that can be described through natural language. Our method capitalizes on visual-language representations learned by video diffusion models on Internet-scale datasets. A key insight of our approach is preserving as much of the generative model's original representation as possible, while fine-tuning it on narrow-domain Referral Object Segmentation datasets. As a result, our framework can accurately segment and track rare and unseen objects, despite being trained on object masks from a limited set of categories. Additionally, it can generalize to non-object dynamic concepts, such as waves crashing in the ocean, as demonstrated in our newly introduced benchmark for Referral Video Process Segmentation (Ref-VPS). Our experiments show that REM performs on par with state-of-the-art approaches on in-domain datasets, like Ref-DAVIS, while outperforming them by up to twelve points in terms of region similarity on out-of-domain data, leveraging the power of Internet-scale pre-training.