Relaxed Recursive Transformers: Effective Parameter Sharing with Layer-wise LoRA
Sangmin Bae, Adam Fisch, Hrayr Harutyunyan, Ziwei Ji, Seungyeon Kim, Tal Schuster
2024-10-29
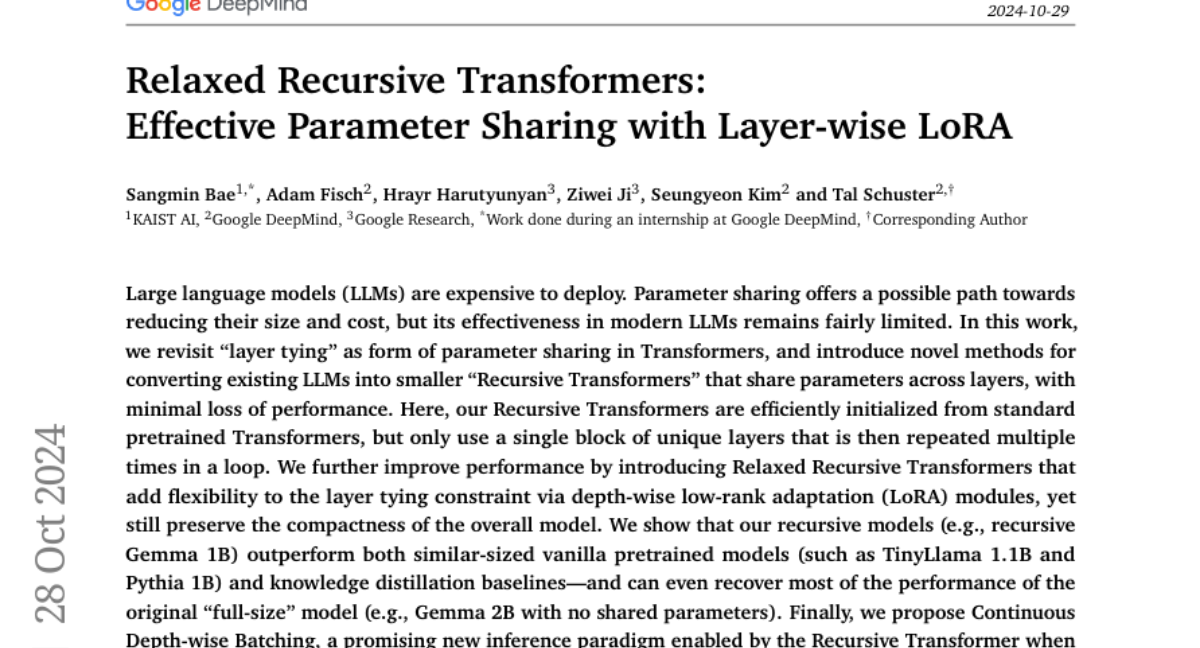
Summary
This paper discusses Relaxed Recursive Transformers, a new model design that reduces the size and cost of large language models (LLMs) while maintaining their performance by sharing parameters across layers.
What's the problem?
Large language models are powerful but very expensive to deploy due to their size and the resources needed to run them. Traditional methods for reducing their size, like parameter sharing, haven't been very effective with modern LLMs. This makes it challenging for organizations to use these models in practical applications without incurring high costs.
What's the solution?
The authors introduce Relaxed Recursive Transformers, which improve parameter sharing by using a single set of layers that can be reused multiple times in a loop. This method allows the model to maintain its performance while being smaller and more efficient. They also add a technique called Low-Rank Adaptation (LoRA), which lets each layer make small adjustments to the shared parameters, enhancing flexibility and performance without needing separate parameters for each layer. This approach allows for effective training and optimization without extensive resources.
Why it matters?
This research is significant because it offers a way to make advanced AI models more accessible by reducing their deployment costs. By improving how LLMs share parameters and adapt to different tasks, Relaxed Recursive Transformers could enable more organizations to utilize powerful AI technologies, leading to broader applications in areas like natural language processing, customer service, and content creation.
Abstract
Large language models (LLMs) are expensive to deploy. Parameter sharing offers a possible path towards reducing their size and cost, but its effectiveness in modern LLMs remains fairly limited. In this work, we revisit "layer tying" as form of parameter sharing in Transformers, and introduce novel methods for converting existing LLMs into smaller "Recursive Transformers" that share parameters across layers, with minimal loss of performance. Here, our Recursive Transformers are efficiently initialized from standard pretrained Transformers, but only use a single block of unique layers that is then repeated multiple times in a loop. We further improve performance by introducing Relaxed Recursive Transformers that add flexibility to the layer tying constraint via depth-wise low-rank adaptation (LoRA) modules, yet still preserve the compactness of the overall model. We show that our recursive models (e.g., recursive Gemma 1B) outperform both similar-sized vanilla pretrained models (such as TinyLlama 1.1B and Pythia 1B) and knowledge distillation baselines -- and can even recover most of the performance of the original "full-size" model (e.g., Gemma 2B with no shared parameters). Finally, we propose Continuous Depth-wise Batching, a promising new inference paradigm enabled by the Recursive Transformer when paired with early exiting. In a theoretical analysis, we show that this has the potential to lead to significant (2-3x) gains in inference throughput.