Repulsive Score Distillation for Diverse Sampling of Diffusion Models
Nicolas Zilberstein, Morteza Mardani, Santiago Segarra
2024-06-25
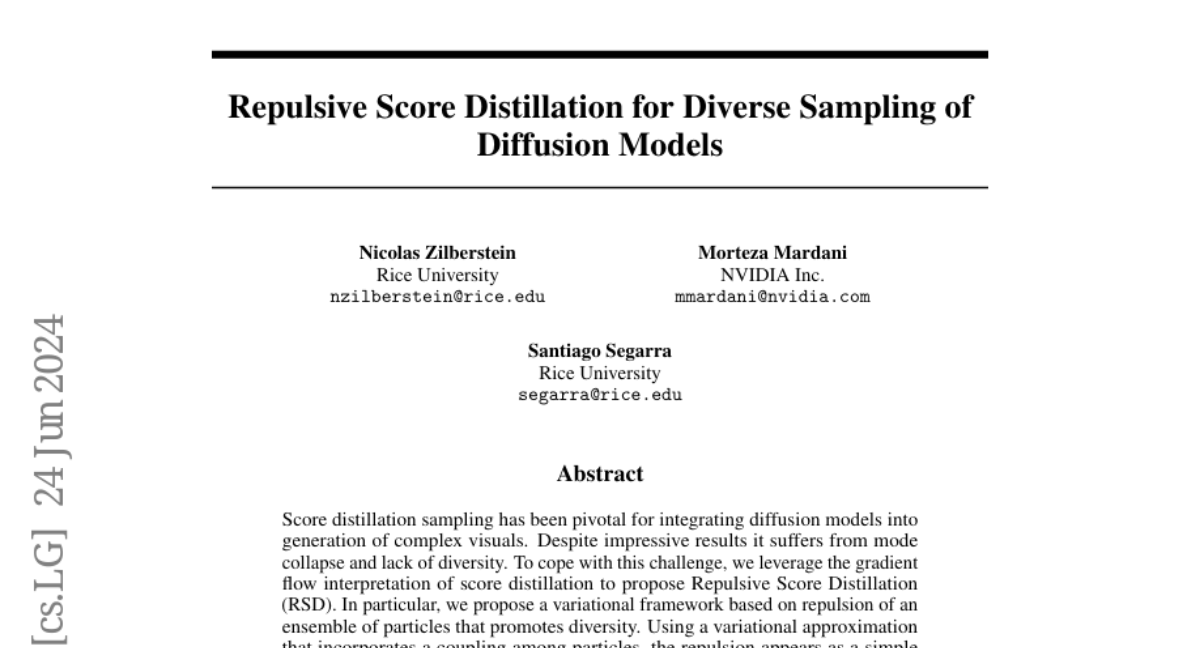
Summary
This paper introduces Repulsive Score Distillation (RSD), a new method that helps improve the generation of complex images using diffusion models by promoting diversity in the outputs.
What's the problem?
While diffusion models have shown great promise in creating detailed visuals, they often suffer from issues like mode collapse, where the model produces very similar outputs instead of a variety of different ones. This lack of diversity can limit the effectiveness and creativity of the generated images.
What's the solution?
To address these challenges, the authors propose RSD, which uses a technique based on the idea of repulsion among particles (or samples) during the generation process. By encouraging these particles to be different from each other, RSD helps create a wider range of outputs. The method incorporates a variational framework that allows particles to interact based on their similarities, which helps maintain a balance between computational efficiency, quality of images, and diversity in the results. They tested RSD on various tasks, including generating images from text descriptions and solving inverse problems, and found it performed better than existing methods.
Why it matters?
This research is important because it enhances how diffusion models generate images, making them more versatile and capable of producing diverse outputs. By improving the quality and variety of generated visuals, RSD can benefit applications in art, design, gaming, and any field that relies on creative visual content.
Abstract
Score distillation sampling has been pivotal for integrating diffusion models into generation of complex visuals. Despite impressive results it suffers from mode collapse and lack of diversity. To cope with this challenge, we leverage the gradient flow interpretation of score distillation to propose Repulsive Score Distillation (RSD). In particular, we propose a variational framework based on repulsion of an ensemble of particles that promotes diversity. Using a variational approximation that incorporates a coupling among particles, the repulsion appears as a simple regularization that allows interaction of particles based on their relative pairwise similarity, measured e.g., via radial basis kernels. We design RSD for both unconstrained and constrained sampling scenarios. For constrained sampling we focus on inverse problems in the latent space that leads to an augmented variational formulation, that strikes a good balance between compute, quality and diversity. Our extensive experiments for text-to-image generation, and inverse problems demonstrate that RSD achieves a superior trade-off between diversity and quality compared with state-of-the-art alternatives.